Open Access
ARTICLE
E2E-MFERC: A Multi-Face Expression Recognition Model for Group Emotion Assessment
1 Jiangsu Key Laboratory of Big Data Security & Intelligent Processing, Nanjing University of Posts and Telecommunications, Nanjing, 210042, China
2 School of Network Security, Jinling Institute of Technology, Nanjing, 211169, China
3 State Grid Jiangsu Electric Power Company Limited, Nanjing, 210000, China
4 School of Computer Science, Nanjing University of Posts and Telecommunications, Nanjing, 210042, China
* Corresponding Author: Xiaolong Xu. Email:
(This article belongs to the Special Issue: Deep Learning based Object Detection and Tracking in Videos)
Computers, Materials & Continua 2024, 79(1), 1105-1135. https://doi.org/10.32604/cmc.2024.048688
Received 15 December 2023; Accepted 01 March 2024; Issue published 25 April 2024
Abstract
In smart classrooms, conducting multi-face expression recognition based on existing hardware devices to assess students’ group emotions can provide educators with more comprehensive and intuitive classroom effect analysis, thereby continuously promoting the improvement of teaching quality. However, most existing multi-face expression recognition methods adopt a multi-stage approach, with an overall complex process, poor real-time performance, and insufficient generalization ability. In addition, the existing facial expression datasets are mostly single face images, which are of low quality and lack specificity, also restricting the development of this research. This paper aims to propose an end-to-end high-performance multi-face expression recognition algorithm model suitable for smart classrooms, construct a high-quality multi-face expression dataset to support algorithm research, and apply the model to group emotion assessment to expand its application value. To this end, we propose an end-to-end multi-face expression recognition algorithm model for smart classrooms (E2E-MFERC). In order to provide high-quality and highly targeted data support for model research, we constructed a multi-face expression dataset in real classrooms (MFED), containing 2,385 images and a total of 18,712 expression labels, collected from smart classrooms. In constructing E2E-MFERC, by introducing Re-parameterization visual geometry group (RepVGG) block and symmetric positive definite convolution (SPD-Conv) modules to enhance representational capability; combined with the cross stage partial network fusion module optimized by attention mechanism (C2f_Attention), it strengthens the ability to extract key information; adopts asymptotic feature pyramid network (AFPN) feature fusion tailored to classroom scenes and optimizes the head prediction output size; achieves high-performance end-to-end multi-face expression detection. Finally, we apply the model to smart classroom group emotion assessment and provide design references for classroom effect analysis evaluation metrics. Experiments based on MFED show that the mAP and F1-score of E2E-MFERC on classroom evaluation data reach 83.6% and 0.77, respectively, improving the mAP of same-scale You Only Look Once version 5 (YOLOv5) and You Only Look Once version 8 (YOLOv8) by 6.8% and 2.5%, respectively, and the F1-score by 0.06 and 0.04, respectively. E2E-MFERC model has obvious advantages in both detection speed and accuracy, which can meet the practical needs of real-time multi-face expression analysis in classrooms, and serve the application of teaching effect assessment very well.Keywords
Cite This Article
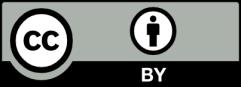