Open Access
ARTICLE
A Deep Real-Time Fire Prediction Parallel D-CNN Model on UDOO BOLT V8
Department of Computer Sciences, College of Computer and Information Sciences, Princess Nourah Bint Abdulrahman University, P.O. Box 84428, Riyadh, 11671, Saudi Arabia
* Corresponding Author: Hanan A. Hosni Mahmoud. Email:
Computers, Materials & Continua 2022, 73(3), 6237-6252. https://doi.org/10.32604/cmc.2022.030963
Received 07 April 2022; Accepted 29 May 2022; Issue published 28 July 2022
Abstract
Hazardous incidences have significant influences on human life, and fire is one of the foremost causes of such hazard in most nations. Fire prediction and classification model from a set of fire images can decrease the risk of losing human lives and assets. Timely promotion of fire emergency can be of great aid. Therefore, construction of these prediction models is relevant and critical. This article proposes an operative fire prediction model that depends on a prediction unit embedded in the processor UDOO BOLT V8 hardware to predict fires in real time. A fire image database is improved to enhance the images quality prior to classify them as either fire or non-fire. Our proposed deep learning–based Very Deep Convolutional Networks Visual Geometry Group (VGG-16) model (Parallel VGG-16) is an enhanced version of the VGG-16 model, by incorporating parallel convolution layers and a fusion module for better accuracy. The experimental results validate the performance of the Parallel VGG-16 which achieves an accuracy of 97%, compared to the compared state-of-the-art models. Moreover, we integrate the prediction module into a UDOO BOLT V8 computer, which precisely controlled the fire alarm so that it can cautious people from fire in real time. In this paper we propose a complete fire prediction model using a camera and the UDOO BOLT V8 embedded system. Our experiments validate the effectiveness and applicability of the proposed fire model.Keywords
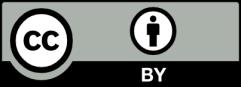