Open Access
ARTICLE
ThyroidNet: A Deep Learning Network for Localization and Classification of Thyroid Nodules
1 Ultrasonic Department, Zhongda Hospital Affiliated to Southeast University, Nanjing, 210009, China
2 School of Physics and Information Engineering, Jiangsu Second Normal University, Nanjing, 211200, China
3 School of Computing and Mathematical Sciences, University of Leicester, Leicester, LE1 7RH, UK
4 Department of Information Systems, Faculty of Computing and Information Technology, King Abdulaziz University, Jeddah, 21589, Saudi Arabia
5 State Key Laboratory of Millimeter Waves, Southeast University, Nanjing, 210096, China
6 Jiangsu Province Engineering Research Center of Basic Education Big Data Application, Jiangsu Second Normal University, Nanjing, 211200, China
7 School of Software Engineering, Quanzhou Normal University, Quanzhou, 362000, China
8 Department of Biological Sciences, Xi’an Jiaotong-Liverpool University, Suzhou, 215123, China
* Corresponding Authors: Xiaodong Gu. Email: ; Yudong Zhang. Email:
# These authors contributed equally to this work. Lu Chen and Huaqiang Chen are considered co-first authors
Computer Modeling in Engineering & Sciences 2024, 139(1), 361-382. https://doi.org/10.32604/cmes.2023.031229
Received 23 May 2023; Accepted 27 September 2023; Issue published 30 December 2023
Abstract
Aim: This study aims to establish an artificial intelligence model, ThyroidNet, to diagnose thyroid nodules using deep learning techniques accurately. Methods: A novel method, ThyroidNet, is introduced and evaluated based on deep learning for the localization and classification of thyroid nodules. First, we propose the multitask TransUnet, which combines the TransUnet encoder and decoder with multitask learning. Second, we propose the DualLoss function, tailored to the thyroid nodule localization and classification tasks. It balances the learning of the localization and classification tasks to help improve the model’s generalization ability. Third, we introduce strategies for augmenting the data. Finally, we submit a novel deep learning model, ThyroidNet, to accurately detect thyroid nodules. Results: ThyroidNet was evaluated on private datasets and was comparable to other existing methods, including U-Net and TransUnet. Experimental results show that ThyroidNet outperformed these methods in localizing and classifying thyroid nodules. It achieved improved accuracy of 3.9% and 1.5%, respectively. Conclusion: ThyroidNet significantly improves the clinical diagnosis of thyroid nodules and supports medical image analysis tasks. Future research directions include optimization of the model structure, expansion of the dataset size, reduction of computational complexity and memory requirements, and exploration of additional applications of ThyroidNet in medical image analysis.Keywords
Cite This Article
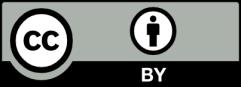