Open Access
ARTICLE
Contrastive Consistency and Attentive Complementarity for Deep Multi-View Subspace Clustering
School of Information Engineering, Southwest University of Science and Technology, Mianyang, 621010, China
* Corresponding Author: Bin Wu. Email:
(This article belongs to the Special Issue: Development and Industrial Application of AI Technologies)
Computers, Materials & Continua 2024, 79(1), 143-160. https://doi.org/10.32604/cmc.2023.046011
Received 15 September 2023; Accepted 28 November 2023; Issue published 25 April 2024
Abstract
Deep multi-view subspace clustering (DMVSC) based on self-expression has attracted increasing attention due to its outstanding performance and nonlinear application. However, most existing methods neglect that view-private meaningless information or noise may interfere with the learning of self-expression, which may lead to the degeneration of clustering performance. In this paper, we propose a novel framework of Contrastive Consistency and Attentive Complementarity (CCAC) for DMVsSC. CCAC aligns all the self-expressions of multiple views and fuses them based on their discrimination, so that it can effectively explore consistent and complementary information for achieving precise clustering. Specifically, the view-specific self-expression is learned by a self-expression layer embedded into the auto-encoder network for each view. To guarantee consistency across views and reduce the effect of view-private information or noise, we align all the view-specific self-expressions by contrastive learning. The aligned self-expressions are assigned adaptive weights by channel attention mechanism according to their discrimination. Then they are fused by convolution kernel to obtain consensus self-expression with maximum complementarity of multiple views. Extensive experimental results on four benchmark datasets and one large-scale dataset of the CCAC method outperform other state-of-the-art methods, demonstrating its clustering effectiveness.Keywords
Cite This Article
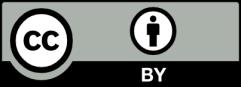