Open Access
ARTICLE
Fermatean Hesitant Fuzzy Prioritized Heronian Mean Operator and Its Application in Multi-Attribute Decision Making
1 School of Business Administration, Guangdong University of Finance and Economics, Guangzhou, 510320, China
2 Antai College of Economics and Management, Shanghai Jiaotong University, Shanghai 200030, China
3 Guangdong Institute of Scientific and Technical Information, Guangzhou, 510033, China
* Corresponding Author: Li-Na Han. Email:
Computers, Materials & Continua 2023, 75(2), 3203-3222. https://doi.org/10.32604/cmc.2023.035480
Received 23 August 2022; Accepted 15 January 2023; Issue published 31 March 2023
Abstract
In real life, incomplete information, inaccurate data, and the preferences of decision-makers during qualitative judgment would impact the process of decision-making. As a technical instrument that can successfully handle uncertain information, Fermatean fuzzy sets have recently been used to solve the multi-attribute decision-making (MADM) problems. This paper proposes a Fermatean hesitant fuzzy information aggregation method to address the problem of fusion where the membership, non-membership, and priority are considered simultaneously. Combining the Fermatean hesitant fuzzy sets with Heronian Mean operators, this paper proposes the Fermatean hesitant fuzzy Heronian mean (FHFHM) operator and the Fermatean hesitant fuzzy weighted Heronian mean (FHFWHM) operator. Then, considering the priority relationship between attributes is often easier to obtain than the weight of attributes, this paper defines a new Fermatean hesitant fuzzy prioritized Heronian mean operator (FHFPHM), and discusses its elegant properties such as idempotency, boundedness and monotonicity in detail. Later, for problems with unknown weights and the Fermatean hesitant fuzzy information, a MADM approach based on prioritized attributes is proposed, which can effectively depict the correlation between attributes and avoid the influence of subjective factors on the results. Finally, a numerical example of multi-sensor electronic surveillance is applied to verify the feasibility and validity of the method proposed in this paper.Keywords
Due to the limitations of decision makers’ cognition and professional differences, various uncertainties often appear in the comprehensive evaluation problems, and the traditional evaluation methods are not applicable or appropriate anymore. To solve the problem of comprehensive evaluation under uncertain circumstances, Zadeh [1] proposed the concept of fuzzy sets, and Atanassov [2] extended the fuzzy set and developed the concept of intuitionistic fuzzy set (IFS) that includes both the degree of membership and non-membership, which is a good approach for dealing with uncertainty. Recently, the similarity measures of improved intuitionistic hesitant fuzzy sets [3], which are essential techniques to cope with uncertainties and awkward information in practical issues, have arisen the attention of some scholars. Since the sum of the membership degree and non-membership degree of the IFS must be less than or equal to 1, it will be subject to many constraints in solving practical problems. Thus, Yager [4] defined a new form of fuzzy set named Pythagorean fuzzy set (PFS), where the sum of squares of the membership degree and non-membership degree is less than or equal to 1. The PFS has received more and more attention because it can characterize uncertain information more sufficiently and capture stronger fuzziness. Not only have its basic definition, operation rules, and fundamental properties been gradually improved but also there have been extensive studies on the aggregation operators, multi-attribute decision-making (MADM), extensions, information measures and other fields, such as Pythagorean fuzzy soft sets [5], interval-valued picture fuzzy sets [6], Pythagorean fuzzy Einstein aggregation operators [7], distance measures and similarity measures [8].
IFS and PFS have been widely applied to numerous fields in real life. However, they also have some limitations related to the membership and non-membership grades, so Riaz et al. [9] introduced the concept of linear Diophantine fuzzy set (LDFS) with the addition of reference parameters. This was gradually expanded into a variety of forms, including the spherical LDFS [10], the LDFS rough sets [11], LDFS Einstein aggregation operators [12], and so on. This type of set can not only relax the strict constraints of satisfaction and dissatisfaction grades but also classify a physical system with the help of reference parameters. Therefore, it is an effective tool for expressing the evaluation values of experts during the decision-making process. Later, Senapati et al. [13] put forward the Fermatean fuzzy set (FFS), which extends the evaluation information, weakens the data requirements for the membership degree and non-membership degree, and broadens the conditions such that the cubic sum is less than or equal to 1. Many scholars have started work in this direction and confirmed its scientificity and effectiveness [14–20], mainly focusing on its applications in MADM [14,15] or multicriteria decision-making (MCDM) [16–18] and the extensions such as Fermatean fuzzy linguistic sets [19] and interval-valued Fermatean fuzzy sets [20]. Due to the different knowledge and experience of decision-makers, the decision-making group tends to be hesitant when confronted with challenging problems, and the membership function of fuzzy sets allows assessment information to contain multiple possible values [21,22]. Torra [21] first defined the hesitant fuzzy set (HFS) in terms of a function that returns a set of membership values for each element and then expanded the membership from a single real-valued set to a multi-real-valued set, which is more helpful for dealing with ambiguity and uncertainty. So far, HFS has been extensively studied in the domain of aggregation operators and decision-making. A Fermatean hesitant fuzzy set (FHFS) is stemmed from the concept of FFS and HFS. Liu et al. [23] effectively combined FFS and HFS to achieve comprehensive evaluations of green restoration levels in five provinces and cities along the Yangtze River Economic Belt. Mishra et al. [24] proposed a generalized distance measure by merging FHFS with a modified VIKOR method. In addition, various models have been investigated to review the information under uncertainty. For instance, the soft multisets (SMS) and SMS topology have been extensively applied to soft computing, fuzzy modeling and decision-making. Riaz et al. [25] established several MCDM algorithms with aggregation operators based on the SMS topology. The rough set is a fundamental tool for dealing with incomplete and inaccurate information because of its inherent characteristics. Sahu et al. [26] proposed a rough set-based approach to help students choose an appropriate subject. Sun et al. [27] developed a new method based on the multilabel fuzzy neighborhood rough sets and maximum relevance and minimum redundancy to deal with data with missing labels.
