Open Access
ARTICLE
Human Gait Recognition Using Deep Learning and Improved Ant Colony Optimization
1 Department of Computer Science, HITEC University Taxila, Taxila, 47080, Pakistan
2 College of Computer Science and Engineering, University of Ha'il, Ha'il, Saudi Arabia
3 College of Computer Engineering and Sciences, Prince Sattam Bin Abdulaziz University, Al-Khraj, Saudi Arabia
4 Faculty of Applied Computing and Technology, Noroff University College, Kristiansand, Norway
5 Department of Applied Artificial Intelligence, Ajou University, Suwon, Korea
6 Department of Computer Science and Engineering, Soonchunhyang University, Asan, Korea
* Corresponding Author: Yunyoung Nam. Email:
(This article belongs to the Special Issue: Recent Advances in Deep Learning, Information Fusion, and Features Selection for Video Surveillance Application)
Computers, Materials & Continua 2022, 70(2), 2113-2130. https://doi.org/10.32604/cmc.2022.018270
Received 03 March 2021; Accepted 07 May 2021; Issue published 27 September 2021
Abstract
Human gait recognition (HGR) has received a lot of attention in the last decade as an alternative biometric technique. The main challenges in gait recognition are the change in in-person view angle and covariant factors. The major covariant factors are walking while carrying a bag and walking while wearing a coat. Deep learning is a new machine learning technique that is gaining popularity. Many techniques for HGR based on deep learning are presented in the literature. The requirement of an efficient framework is always required for correct and quick gait recognition. We proposed a fully automated deep learning and improved ant colony optimization (IACO) framework for HGR using video sequences in this work. The proposed framework consists of four primary steps. In the first step, the database is normalized in a video frame. In the second step, two pre-trained models named ResNet101 and InceptionV3 are selected and modified according to the dataset's nature. After that, we trained both modified models using transfer learning and extracted the features. The IACO algorithm is used to improve the extracted features. IACO is used to select the best features, which are then passed to the Cubic SVM for final classification. The cubic SVM employs a multiclass method. The experiment was carried out on three angles (0, 18, and 180) of the CASIA B dataset, and the accuracy was 95.2, 93.9, and 98.2 percent, respectively. A comparison with existing techniques is also performed, and the proposed method outperforms in terms of accuracy and computational time.Keywords
Cite This Article
Citations
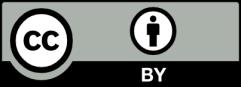