Open Access
ARTICLE
Ensemble 1D DenseNet Damage Identification Method Based on Vibration Acceleration
1 School of Civil Engineering, Chongqing Jiaotong University, Chongqing, 400074, China
2 School of Architecture and Art Design, Henan Polytechnic University, Jiaozuo, 454001, China
3 Zhong Yun International Engineering Co., Ltd., Zhengzhou, 450007, China
* Corresponding Author: Chun Sha. Email:
Structural Durability & Health Monitoring 2023, 17(5), 369-381. https://doi.org/10.32604/sdhm.2023.027948
Received 23 December 2022; Accepted 14 March 2023; Issue published 07 September 2023
Abstract
Convolution neural networks in deep learning can solve the problem of damage identification based on vibration acceleration. By combining multiple 1D DenseNet submodels, a new ensemble learning method is proposed to improve identification accuracy. 1D DenseNet is built using standard 1D CNN and DenseNet basic blocks, and the acceleration data obtained from multiple sampling points is brought into the 1D DenseNet training to generate submodels after offset sampling. When using submodels for damage identification, the voting method ideas in ensemble learning are used to vote on the results of each submodel, and then vote centrally. Finally, the cantilever damage problem simulated by ABAQUS is selected as a case study to discuss the excellent performance of the proposed method. The results show that the ensemble 1D DenseNet damage identification method outperforms any submodel in terms of accuracy. Furthermore, the submodel is visualized to demonstrate its operation mode.Keywords
Cite This Article
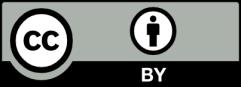