Open Access
ARTICLE
Generative Multi-Modal Mutual Enhancement Video Semantic Communications
1 The College of Communication and Information Engineering, Nanjing University of Posts and Telecommunications, Nanjing, 210023, China
2 The College of Science, Nanjing University of Posts and Telecommunications, Nanjing, 210023, China
* Corresponding Author: Wei Wu. Email:
(This article belongs to the Special Issue: Machine Learning Empowered Distributed Computing: Advance in Architecture, Theory and Practice)
Computer Modeling in Engineering & Sciences 2024, 139(3), 2985-3009. https://doi.org/10.32604/cmes.2023.046837
Received 16 October 2023; Accepted 19 December 2023; Issue published 11 March 2024
Abstract
Recently, there have been significant advancements in the study of semantic communication in single-modal scenarios. However, the ability to process information in multi-modal environments remains limited. Inspired by the research and applications of natural language processing across different modalities, our goal is to accurately extract frame-level semantic information from videos and ultimately transmit high-quality videos. Specifically, we propose a deep learning-based Multi-Modal Mutual Enhancement Video Semantic Communication system, called M3E-VSC. Built upon a Vector Quantized Generative Adversarial Network (VQGAN), our system aims to leverage mutual enhancement among different modalities by using text as the main carrier of transmission. With it, the semantic information can be extracted from key-frame images and audio of the video and perform differential value to ensure that the extracted text conveys accurate semantic information with fewer bits, thus improving the capacity of the system. Furthermore, a multi-frame semantic detection module is designed to facilitate semantic transitions during video generation. Simulation results demonstrate that our proposed model maintains high robustness in complex noise environments, particularly in low signal-to-noise ratio conditions, significantly improving the accuracy and speed of semantic transmission in video communication by approximately 50 percent.Keywords
Cite This Article
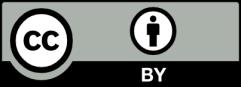