In the comprehensive evaluation (decision) problems, the information aggregation operators have been applied to a variety of theoretical and practical studies related to FFSs. Among them, Senapati et al. [28] proposed the Fermatean fuzzy weighted average (FFWA) operator and Fermatean fuzzy weighted geometric (FFWG) operator and gave the application of them in MADM. Aydemir et al. [29] combined the Dombi aggregation operators with FFS and presented a Fermatean fuzzy TOPSIS decision-making method. By extending the Hamacher aggregation operators and the prioritized aggregation (PA) operator [30]. Jan et al. [31] proposed the Fermatean fuzzy Hamacher prioritized weighted average (FFHPWA) operator and Fermatean fuzzy Hamacher prioritized weighted geometric (FFHPWG) operator. Rani et al. [32,33] proposed several Fermatean fuzzy Heronian mean operators and Fermatean fuzzy Einstein information aggregation operators based on Heronian mean (HM) operators and Einstein aggregation operators, and then applied them to the choice of food waste treatment technology. Induced by the Hamacher operations and FFS, Hadi et al. [34] proposed several Fermatean fuzzy Hamacher arithmetic and geometric aggregation operators, such as Fermatean fuzzy Hamacher ordered weighted average (FFHOWA) operator, Fermatean fuzzy Hamacher hybrid weighted (FFHHW) operator and Fermatean fuzzy Hamacher ordered weighted geometric (FFHOWG) operator. In addition, Shit et al. [35] developed various Fermatean fuzzy Dombi aggregation operators and applied them to MADM. Based on FFSs and probabilistic hesitant fuzzy sets, Liu et al. [23] proposed the probabilistic hesitant Fermatean fuzzy Dombi Choquet integral geometric (PHFFDCIG) operator and the probabilistic hesitant Fermatean fuzzy Dombi Choquet integral average (PHFFDCIA) operator, which were applied to the evaluation of regional green restoration level. Since the Fermatean fuzzy linguistic (FFL) set theory provides an efficient tool for modeling a higher level of uncertain information, Verma [36] defined several new aggregation operators, including the FFL-weighted average (FFLWA) operator, FFL-weighted geometric (FFLWG) operator, FFL-ordered weighted average (FFLOWA) operator, FFL-hybrid average (FFLHA) operator, and so on. Akram et al. [37] proposed a series of Hamy-inspired operators based on 2-tuple linguistic Fermatean fuzzy information. With the popularization of FFSs, more and more scholars pay attention to the application in solving MCDM problems [38–40]. At present, FFSs have been extended to various fields since they are better able to describe fuzziness, such as blockchain platform evaluation [41], treatment of COVID-19 [42], green construction supplier evaluation [43] and pattern recognition [44]. To further extend FFSs, Gul et al. [45] proposed an improved TOPSIS methodology with FFSs and applied it to the real-life manufacturing risk evaluation problem. Further, Yang et al. [46] investigated the properties of continuous Fermatean fuzzy information and examined their continuity, derivatives and differentials. Akram et al. [47] defined a triangular interval-valued Fermatean fuzzy number and its arithmetic operations. Undoubtedly, these operators have been successfully applied in MCDM or MADM under a Fermatean fuzzy environment, but they also have several drawbacks in their applications. That is, they do not take the interrelationships between the attributes into account during the process of aggregation.
In real life, the lack of complete information, the inaccurate data, and the preferences of decision-makers during qualitative judgment would have a significant influence on the results. Most of the Fermatean fuzzy information aggregation methods mentioned above are proposed under the condition that the attributes are independent. However, in practical problems, there may be different degrees of correlation between the attributes, such as complementarity, redundancy and preference. HM operators can effectively capture the correlations between input variables and aggregate multiple variables into one variable. In the decision-making, decision-makers will inevitably encounter situations where the attributes are interrelated and influence each other. If conventional operators are still used for information aggregation, the results may be inaccurate. Considering the interconnection of numerous criteria or input attributes, the HM operator has attracted wide attention, which can effectively capture the correlation between input variables and aggregate multiple variables.
