Open Access
ARTICLE
Q-Learning Based Routing Protocol for Congestion Avoidance
1 Department of Computer Science and Engineering, Chungnam National University, Korea
2 College of Technological Innovation, Zayed University, Abu Dhabi, UAE
3 Institute of Management Sciences, Peshawar, Pakistan
4 Department of Electrical Engineering, University of Engineering and Technology, Peshawar, Pakistan
5 Department of Computer and Telecommunications Engineering, Yonsei University, Korea
* Corresponding Author: Ki-Il Kim. Email:
(This article belongs to the Special Issue: Intelligent Software-defined Networking (SDN) Technologies for Future Generation Networks)
Computers, Materials & Continua 2021, 68(3), 3671-3692. https://doi.org/10.32604/cmc.2021.017475
Received 31 January 2021; Accepted 22 March 2021; Issue published 06 May 2021
Abstract
The end-to-end delay in a wired network is strongly dependent on congestion on intermediate nodes. Among lots of feasible approaches to avoid congestion efficiently, congestion-aware routing protocols tend to search for an uncongested path toward the destination through rule-based approaches in reactive/incident-driven and distributed methods. However, these previous approaches have a problem accommodating the changing network environments in autonomous and self-adaptive operations dynamically. To overcome this drawback, we present a new congestion-aware routing protocol based on a Q-learning algorithm in software-defined networks where logically centralized network operation enables intelligent control and management of network resources. In a proposed routing protocol, either one of uncongested neighboring nodes are randomly selected as next hop to distribute traffic load to multiple paths or Q-learning algorithm is applied to decide the next hop by modeling the state, Q-value, and reward function to set the desired path toward the destination. A new reward function that consists of a buffer occupancy, link reliability and hop count is considered. Moreover, look ahead algorithm is employed to update the Q-value with values within two hops simultaneously. This approach leads to a decision of the optimal next hop by taking congestion status in two hops into account, accordingly. Finally, the simulation results presented approximately 20% higher packet delivery ratio and 15% shorter end-to-end delay, compared to those with the existing scheme by avoiding congestion adaptively.Keywords
Cite This Article
Citations
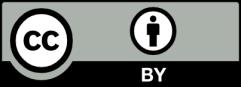