Open Access
ARTICLE
An Optimal Big Data Analytics with Concept Drift Detection on High-Dimensional Streaming Data
1 Department of Mathematics, Faculty of Science, New Valley University, El-Kharga, 72511, Egypt
2 Department of Information Systems, College of Computer and Information Sciences, Princess Nourah Bint Abdulrahman University, Riyadh, 84428, Saudi Arabia
3 Department of Computer Sciences, College of Computer and Information Sciences, Princess Nourah Bint Abdulrahman University, Riyadh, 84428, Saudi Arabia
4 Department of Entrepreneurship and Logistics, Plekhanov Russian University of Economics, Moscow, 117997, Russia
5 Department of Logistics, State University of Management, Moscow, 109542, Russia
* Corresponding Author: Romany F. Mansour. Email:
Computers, Materials & Continua 2021, 68(3), 2843-2858. https://doi.org/10.32604/cmc.2021.016626
Received 07 January 2021; Accepted 01 March 2021; Issue published 06 May 2021
Abstract
Big data streams started becoming ubiquitous in recent years, thanks to rapid generation of massive volumes of data by different applications. It is challenging to apply existing data mining tools and techniques directly in these big data streams. At the same time, streaming data from several applications results in two major problems such as class imbalance and concept drift. The current research paper presents a new Multi-Objective Metaheuristic Optimization-based Big Data Analytics with Concept Drift Detection (MOMBD-CDD) method on High-Dimensional Streaming Data. The presented MOMBD-CDD model has different operational stages such as pre-processing, CDD, and classification. MOMBD-CDD model overcomes class imbalance problem by Synthetic Minority Over-sampling Technique (SMOTE). In order to determine the oversampling rates and neighboring point values of SMOTE, Glowworm Swarm Optimization (GSO) algorithm is employed. Besides, Statistical Test of Equal Proportions (STEPD), a CDD technique is also utilized. Finally, Bidirectional Long Short-Term Memory (Bi-LSTM) model is applied for classification. In order to improve classification performance and to compute the optimum parameters for Bi-LSTM model, GSO-based hyperparameter tuning process is carried out. The performance of the presented model was evaluated using high dimensional benchmark streaming datasets namely intrusion detection (NSL KDDCup) dataset and ECUE spam dataset. An extensive experimental validation process confirmed the effective outcome of MOMBD-CDD model. The proposed model attained high accuracy of 97.45% and 94.23% on the applied KDDCup99 Dataset and ECUE Spam datasets respectively.Keywords
Cite This Article
Citations
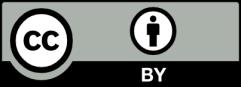