Open Access
ARTICLE
Enhancing Skin Cancer Diagnosis with Deep Learning: A Hybrid CNN-RNN Approach
1 Faculty of Information Technology, Beijing University of Technology, Beijing, 100124, China
2 Computer Science International Engineering Collage, Maynooth University, Kildare, W23 F2H6, Irland
3 Research Center for Data Hub and Security, Zhejiang Lab, Hangzhou, 311121, China
4 Computer Science Department, MNS University of Agriculture, Multan, 59220, Pakistan
* Corresponding Authors: Syeda Shamaila Zareen. Email: ; Guangmin Sun. Email:
(This article belongs to the Special Issue: Advanced Artificial Intelligence and Machine Learning Frameworks for Signal and Image Processing Applications)
Computers, Materials & Continua 2024, 79(1), 1497-1519. https://doi.org/10.32604/cmc.2024.047418
Received 05 November 2023; Accepted 31 January 2024; Issue published 25 April 2024
Abstract
Skin cancer diagnosis is difficult due to lesion presentation variability. Conventional methods struggle to manually extract features and capture lesions spatial and temporal variations. This study introduces a deep learning-based Convolutional and Recurrent Neural Network (CNN-RNN) model with a ResNet-50 architecture which used as the feature extractor to enhance skin cancer classification. Leveraging synergistic spatial feature extraction and temporal sequence learning, the model demonstrates robust performance on a dataset of 9000 skin lesion photos from nine cancer types. Using pre-trained ResNet-50 for spatial data extraction and Long Short-Term Memory (LSTM) for temporal dependencies, the model achieves a high average recognition accuracy, surpassing previous methods. The comprehensive evaluation, including accuracy, precision, recall, and F1-score, underscores the model’s competence in categorizing skin cancer types. This research contributes a sophisticated model and valuable guidance for deep learning-based diagnostics, also this model excels in overcoming spatial and temporal complexities, offering a sophisticated solution for dermatological diagnostics research.Keywords
Cite This Article
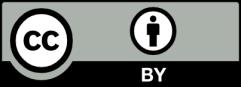