Open Access
ARTICLE
Improving Speech Enhancement Framework via Deep Learning
1 Department of Information Technology, Takming University of Science and Technology, Taipei City, 11451, Taiwan
2 Department of Electrical Engineering, National Chin-Yi University of Technology, Taichung, 411030, Taiwan
* Corresponding Author: Wen-Tsai Sung. Email:
Computers, Materials & Continua 2023, 75(2), 3817-3832. https://doi.org/10.32604/cmc.2023.037380
Received 01 November 2022; Accepted 06 January 2023; Issue published 31 March 2023
Abstract
Speech plays an extremely important role in social activities. Many individuals suffer from a “speech barrier,” which limits their communication with others. In this study, an improved speech recognition method is proposed that addresses the needs of speech-impaired and deaf individuals. A basic improved connectionist temporal classification convolutional neural network (CTC-CNN) architecture acoustic model was constructed by combining a speech database with a deep neural network. Acoustic sensors were used to convert the collected voice signals into text or corresponding voice signals to improve communication. The method can be extended to modern artificial intelligence techniques, with multiple applications such as meeting minutes, medical reports, and verbatim records for cars, sales, etc. For experiments, a modified CTC-CNN was used to train an acoustic model, which showed better performance than the earlier common algorithms. Thus a CTC-CNN baseline acoustic model was constructed and optimized, which reduced the error rate to about 18% and improved the accuracy rate.Keywords
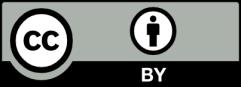