Open Access
ARTICLE
Traffic-Aware Fuzzy Classification Model to Perform IoT Data Traffic Sourcing with the Edge Computing
College of Information Engineering, Zhengzhou University of Technology, Zhengzhou, 450044, China
* Corresponding Author: Huixiang Xu. Email:
Computers, Materials & Continua 2024, 78(2), 2309-2335. https://doi.org/10.32604/cmc.2024.046253
Received 25 September 2023; Accepted 19 December 2023; Issue published 27 February 2024
Abstract
The Internet of Things (IoT) has revolutionized how we interact with and gather data from our surrounding environment. IoT devices with various sensors and actuators generate vast amounts of data that can be harnessed to derive valuable insights. The rapid proliferation of Internet of Things (IoT) devices has ushered in an era of unprecedented data generation and connectivity. These IoT devices, equipped with many sensors and actuators, continuously produce vast volumes of data. However, the conventional approach of transmitting all this data to centralized cloud infrastructures for processing and analysis poses significant challenges. However, transmitting all this data to a centralized cloud infrastructure for processing and analysis can be inefficient and impractical due to bandwidth limitations, network latency, and scalability issues. This paper proposed a Self-Learning Internet Traffic Fuzzy Classifier (SLItFC) for traffic data analysis. The proposed techniques effectively utilize clustering and classification procedures to improve classification accuracy in analyzing network traffic data. SLItFC addresses the intricate task of efficiently managing and analyzing IoT data traffic at the edge. It employs a sophisticated combination of fuzzy clustering and self-learning techniques, allowing it to adapt and improve its classification accuracy over time. This adaptability is a crucial feature, given the dynamic nature of IoT environments where data patterns and traffic characteristics can evolve rapidly. With the implementation of the fuzzy classifier, the accuracy of the clustering process is improvised with the reduction of the computational time. SLItFC can reduce computational time while maintaining high classification accuracy. This efficiency is paramount in edge computing, where resource constraints demand streamlined data processing. Additionally, SLItFC’s performance advantages make it a compelling choice for organizations seeking to harness the potential of IoT data for real-time insights and decision-making. With the Self-Learning process, the SLItFC model monitors the network traffic data acquired from the IoT Devices. The Sugeno fuzzy model is implemented within the edge computing environment for improved classification accuracy. Simulation analysis stated that the proposed SLItFC achieves 94.5% classification accuracy with reduced classification time.Keywords
Cite This Article
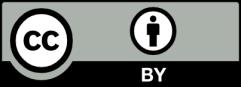