Open Access
ARTICLE
Automatic Finding of Brain-Tumour Group Using CNN Segmentation and Moth-Flame-Algorithm, Selected Deep and Handcrafted Features
1 Faculty of Electronic Engineering Technology, University Malaysia Perils (UniMAP), Pauh Putra Campus, Arau, Perlis, 02600, Malaysia
2 Department of Electrical and Communication Engineering, National University of Science and Technology, AL Hail, AL Seeb, 130, Sultanate of Oman
3 Department of Research and Innovation, Saveetha School of Engineering, Saveetha Institute of Medical and Technical Sciences (SIMATS), Chennai, TamiNadu, 602105, India
* Corresponding Authors: Imad Saud Al Naimi. Email: ; Muhammad lmran Ahmad. Email:
(This article belongs to the Special Issue: Deep Learning in Medical Imaging-Disease Segmentation and Classification)
Computers, Materials & Continua 2024, 79(2), 2585-2608. https://doi.org/10.32604/cmc.2024.046461
Received 02 December 2023; Accepted 08 March 2024; Issue published 15 May 2024
Abstract
Augmentation of abnormal cells in the brain causes brain tumor (BT), and early screening and treatment will reduce its harshness in patients. BT’s clinical level screening is usually performed with Magnetic Resonance Imaging (MRI) due to its multi-modality nature. The overall aims of the study is to introduce, test and verify an advanced image processing technique with algorithms to automatically extract tumour sections from brain MRI scans, facilitating improved accuracy. The research intends to devise a reliable framework for detecting the BT region in the two-dimensional (2D) MRI slice, and identifying its class with improved accuracy. The methodology for the devised framework comprises the phases of: (i) Collection and resizing of images, (ii) Implementation and Segmentation of Convolutional Neural Network (CNN), (iii) Deep feature extraction, (iv) Handcrafted feature extraction, (v) Moth-Flame-Algorithm (MFA) supported feature reduction, and (vi) Performance evaluation. This study utilized clinical-grade brain MRI of BRATS and TCIA datasets for the investigation. This framework segments detected the glioma (low/high grade) and glioblastoma class BT. This work helped to get a segmentation accuracy of over 98% with VGG-UNet and a classification accuracy of over 98% with the VGG16 scheme. This study has confirmed that the implemented framework is very efficient in detecting the BT in MRI slices with/without the skull section.Keywords
Cite This Article
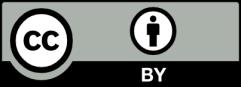