Open Access
ARTICLE
Using Hybrid Penalty and Gated Linear Units to Improve Wasserstein Generative Adversarial Networks for Single-Channel Speech Enhancement
1 School of Computer Science, Qinghai Normal University, Xining, 810008, China
2 The State Key Laboratory of Tibetan Intelligent Information Processing and Application, Xining, 810008, China
3 School of Electronic and Information Engineering, Lanzhou City University, Lanzhou, 730000, China
* Corresponding Author: Heming Huang. Email:
(This article belongs to the Special Issue: Bio-inspired Computer Modelling: Theories and Applications in Engineering and Sciences)
Computer Modeling in Engineering & Sciences 2023, 135(3), 2155-2172. https://doi.org/10.32604/cmes.2023.021453
Received 15 January 2022; Accepted 06 July 2022; Issue published 23 November 2022
Abstract
Recently, speech enhancement methods based on Generative Adversarial Networks have achieved good performance in time-domain noisy signals. However, the training of Generative Adversarial Networks has such problems as convergence difficulty, model collapse, etc. In this work, an end-to-end speech enhancement model based on Wasserstein Generative Adversarial Networks is proposed, and some improvements have been made in order to get faster convergence speed and better generated speech quality. Specifically, in the generator coding part, each convolution layer adopts different convolution kernel sizes to conduct convolution operations for obtaining speech coding information from multiple scales; a gated linear unit is introduced to alleviate the vanishing gradient problem with the increase of network depth; the gradient penalty of the discriminator is replaced with spectral normalization to accelerate the convergence rate of the model; a hybrid penalty term composed of L1 regularization and a scale-invariant signal-to-distortion ratio is introduced into the loss function of the generator to improve the quality of generated speech. The experimental results on both TIMIT corpus and Tibetan corpus show that the proposed model improves the speech quality significantly and accelerates the convergence speed of the model.Keywords
Cite This Article
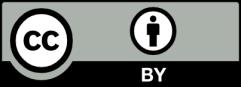