Open Access
ARTICLE
Contrastive Clustering for Unsupervised Recognition of Interference Signals
1 The School of Communication Engineering, Hangzhou Dianzi University, Hangzhou, 310018, China
2 The Science and Technology on Communication Information Security Control Laboratory, Jiaxing, 314001, China
* Corresponding Author: Zhijin Zhao. Email:
Computer Systems Science and Engineering 2023, 46(2), 1385-1400. https://doi.org/10.32604/csse.2023.034543
Received 20 July 2022; Accepted 21 October 2022; Issue published 09 February 2023
Abstract
Interference signals recognition plays an important role in anti-jamming communication. With the development of deep learning, many supervised interference signals recognition algorithms based on deep learning have emerged recently and show better performance than traditional recognition algorithms. However, there is no unsupervised interference signals recognition algorithm at present. In this paper, an unsupervised interference signals recognition method called double phases and double dimensions contrastive clustering (DDCC) is proposed. Specifically, in the first phase, four data augmentation strategies for interference signals are used in data-augmentation-based (DA-based) contrastive learning. In the second phase, the original dataset’s k-nearest neighbor set (KNNset) is designed in double dimensions contrastive learning. In addition, a dynamic entropy parameter strategy is proposed. The simulation experiments of 9 types of interference signals show that random cropping is the best one of the four data augmentation strategies; the feature dimensional contrastive learning in the second phase can improve the clustering purity; the dynamic entropy parameter strategy can improve the stability of DDCC effectively. The unsupervised interference signals recognition results of DDCC and five other deep clustering algorithms show that the clustering performance of DDCC is superior to other algorithms. In particular, the clustering purity of our method is above 92%, SCAN’s is 81%, and the other three methods’ are below 71% when jamming-noise-ratio (JNR) is −5 dB. In addition, our method is close to the supervised learning algorithm.Keywords
Cite This Article
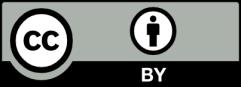