Open Access
ARTICLE
Incremental Learning Framework for Mining Big Data Stream
1 Information Systems Department, Faculty of Computers and Information, Mansoura University, Mansoura, 35516, Egypt
2 Machine Learning and Information Retrieval Department, Faculty of Artificial Intelligence, Kafrelsheikh University, Kafr El-Sheikh, Egypt
3 Department of Computer Science, College of Computers and Information Technology, Taif University, Taif, 21944, Saudi Arabia
* Corresponding Author: Mohammad Dahman Alshehri. Email:
Computers, Materials & Continua 2022, 71(2), 2901-2921. https://doi.org/10.32604/cmc.2022.021342
Received 29 June 2021; Accepted 30 July 2021; Issue published 07 December 2021
Abstract
At this current time, data stream classification plays a key role in big data analytics due to its enormous growth. Most of the existing classification methods used ensemble learning, which is trustworthy but these methods are not effective to face the issues of learning from imbalanced big data, it also supposes that all data are pre-classified. Another weakness of current methods is that it takes a long evaluation time when the target data stream contains a high number of features. The main objective of this research is to develop a new method for incremental learning based on the proposed ant lion fuzzy-generative adversarial network model. The proposed model is implemented in spark architecture. For each data stream, the class output is computed at slave nodes by training a generative adversarial network with the back propagation error based on fuzzy bound computation. This method overcomes the limitations of existing methods as it can classify data streams that are slightly or completely unlabeled data and providing high scalability and efficiency. The results show that the proposed model outperforms state-of-the-art performance in terms of accuracy (0.861) precision (0.9328) and minimal MSE (0.0416).Keywords
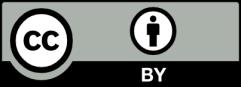