Open Access
ARTICLE
Real-Time Anomaly Detection in Packaged Food X-Ray Images Using Supervised Learning
1 Department of Computer Science, Kyonggi University, Suwon-si, 16227, Korea
2 Department of IT Contents, Hanshin University, Osan-si, 18101, Korea
3 Department of Computer Software Engineering, Soonchunhyang University, Asan-si, 31538, Korea
4 R&D Center, XAVIS Co. Ltd, Seongnam-si, 13202, Korea
* Corresponding Author: Min Hong. Email:
Computers, Materials & Continua 2021, 67(2), 2547-2568. https://doi.org/10.32604/cmc.2021.014642
Received 05 October 2020; Accepted 22 December 2020; Issue published 05 February 2021
Abstract
Physical contamination of food occurs when it comes into contact with foreign objects. Foreign objects can be introduced to food at any time during food delivery and packaging and can cause serious concerns such as broken teeth or choking. Therefore, a preventive method that can detect and remove foreign objects in advance is required. Several studies have attempted to detect defective products using deep learning networks. Because it is difficult to obtain foreign object-containing food data from industry, most studies on industrial anomaly detection have used unsupervised learning methods. This paper proposes a new method for real-time anomaly detection in packaged food products using a supervised learning network. In this study, a realistic X-ray image training dataset was constructed by augmenting foreign objects with normal product images in a cut-paste manner. Based on the augmented training dataset, we trained YOLOv4, a real-time object detection network, and detected foreign objects in the test data. We evaluated this method on images of pasta, snacks, pistachios, and red beans under the same conditions. The results show that the normal and defective products were classified with an accuracy of at least 94% for all packaged foods. For detecting foreign objects that are typically difficult to detect using the unsupervised learning and traditional methods, the proposed method achieved high-performance real-time anomaly detection. In addition, to eliminate the loss in high-resolution X-ray images, the false positive rate and accuracy could be lowered to 5% with patch-based training and a new post-processing algorithm.Keywords
Cite This Article
Citations
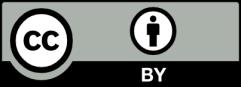
This work is licensed under a Creative Commons Attribution 4.0 International License , which permits unrestricted use, distribution, and reproduction in any medium, provided the original work is properly cited.