Open Access
ARTICLE
Single-point and Filtered Relative Position Estimation for Visual Docking
1 PhD Student, Aerospace Engineering, Texas A&M University, College Station, 77843.
E-mail: dtconway@tamu.edu.
2 Professor, Aerospace Engineering, Texas A&M University, College Station, 77843.
E-mail: mortari@tamu.edu.
Computer Modeling in Engineering & Sciences 2016, 111(2), 147-169. https://doi.org/10.3970/cmes.2016.111.147
Abstract
This paper presents a new method to estimate position from line-ofsight measurements to known targets when attitude is known. The algorithm has two stages. The first produces a closed-form unbiased estimate for position that does not account for the measurement error covariance. The second stage is iterative and produces an estimate of position that explicitly accounts for the measurement error covariance and the coupling between measurement error and sensor-to-target distance. The algorithm gives an accurate estimate of both position and the corresponding position error covariance and has a low computational cost. The computational complexity is O(n) for n point-targets and only a 3 × 3 linear system must be solved. The algorithm is demonstrated for single-point position estimation to verify the accuracy of the resulting position and covariance. Significant improvements over current methods are shown through statistical tests. The algorithm is then demonstrated in the context of sequential filtering for space vehicle docking.Cite This Article
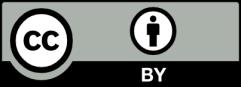