In recent years, many scholars have paid attention to extending the HM operator with different fuzzy environments and proposed numerous aggregation operators. Li et al. [48] studied the generalized HM operators under the Pythagorean fuzzy environment and put forward some Pythagorean fuzzy HM operators. Fan et al. [49] proposed two HM operators to aggregate the linguistic neutrosophic multisets and then discussed their properties. As for interval-valued fuzzy sets, Hu et al. [50] presented a study of MADM based on the correlations between attributes under the interval-valued intuitionistic fuzzy environment. Narang et al. [51] merged an improved generalized weighted HM operator and generalized geometric weighted HM operators with the traditional combined compromise solution (CoCoSo) method to present a new decision-making model. Since the neutrosophic cubic numbers (NCNs) can easily express incomplete, indeterminate and inconsistent information, Gulistan et al. [52] combined HM operators with NCNs. Additionally, HM operators have been extended to a variety of new forms in the process of MADM, such as cubic fuzzy HM Dombi aggregation operators [53], linguistic HM operators [54], and power generalized HM operators [55]. Most existing Fermatean fuzzy aggregation methods assume that the attribute weights are known. Considering that the priority relationship between attributes is often easier to obtain than the attribute weights, the prioritized aggregation (PA) operators [31] are effectively used to determine the attribute weights, which can make the decision-making results objective and fair. Chen et al. [56] proposed a prioritized measure-guided aggregation operator based on the ordered weighted average (OWA) operator and the Choquet integral. As an extension of PA operators, He et al. [57] used the priority labels to express the prioritized relationships between different criteria and then presented some scaled PA operators. The PA operators have always been one of the hot spots in MADM and MCDM problems and have also been applied to the intuitionistic fuzzy environment [58] and Pythagorean fuzzy environment [59,60]. To comprehensively take advantage of HM operators and PA operators, this paper developed a Fermatean hesitant fuzzy aggregation method based on these two operators.
From the literature review, this paper can draw the following conclusions. First, from the literature in the WOS database, FFSs have been preliminarily studied by scholars, mainly focusing on aggregation operators and their applications in MADM and the extensions, while few studies have aggregated the Fermatean fuzzy numbers based on HM operators. Second, as far as Fermatean hesitant fuzzy sets are concerned, the research is still at the initial stage. Thus, only a few papers have shown relevant investigations, and even fewer scholars have studied the combination of FHFS and HM operators. Finally, as a special generalization of the Bonferroni mean operator, HM operators have attracted significant attention from scholars and have been expanded into a variety of forms, such as linguistic Pythagorean HM operators, cubic HM operators, power generalized HM operators, and so on [48,52,55]. In addition, many authors have proposed MCDM approaches with these operators above. Li et al. [48] extended the generalized HM operators to the Pythagorean fuzzy context and developed several Pythagorean fuzzy HM operators. Later, Fan et al. [49] introduced four generalized HM operators for bipolar neutrosophic numbers and discussed their properties. However, most of the existing Fermatean fuzzy information aggregation methods assume that the attribute weights are known. Although the priority relationship between attributes is often easier to obtain than the attribute weights, there is almost no research concerning the prioritized HM operators.
To sum up, the main contributions and theoretical innovations of this paper are as follows. On the one hand, this paper introduces hesitance on the basis of Fermatean fuzziness and fully considers the impact of hesitant fuzzy data on decision-making, which can effectively address the uncertainty of associated attributes under the Fermatean fuzzy environment. On the other hand, in order to effectively use prioritized operators to determine the attribute weights and ensure more objective and accurate results, this paper proposes the HM operator with the priority relationship, which further improves the theoretical system of the aggregation operators of FFSs. Additionally, the attributes tend to be related to each other rather than independent of each other in MADM problems, and the interactions will directly affect the results. The method proposed in this paper can effectively describe the correlation between attributes and avoid the influence of subjective factors on the results, so it has a critical reference significance for decision-making in reality. Therefore, this paper presents some aggregation operators under the Fermatean fuzzy environment for MADM and provides a scientific approach for information evaluation when attributes are associated with each other.
To further expand the application of HM operators and PA operators in a new fuzzy environment, the remainder of this paper is organized as follows. Some basic concepts and operational rules of FFS are defined in Section 2. Section 3 extends HM operators to the Fermatean fuzzy set, and introduces the Fermatean hesitant fuzzy Heronian mean (FHFHM) operator and the Fermatean hesitant fuzzy weighted Heronian mean (FHFWHM) operator, followed by related properties. In Section 4, the paper proposes the Fermatean hesitant fuzzy prioritized Heronian mean (FHFPHM) operator based on the FHFWHM operator and PA operators. In Section 5, the paper proposes a MADM method based on the FHFPHM operator and utilizes a numerical example to verify the method proposed in this paper. Section 6 concludes the paper with some remarks.
2 Preliminaries and Basic Theory
In this section, some basic concepts and operational rules about the HFS, IFS, PFS, FFS, and the HM operator are recalled briefly.
Definition 1 [21]: Assume
Definition 2 [2]: Let
where
Definition 3 [4]: The PFS defined on a non-empty set
where
Definition 4 [13]: Let
where
Definition 5 [31]: Let
The larger the score function, the better the FFS. In particular, researchers can further compare the accuracy functions if the score functions are the same. The FFS is superior when the accuracy function is more prominent.
According to the properties of FFS, Senapati et al. proposed several basic algorithms.
Definition 6 [28]: Let
1)
2)
3)
4)
Definition 7 [47]: Assume that
Considering the influence of priority, Yager defined the PA operator as follows [31].
