Open Access
ARTICLE
Image Super-Resolution Reconstruction Based on Dual Residual Network
1 Harbin Engineering University, Harbin, 150001, China
2 Xidian University, Xi'an, 710000, China
3 The 54th Research Institute of CETC, Shijiazhuang, 050000, China
4 The Chinese University of Hong Kong, Hong Kong, 999077, China
* Corresponding Author: Liguo Zhang. Email:
Journal of New Media 2022, 4(1), 27-39. https://doi.org/10.32604/jnm.2022.027826
Received 26 January 2022; Accepted 09 March 2022; Issue published 21 April 2022
Abstract
Research shows that deep learning algorithms can effectively improve a single image's super-resolution quality. However, if the algorithm is solely focused on increasing network depth and the desired result is not achieved, difficulties in the training process are more likely to arise. Simultaneously, the function space that can be transferred from a low-resolution image to a high-resolution image is enormous, making finding a satisfactory solution difficult. In this paper, we propose a deep learning method for single image super-resolution. The MDRN network framework uses multi-scale residual blocks and dual learning to fully acquire features in low-resolution images. Finally, these features will be sent to the image reconstruction module to restore high-quality images. The function space is constrained by the closed-loop formed by dual learning, which provides additional supervision for the super-resolution reconstruction of the image. The up-sampling process includes residual blocks with short-hop connections, so that the network focuses on learning high-frequency information, and strives to reconstruct images with richer feature details. The experimental results of ×4 and ×8 super-resolution reconstruction of the image show that the quality of the reconstructed image with this method is better than some existing experimental results of image super-resolution reconstruction in subjective visual effects and objective evaluation indicators.Keywords
Cite This Article
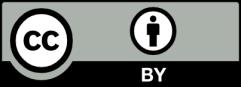
This work is licensed under a Creative Commons Attribution 4.0 International License , which permits unrestricted use, distribution, and reproduction in any medium, provided the original work is properly cited.