Open Access
ARTICLE
Sepsis Prediction Using CNNBDLSTM and Temporal Derivatives Feature Extraction in the IoT Medical Environment
1 Department of Information Systems, College of Computer and Information Sciences, Princess Nourah Bint Abdulrahman University, Riyadh, 11671, Kingdom of Saudi Arabia
2 Department of Computer Science and Information Technology, Applied College, Princess Nourah Bint Abdulrahman University, Riyadh, 11671, Kingdom of Saudi Arabia
* Corresponding Author: Nurul Halimatul Asmak Ismail. Email:
Computers, Materials & Continua 2024, 79(1), 1157-1185. https://doi.org/10.32604/cmc.2024.048051
Received 26 November 2023; Accepted 20 February 2024; Issue published 25 April 2024
Abstract
Background: Sepsis, a potentially fatal inflammatory disease triggered by infection, carries significant health implications worldwide. Timely detection is crucial as sepsis can rapidly escalate if left undetected. Recent advancements in deep learning (DL) offer powerful tools to address this challenge. Aim: Thus, this study proposed a hybrid CNNBDLSTM, a combination of a convolutional neural network (CNN) with a bi-directional long short-term memory (BDLSTM) model to predict sepsis onset. Implementing the proposed model provides a robust framework that capitalizes on the complementary strengths of both architectures, resulting in more accurate and timelier predictions. Method: The sepsis prediction method proposed here utilizes temporal feature extraction to delineate six distinct time frames before the onset of sepsis. These time frames adhere to the sepsis-3 standard requirement, which incorporates 12-h observation windows preceding sepsis onset. All models were trained using the Medical Information Mart for Intensive Care III (MIMIC-III) dataset, which sourced 61,522 patients with 40 clinical variables obtained from the IoT medical environment. The confusion matrix, the area under the receiver operating characteristic curve (AUCROC) curve, the accuracy, the precision, the F1-score, and the recall were deployed to evaluate the models. Result: The CNNBDLSTM model demonstrated superior performance compared to the benchmark and other models, achieving an AUCROC of 99.74% and an accuracy of 99.15% one hour before sepsis onset. These results indicate that the CNNBDLSTM model is highly effective in predicting sepsis onset, particularly within a close proximity of one hour. Implication: The results could assist practitioners in increasing the potential survival of the patient one hour before sepsis onset.Keywords
Cite This Article
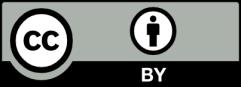