Definition 8 [31]: Let
where
3 Fermatean Hesitant Fuzzy Aggregation Operators Based on the Heronian Mean Operator
Since the membership and non-membership degrees of FFS often show fuzziness in many cases, this section not only proposes the concept of Fermatean hesitant fuzzy set (FHFS) but also puts forward the Fermatean hesitant fuzzy Heronian mean (FHFHM) operator combined with the HM operators.
Definition 9: The Fermatean hesitant fuzzy set interpreted on a non-empty set
where
Definition 10: Let
Theorem 1: Assume that
Proof: According to Definition 4, the following conclusions can be drawn.
Then
So,
Therefore,
The FHFHM operator satisfies the following excellent properties: idempotency, monotonicity, invariance, boundedness, and so on.
Property 1 (Idempotency): Let
Property 2 (Monotonicity): Let
when
Proof: Since
Similarly, the non-membership degree can be concluded as follows:
According to the comparison rules of Definition 3 , it can be inferred that
Property 3 (Invariance): Assume
where
Property 4 (Boundedness): Let
Then,
Proof: Refer to the proof process of Properties 1–3, this is easily proven.
4 The Fermatean Hesitant Fuzzy Prioritized Heronian Mean Operator
Although the attribute weights need to be taken into account during the MADM process, the FHFHM operator mentioned above does not actually consider this effect. Therefore, the Fermatean hesitant fuzzy weighted Heronian mean (FHFWHM) operator is defined. Compared with the weight of attributes, the priority relationship between attributes is easier to obtain. This paper further combines the FHFWHM operator with PA operators and proposes a Fermatean hesitant fuzzy prioritized Heronian mean (FHFPHM) operator.
Definition 11: Let
Then
is termed as a Fermatean hesitant fuzzy weighted Heronian mean (FHFWHM) operator.
Definition 12: Let
where
Theorem 2: Assume that
Proof: The proof process is similar to that of Theorem 1.
The FHFPHM operator still satisfies the following properties: idempotency, monotonicity, invariance, boundedness, and so on.
Property 5 (Idempotency): Assume
Property 6 (Monotonicity): Let
Proof: Refer to the proof process of Property 2.
Property 7 (Invariance): Let
where
Property 8 (Boundedness): Let
Then
Proof: Refer to the proof process of Property 4.
5 MADM Based on Fermatean Hesitant Information and Its Application
5.1 MADM Based on Fermatean Hesitant Information
Based on the FHFPHM operator, this section presents a Fermatean hesitant fuzzy decision-making method with attribute priorities. Let
Step 1: The comprehensive score of the scheme
Step 2: Using the FHFPHM operator, the Fermatean hesitant fuzzy preference information of alternative
Step 3: The composite score of alternatives is calculated according to Definition 3.
The rankings of alternatives are generated by the descending of the composite scores.
This section applied the proposed Fermatean hesitant fuzzy MADM approach to the multi-sensor electronic reconnaissance decision-making problem. In this problem, the paper uses the multi-attribute information of the enemy’s pulse repetition frequency, carrier frequency, power and pulse width measured by the Radar, ESM, infrared and other sensors to determine the type of the enemy's airborne platforms. However, in the complex electromagnetic environment, electronic reconnaissance equipment frequently experiences interference from airborne clutter and enemy signals during the measurement process, which leads to certain information uncertainty. This conforms to the characteristics of Fermatean hesitant fuzzy numbers. Therefore, it is appropriate to select FHFS to make relevant decisions.
Assume that there are four types of airborne platforms reported to the fusion center by each electronic reconnaissance sensor, and each of them has four types of attributes:
Step 1: Calculate the comprehensive scores by Definition 5, and then use Definition 12 to calculate the attribute weights with priority relationships, as shown in Table 2.
Step 2: Using the FHFPHM operator, the preference information of alternatives
Step 3: According to Definition 5, all the alternatives are ranked and the results are listed in Table 4.
When
The results show that when the parameters
To illustrate the rationality of the FHFPHM operator, this paper further compares it with the Fermatean fuzzy weighted average (FFWA) operator and the Fermatean fuzzy weighted power average (FFWPA) operator proposed by Senapati et al. [28], and the dual hesitant fuzzy geometric weighted Heronian mean (DHFGHM) operator developed by Qu et al. [61].
Definition 13 [28]: Let
Since the evaluation data in Table 1 also abides by the characteristics of dual hesitant fuzzy sets, the dual hesitant fuzzy geometric weighted Heronian mean (DHFGWHM) operator proposed by Qu et al. [61] can also be used for calculation. The formula is given as follows:
Definition 14 [61]: Let
References [28] and [61] assume that the attribute weights are known. To facilitate the comparative analysis, the attribute weights calculated by the method proposed in this paper (as shown in Table 2) are used for calculation here. Based on the FFWA, FFWPA, and DHFGWHM operators, the information aggregation results for each scheme are obtained in Table 5.
