Open Access
ARTICLE
Differentially Private Support Vector Machines with Knowledge Aggregation
School of Cyberspace Security, Xi’an University of Posts and Telecommunications, Xi’an, 710121, China
* Corresponding Author: Shuanggen Liu. Email:
(This article belongs to the Special Issue: Security, Privacy, and Robustness for Trustworthy AI Systems)
Computers, Materials & Continua 2024, 78(3), 3891-3907. https://doi.org/10.32604/cmc.2024.048115
Received 28 November 2023; Accepted 23 January 2024; Issue published 26 March 2024
Abstract
With the widespread data collection and processing, privacy-preserving machine learning has become increasingly important in addressing privacy risks related to individuals. Support vector machine (SVM) is one of the most elementary learning models of machine learning. Privacy issues surrounding SVM classifier training have attracted increasing attention. In this paper, we investigate Differential Privacy-compliant Federated Machine Learning with Dimensionality Reduction, called FedDPDR-DPML, which greatly improves data utility while providing strong privacy guarantees. Considering in distributed learning scenarios, multiple participants usually hold unbalanced or small amounts of data. Therefore, FedDPDR-DPML enables multiple participants to collaboratively learn a global model based on weighted model averaging and knowledge aggregation and then the server distributes the global model to each participant to improve local data utility. Aiming at high-dimensional data, we adopt differential privacy in both the principal component analysis (PCA)-based dimensionality reduction phase and SVM classifiers training phase, which improves model accuracy while achieving strict differential privacy protection. Besides, we train Differential privacy (DP)-compliant SVM classifiers by adding noise to the objective function itself, thus leading to better data utility. Extensive experiments on three high-dimensional datasets demonstrate that FedDPDR-DPML can achieve high accuracy while ensuring strong privacy protection.Keywords
Cite This Article
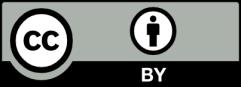