Open Access
ARTICLE
Efficient Image Captioning Based on Vision Transformer Models
1 Department of Data Science, Faculty of Artificial Intelligence, Kafrelsheikh University, Egypt
2 Department of Electrical Engineering, Faculty of Engineering, Kafrelsheikh University, Egypt
3 Department of Computer Science, Faculty of Computer and Information Science, Mansoura, Egypt
* Corresponding Author: Samar Elbedwehy. Email:
Computers, Materials & Continua 2022, 73(1), 1483-1500. https://doi.org/10.32604/cmc.2022.029313
Received 01 March 2022; Accepted 12 April 2022; Issue published 18 May 2022
Abstract
Image captioning is an emerging field in machine learning. It refers to the ability to automatically generate a syntactically and semantically meaningful sentence that describes the content of an image. Image captioning requires a complex machine learning process as it involves two sub models: a vision sub-model for extracting object features and a language sub-model that use the extracted features to generate meaningful captions. Attention-based vision transformers models have a great impact in vision field recently. In this paper, we studied the effect of using the vision transformers on the image captioning process by evaluating the use of four different vision transformer models for the vision sub-models of the image captioning The first vision transformers used is DINO (self-distillation with no labels). The second is PVT (Pyramid Vision Transformer) which is a vision transformer that is not using convolutional layers. The third is XCIT (cross-Covariance Image Transformer) which changes the operation in self-attention by focusing on feature dimension instead of token dimensions. The last one is SWIN (Shifted windows), it is a vision transformer which, unlike the other transformers, uses shifted-window in splitting the image. For a deeper evaluation, the four mentioned vision transformers have been tested with their different versions and different configuration, we evaluate the use of DINO model with five different backbones, PVT with two versions: PVT_v1and PVT_v2, one model of XCIT, SWIN transformer. The results show the high effectiveness of using SWIN-transformer within the proposed image captioning model with regard to the other models.Keywords
Cite This Article
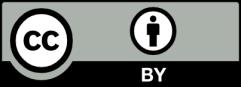