It can be seen from Table 5 that the results obtained by the method proposed in this paper are different from the results obtained in reference [28]. The main reason may lie in whether or not the interactions between the attributes are considered. Additionally, the results obtained in this paper are basically consistent with those in reference [61]. This paper not only considered the problem of attribute correlations during the ranking process but also used the score function and accuracy function to compare different parameters, which makes the results comprehensive and scientific. However, compared with dual hesitant fuzzy sets, the application range of the obtained method is much broader since it allows the cubic sum of the membership degree and non-membership degree to be less than 1. References [28] and [61] both assume that the attribute weights are known, but when solving practical problems, it is difficult to determine the weights of attributes, while the priority relationship between attributes is relatively easier to obtain. Therefore, the proposed method is consistent with actual situations, reasonable, and comprehensive.
In this paper, a novel approach is proposed to solve MADM problems, where the membership degree, non-membership degree and priority relationship are considered simultaneously. First, the paper introduced the Fermatean hesitant fuzzy Heronian mean (FHFHM) operator and Fermatean hesitant fuzzy weighted Heronian mean (FHFWHM) operator and discussed their related properties such as idempotency, monotonicity, boundedness. Then considering that the attribute priority is often easier to obtain than the attribute weight, the paper proposed a method of MADM based on the Fermatean hesitant fuzzy prioritized Heronian mean (FHFPHM) operator under the Fermatean fuzzy environment. This method effectively combines Fermatean fuzzy information and hesitant fuzzy information, improves the application scope of the membership degree and the non-membership degree, and reflects the personal preference and hesitancy degree of experts in the process of multi-attribute group decision-making. This makes the results of information aggregation accurate, scientific, and rational. Furthermore, the paper took the priority problems between attributes into consideration, which effectively improves the comprehensiveness of the results and makes them more scientific. Then a numerical example of multi-sensor electronic reconnaissance was implemented. The results demonstrate that the ordering of the alternatives may be different for the different values
In future researches, researches can introduce more factors beyond the priority relationship based on the combination of FFS and HFS, such as distance measures, similarity measures, and correlation coefficient measures. Also, it is suggested to merge the MADM method with q-rung orthopair fuzzy sets, neutrosophic cubic numbers and soft rough sets to enrich and improve the theoretical system of FFSs. Additionally, we will focus on extending more types of aggregation operators and also apply the developed methodology to more fields, such as the performance evaluation of internal departments, supplier capability assessment in green supply chains and science-technology project assessment.
Funding Statement: The authors received no specific funding for this study.
Conflicts of Interest: The authors declare that they have no conflicts of interest to report regarding the present study.
References
1. L. A. Zadeh, “Fuzzy sets,” Information and Control, vol. 8, no. 3, pp. 338–353, 1965. [Google Scholar]
2. K. T. Atanassov, “Intuitionistic fuzzy sets,” Fuzzy Sets and Systems, vol. 20, no. 1, pp. 87–96, 1986. [Google Scholar]
3. T. Mahmood, W. Ali, Z. Ali and P. Chinram, “Power aggregation operators and similarity measures based on improved intuitionistic hesitant fuzzy sets and their applications to multiple attribute decision making,” Computer Modeling in Engineering & Sciences, vol. 126, no. 3, pp. 1165–1187, 2021. [Google Scholar]
4. R. R. Yager, “Pythagorean fuzzy subsets,” in 2013 Joint IFSA World Congress and NAFIPS Annual Meeting (IFSA/NAFIPS), Edmonton, Canada, pp. 57–61, 2013. [Google Scholar]
5. R. M. Zulqarnain, X. L. Xin, H. Garg and R. Ali, “Interaction aggregation operators to solve multi criteria decision making problem under Pythagorean fuzzy soft environment,” Journal of Intelligent & Fuzzy Systems, vol. 40, no. 1, pp. 1151–1171, 2021. [Google Scholar]
6. A. Ashraf, K. Ullah, A. Hussain and M. Bari, “Interval-valued picture fuzzy Maclaurin symmetric mean operator with application in multiple attribute decision-making,” Reports in Mechanical Engineering, vol. 3, no. 1, pp. 301–317, 2022. [Google Scholar]
