Open Access
ARTICLE
Prediction of Damping Capacity Demand in Seismic Base Isolators via Machine Learning
1 Department of Civil Engineering, Istanbul University–Cerrahpaşa, Istanbul, 34320, Turkey
2 Department of Informatics, Mimar Sinan Fine Arts University, Istanbul, 34427, Turkey
3 Department of Civil and Environmental Engineering, Temple University, Philadelphia, PA, 19122, USA
4 Department of Smart City, Gachon University, Seongnam, 13120, Korea
* Corresponding Authors: Gebrail Bekdaş. Email: ; Zong Woo Geem. Email:
(This article belongs to the Special Issue: Meta-heuristic Algorithms in Materials Science and Engineering)
Computer Modeling in Engineering & Sciences 2024, 138(3), 2899-2924. https://doi.org/10.32604/cmes.2023.030418
Received 04 April 2023; Accepted 26 July 2023; Issue published 15 December 2023
Abstract
Base isolators used in buildings provide both a good acceleration reduction and structural vibration control structures. The base isolators may lose their damping capacity over time due to environmental or dynamic effects. This deterioration of them requires the determination of the maintenance and repair needs and is important for the long-term isolator life. In this study, an artificial intelligence prediction model has been developed to determine the damage and maintenance-repair requirements of isolators as a result of environmental effects and dynamic factors over time. With the developed model, the required damping capacity of the isolator structure was estimated and compared with the previously placed isolator capacity, and the decrease in the damping property was tried to be determined. For this purpose, a data set was created by collecting the behavior of structures with single degrees of freedom (SDOF), different stiffness, damping ratio and natural period isolated from the foundation under far fault earthquakes. The data is divided into 5 different damping classes varying between 10% and 50%. Machine learning model was trained in damping classes with the data on the structure’s response to random seismic vibrations. As a result of the isolator behavior under randomly selected earthquakes, the recorded motion and structural acceleration of the structure against any seismic vibration were examined, and the decrease in the damping capacity was estimated on a class basis. The performance loss of the isolators, which are separated according to their damping properties, has been tried to be determined, and the reductions in the amounts to be taken into account have been determined by class. In the developed prediction model, using various supervised machine learning classification algorithms, the classification algorithm providing the highest precision for the model has been decided. When the results are examined, it has been determined that the damping of the isolator structure with the machine learning method is predicted successfully at a level exceeding 96%, and it is an effective method in deciding whether there is a decrease in the damping capacity.Keywords
Cite This Article
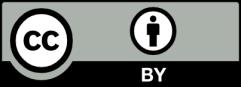