Open Access
ARTICLE
Facial Image-Based Autism Detection: A Comparative Study of Deep Neural Network Classifiers
1 Faculty of Computer Science and Information Technology, The Superior University, Lahore, 54600, Pakistan
2 Intelligent Data Visual Computing Research (IDVCR), Faculty of Computer Science and Information Technology, The Superior University, Lahore, 54600, Pakistan
3 Information Systems Department, College of Computer and Information Sciences, Imam Mohammad Ibn Saud Islamic University (IMSIU), Riyadh, 12571, Saudi Arabia
4 School of Computer Science and Electronic Engineering (CSEE), University of Essex, Wivenhoe Park, Colchester, CO4 3SQ, UK
* Corresponding Author: Sheeraz Akram. Email:
(This article belongs to the Special Issue: Deep Learning in Medical Imaging-Disease Segmentation and Classification)
Computers, Materials & Continua 2024, 78(1), 105-126. https://doi.org/10.32604/cmc.2023.045022
Received 15 August 2023; Accepted 07 November 2023; Issue published 30 January 2024
Abstract
Autism Spectrum Disorder (ASD) is a neurodevelopmental condition characterized by significant challenges in social interaction, communication, and repetitive behaviors. Timely and precise ASD detection is crucial, particularly in regions with limited diagnostic resources like Pakistan. This study aims to conduct an extensive comparative analysis of various machine learning classifiers for ASD detection using facial images to identify an accurate and cost-effective solution tailored to the local context. The research involves experimentation with VGG16 and MobileNet models, exploring different batch sizes, optimizers, and learning rate schedulers. In addition, the “Orange” machine learning tool is employed to evaluate classifier performance and automated image processing capabilities are utilized within the tool. The findings unequivocally establish VGG16 as the most effective classifier with a 5-fold cross-validation approach. Specifically, VGG16, with a batch size of 2 and the Adam optimizer, trained for 100 epochs, achieves a remarkable validation accuracy of 99% and a testing accuracy of 87%. Furthermore, the model achieves an F1 score of 88%, precision of 85%, and recall of 90% on test images. To validate the practical applicability of the VGG16 model with 5-fold cross-validation, the study conducts further testing on a dataset sourced from autism centers in Pakistan, resulting in an accuracy rate of 85%. This reaffirms the model’s suitability for real-world ASD detection. This research offers valuable insights into classifier performance, emphasizing the potential of machine learning to deliver precise and accessible ASD diagnoses via facial image analysis.Keywords
Cite This Article
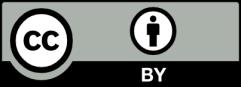