7. Z. Ali, T. Mahmood, K. Ullah and Q. Khan, “Einstein geometric aggregation operators using a novel complex interval-valued Pythagorean fuzzy setting with application in green supplier chin management,” Reports in Mechanical Engineering, vol. 2, no. 1, pp. 105–134, 2021. [Google Scholar]
8. X. Peng, “New similarity measure and distance measure for Pythagorean fuzzy set,” Complex & Intelligent Systems, vol. 5, no. 2, pp. 101–111, 2019. [Google Scholar]
9. M. Riaz and M. R. Hashmi, “Linear Diophantine fuzzy set and its applications towards multi-attribute decision-making problems,” Journal of Intelligent & Fuzzy Systems, vol. 37, no. 4, pp. 5417–5439, 2019. [Google Scholar]
10. M. Riaz, M. R. Hashmi, D. Pamucar and Y. M. Chu, “Spherical linear Diophantine fuzzy sets with modeling uncertainties in MCDM,” Computer Modeling in Engineering & Sciences, vol. 126, no. 3, pp. 1125–1164, 2021. [Google Scholar]
11. M. R. Hashmi, S. T. Tehrim, M. Riaz, D. Pamucar and G. Cirovic, “Spherical linear Diophantine fuzzy soft rough sets with multi-criteria decision making,” Axioms, vol. 10, no. 3, pp. 1–24, 2021. [Google Scholar]
12. H. M. A. Farid, M. Riaz, M. J. Khan, P. Kumam and K. Sitthithakerngkiet, “Sustainable thermal power equipment supplier selection by Einstein prioritized linear Diophantine fuzzy aggregation operators,” AIMS Mathematics, vol. 7, no. 6, pp. 11201–11242, 2022. [Google Scholar]
13. T. Senapati and R. R. Yager, “Fermatean fuzzy sets,” Journal of Ambient Intelligence and Humanized Computing, vol. 11, no. 2, pp. 663–674, 2020. [Google Scholar]
14. M. Keshavarz-Ghorabaee, M. Amiri, M. Hashemi-Tabatabaei, E. K. Zavadskas and A. Kaklauskas, “A new decision-making approach based on Fermatean fuzzy sets and WASPAS for green construction supplier evaluation,” Mathematics, vol. 8, no. 12, Article ID 2202, 2020. [Google Scholar]
15. G. Ali and M. N. Ansari, “Multi-attribute decision-making under Fermatean fuzzy bipolar soft framework,” Granular Computing, vol. 7, no. 2, pp. 337–352, 2022. [Google Scholar]
16. A. R. Mishra and P. Rani, “Multi-criteria healthcare waste disposal location selection based on Fermatean fuzzy WASPAS method,” Complex & Intelligent Systems, vol. 7, no. 5, pp. 2469–2484, 2021. [Google Scholar]
17. S. Biswas, D. Pamucar, S. Kar and S. S. Sana, “A new integrated FUCOM-CODAS framework with Fermatean fuzzy information for multi-criteria group decision-making,” Symmetry, vol. 13, no. 12, pp. 1–36, 2021. [Google Scholar]
18. Z. Deng and J. Y. Wang, “Evidential Fermatean fuzzy multicriteria decision-making based on Fermatean fuzzy entropy,” International Journal of Intelligent Systems, vol. 36, no. 10, pp. 5866–5886, 2021. [Google Scholar]
19. D. H. Liu, Y. Y. Liu and X. H. Chen, “Fermatean fuzzy linguistic set and its application in multicriteria decision making,” International Journal of Intelligent Systems, vol. 34, no. 5, pp. 878–894, 2019. [Google Scholar]
20. S. Jeevaraj, “Ordering of interval-valued Fermatean fuzzy sets and its applications,” Expert Systems with Applications, vol. 185, no. 3, Article ID 115613, pp. 1–20, 2021. [Google Scholar]
21. V. Torra, “Hesitant fuzzy sets,” International Journal of Intelligent Systems, vol. 25, no. 6, pp. 529–539, 2010. [Google Scholar]
22. B. Zhu, “Research on decision-making method and application based on preference relationship,” Ph.D. dissertation., Southeast University, Nanjing, 2014. [Google Scholar]
23. J. Liu and S. H. Luo, “Probabilistic hesitant Fermatean fuzzy extension MULTIMOORA method for evaluation of regional green restoration leve,” Control and Decision, vol. 37, no. 10, pp. 2685–2695, 2022. [Google Scholar]
24. A. R. Mishra, S. M. Chen and P. Rani, “Multiattribute decision making based on Fermatean hesitant fuzzy sets and modified VIKOR method,” Information Sciences, vol. 607, no. 4, pp. 1532–1549, 2021. [Google Scholar]
25. M. Riaz, N. Çagman, N. Wali and A. Mushtaq, “Certain properties of soft multi-set topology with applications in multi-criteria decision making,” Decision Making: Applications in Management and Engineering, vol. 3, no. 2, pp. 70–96, 2020. [Google Scholar]
26. R. Sahu, S. R. Dash and S. Das, “Career selection of students using hybridized distance measure based on picture fuzzy set and rough set theory,” Decision Making: Applications in Management and Engineering, vol. 4, no. 1, pp. 104–126, 2021. [Google Scholar]
27. L. Sun, T. Yin, W. Ding, Y. Qian and J. Xu, “Feature selection with missing labels using multilabel fuzzy neighborhood rough sets and maximum relevance minimum redundancy,” IEEE Transactions on Fuzzy Systems, vol. 30, no. 5, pp. 1197–1211, 2021. [Google Scholar]
28. T. Senapati and R. R. Yager, “Fermatean fuzzy weighted averaging/geometric operators and its application in multi-criteria decision-making methods,” Engineering Applications of Artificial Intelligence, vol. 85, no. S1, pp. 112–121, 2019. [Google Scholar]
29. S. B. Aydemir and S. Yilmaz Gunduz, “Fermatean fuzzy TOPSIS method with Dombi aggregation operators and its application in multi-criteria decision making,” Journal of Intelligent & Fuzzy Systems, vol. 39, no. 1, pp. 851–869, 2020. [Google Scholar]
30. R. R. Yager, “Prioritized aggregation operators,” International Journal of Approximate Reasoning, vol. 48, no. 1, pp. 263–274, 2008. [Google Scholar]
31. A. Jan, A. Khan, W. Khan and M. Afridi, “A novel approach to MADM problems using Fermatean fuzzy Hamacher prioritized aggregation operators,” Soft Computing, vol. 25, no. 22, pp. 13897–13910, 2021. [Google Scholar]
32. P. Rani, A. R. Mishra, A. Saha, I. M. Hezam and D. Pamucar, “Fermatean fuzzy Heronian mean operators and MEREC-based additive ratio assessment method: An application to food waste treatment technology selection,” International Journal of Intelligent Systems, vol. 37, no. 3, pp. 2612–2674, 2022. [Google Scholar]
33. P. Rani and A. R. Mishra, “Fermatean fuzzy Einstein aggregation operators-based MULTIMOORA method for electric vehicle charging station selection,” Expert Systems with Applications, vol. 182, no. 2, pp. 1–23, 2021. [Google Scholar]
34. A. Hadi, W. Khan and A. Khan, “A novel approach to MADM problems using Fermatean fuzzy Hamacher aggregation operators,” International Journal of Intelligent Systems, vol. 36, no. 7, pp. 3464–3499, 2021. [Google Scholar]
35. C. Shit and G. Ghorai, “Multiple attribute decision-making based on different types of Dombi aggregation operators under Fermatean fuzzy information,” Soft Computing, vol. 25, no. 22, pp. 13869–13880, 2021. [Google Scholar]
36. R. Verma, “A decision-making approach based on new aggregation operators under fermatean fuzzy linguistic information environment,” Axioms, vol. 10, no. 2, pp. 1–39, 2021. [Google Scholar]
37. M. Akram, R. Bibi and M. M. Ali Al-Shamiri, “A decision-making framework based on 2-tuple linguistic Fermatean fuzzy Hamy mean operators,” Mathematical Problems in Engineering, vol. 2022, Article ID 1501880, pp. 1–29, 2022. [Google Scholar]
38. A. H. Ganie, “Multicriteria decision-making based on distance measures and knowledge measures of Fermatean fuzzy sets,” Granular Computing, vol. 7, no. 4, pp. 1–20, 2022. [Google Scholar]
39. M. Akram, G. Muhiuddin and G. Santos-Garcıa, “An enhanced VIKOR method for multi-criteria group decision-making with complex Fermatean fuzzy sets,” Mathematical Biosciences and Engineering, vol. 19, no. 7, pp. 7201–7231, 2022. [Google Scholar] [PubMed]
40. L. P. Zhou, S. P. Wan and J. Y. Dong, “A Fermatean fuzzy ELECTRE method for multi-criteria group decision-making,” Informatica, vol. 33, no. 1, pp. 181–224, 2022. [Google Scholar]
41. H. Lai, H. Liao, Y. Long and E. K. Zavadskas, “A hesitant Fermatean fuzzy CoCoSo method for group decision-making and an application to blockchain platform evaluation,” International Journal of Fuzzy Systems, vol. 24, no. 6, pp. 2643–2661, 2022. [Google Scholar]
42. S. Gül, “Fermatean fuzzy set extensions of SAW, ARAS, and VIKOR with applications in COVID-19 testing laboratory selection problem,” Expert Systems, vol. 38, no. 8, Article ID 1501880, pp. 1–16, 2021. [Google Scholar]
43. D. M. Wei, D. Meng, Y. Rong, Y. Liu, H. Garg et al., “Fermatean fuzzy Schweizer-Sklar operators and BWM-entropy-based combined compromise solution approach: An application to green supplier selection,” Entropy, vol. 24, no. 6, pp. 1–32, 2022. [Google Scholar]
44. P. Ejegwa and D. Zuakwagh, “Fermatean fuzzy modified composite relation and its application in pattern recognition,” Journal of Fuzzy Extension and Applications, vol. 3, no. 2, pp. 140–151, 2022. [Google Scholar]
45. M. Gul, H. W. Lo and M. Yucesan, “Fermatean fuzzy TOPSIS-based approach for occupational risk assessment in manufacturing,” Complex & Intelligent Systems, vol. 7, no. 5, pp. 2635–2653, 2021. [Google Scholar]
46. Z. Yang, H. Garg and X. Li, “Differential calculus of Fermatean fuzzy functions: Continuities, derivatives, and differentials,” International Journal of Computational Intelligence Systems, vol. 14, no. 1, pp. 282–294, 2021. [Google Scholar]
47. M. Akram, S. M. U. Shah, M. M. A. Al-Shamiri and S. A. Edalatpanah, “Fractional transportation problem under interval-valued Fermatean fuzzy sets,” AIMS Mathematics, vol. 7, no. 9, pp. 17327–17348, 2022. [Google Scholar]
48. Z. Li and G. Wei, “Pythagorean fuzzy heronian mean operators in multiple attribute decision making and their application to supplier selection,” International Journal of Knowledge-Based and Intelligent Engineering Systems, vol. 23, no. 2, pp. 77–91, 2019. [Google Scholar]
49. C. Fan, K. Hu, S. Feng, J. Ye and E. Fan, “Heronian mean operators of linguistic neutrosophic multisets and their multiple attribute decision-making methods,” International Journal of Distributed Sensor Networks, vol. 15, no. 4, Article ID 1550147719843059, pp. 1–12, 2019. [Google Scholar]
50. X. Hu, S. Yang and Y. R. Zhu, “Multiple-attribute decision making based on interval-valued intuitionistic fuzzy generalized weighted Heronian mean,” Information, vol. 13, no. 3, pp. 1–30, 2022. [Google Scholar]
51. M. Narang, M. C. Joshi, K. Bisht and A. Pal, “Stock portfolio selection using a new decision-making approach based on the integration of fuzzy CoCoSo with Heronian mean operator,” Decision Making: Applications in Management and Engineering, vol. 5, no. 1, pp. 90–112, 2022. [Google Scholar]
52. M. Gulistan, M. Mohammad, F. Karaaslan, S. Kadry, S. Khan et al., “Neutrosophic cubic Heronian mean operators with applications in multiple attribute group decision-making using cosine similarity functions,” International Journal of Distributed Sensor Networks, vol. 15, no. 9, pp. 1–21, 2019. [Google Scholar]
53. S. Ayub, S. Abdullah, F. Ghani, M. Qiyas and M. Yaqub Khan, “Cubic fuzzy Heronian mean Dombi aggregation operators and their application on multi-attribute decision-making problem,” Soft Computing, vol. 25, no. 6, pp. 4175–4189, 2021. [Google Scholar]
54. X. Deng, J. Wang and G. Wei, “Some 2-tuple linguistic Pythagorean Heronian mean operators and their application to multiple attribute decision-making,” Journal of Experimental & Theoretical Artificial Intelligence, vol. 31, no. 4, pp. 555–574, 2019. [Google Scholar]
55. D. Ju, Y. Ju and A. Wang, “Multi-attribute group decision making based on power generalized Heronian mean operator under hesitant fuzzy linguistic environment,” Soft Computing, vol. 23, no. 11, pp. 3823–3842, 2019. [Google Scholar]
56. L. Chen and Z. A. Xu, “Prioritized aggregation operator based on the OWA operator and prioritized measure,” Journal of Intelligent & Fuzzy Systems, vol. 27, no. 3, pp. 1297–1307, 2014. [Google Scholar]
57. Y. D. He, H. Y. Chen, Z. He, G. D. Wang and L. G. Zhou, “Scaled prioritized aggregation operators and their applications to decision making,” Soft Computing, vol. 20, no. 3, pp. 1021–1039, 2016. [Google Scholar]
58. R. Arora and H. Garg, “Group decision-making method based on prioritized linguistic intuitionistic fuzzy aggregation operators and its fundamental properties,” Computational and Applied Mathematics, vol. 38, no. 2, pp. 1–32, 2019. [Google Scholar]
59. M. S. A. Khan, S. Abdullah, A. Ali and F. Amin, “Pythagorean fuzzy prioritized aggregation operators and their application to multi-attribute group decision making,” Granular Computing, vol. 4, no. 2, pp. 249–263, 2019. [Google Scholar]
60. M. Akram, X. Peng, A. N. Al-Kenani and A. Sattar, “Prioritized weighted aggregation operators under complex Pythagorean fuzzy information,” Journal of Intelligent & Fuzzy Systems, vol. 39, no. 3, pp. 4763–4783, 2020. [Google Scholar]
61. G. H. Qu, B. Y. Wang, W. H. Qu, Z. S. Xu and Q. Zhang, “A multiple-attribute decision-making method based on the dual hesitant fuzzy geometric heronian means operator and its application,” Chinese Journal of Management Science, vol. 29, no. 1, pp. 217–225, 2021. [Google Scholar]
IFS | Intuitionistic fuzzy set |
PFS | Pythagorean fuzzy set |
LDFS | Linear Diophantine fuzzy set |
FFS | Fermatean fuzzy set |
HFS | Hesitant fuzzy set |
FHFS | Fermatean hesitant fuzzy set |
VIKOR | VlseKriterijumska Optimizacija IKompromisno Resenje |
FFWA | Fermatean fuzzy weighted average operator |
FFWG | Fermatean fuzzy weighted geometric operator |
MADM | Multi-attribute decision making |
PA | Prioritized aggregation operator |
FFHPWA | Fermatean fuzzy Hamacher priority weighted average operator |
HHHPWG | Fermatean fuzzy Hamacher priority weighted geometric operator |
HM | Heronian mean operator |
FFHIWG | Fermatean fuzzy Hammas interactive weighted geometric operator |
FFHIOWG | Fermatean fuzzy Hammas interactive ordered weighted geometric operator |
FFHIHWG | Fermatean fuzzy Hammas interactive hybrid weighted geometric operator |
PHFFDCIG | Probabilistic hesitant Fermatean fuzzy Dombi Choquet integral geometric operator |
PHFFDCIA | Probabilistic hesitant Fermatean fuzzy Dombi Choquet integral average operator |
TOPSIS | Technique for Order Preference by Similarity to an Ideal Solution |
IVIFS | Interval-valued intuitionistic fuzzy set |
CoCoSo | Combined compromise solution |
NCN | Neutrosophic cubic number |
FHFHM | Fermatean hesitant fuzzy Heronian mean operator |
FHFWHM | Fermatean hesitant fuzzy weighted Heronian mean operator |
FHFPHM | Fermatean hesitant fuzzy priority Heronian mean operator |
FFWPA | Fermatean fuzzy weighted power average operator |
DHFGWHM | Dual hesitant fuzzy geometric weighted Heronian mean operator |
Cite This Article
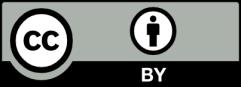