Open Access
ARTICLE
RRCNN: Request Response-Based Convolutional Neural Network for ICS Network Traffic Anomaly Detection
1 Country School of Cybersecurity, Chengdu University of Information Technology, Chengdu, 610225, China
2 Advanced Cryptography and System Security Key Laboratory of Sichuan Province, Chengdu, 610225, China
3 DAQSOFT CO., LTD, Chengdu, 610213, China
4 Communication and Information Technology Center, Petro China Southwest Oil and Gas Company, Chengdu, 610057, China
5 School of Computing and Information, University of Pittsburgh, Pittsburgh, 152607, PA, USA
* Corresponding Author: Shibin Zhang. Email:
Computers, Materials & Continua 2023, 75(3), 5743-5759. https://doi.org/10.32604/cmc.2023.035919
Received 09 September 2022; Accepted 19 November 2022; Issue published 29 April 2023
Abstract
Nowadays, industrial control system (ICS) has begun to integrate with the Internet. While the Internet has brought convenience to ICS, it has also brought severe security concerns. Traditional ICS network traffic anomaly detection methods rely on statistical features manually extracted using the experience of network security experts. They are not aimed at the original network data, nor can they capture the potential characteristics of network packets. Therefore, the following improvements were made in this study: (1) A dataset that can be used to evaluate anomaly detection algorithms is produced, which provides raw network data. (2) A request response-based convolutional neural network named RRCNN is proposed, which can be used for anomaly detection of ICS network traffic. Instead of using statistical features manually extracted by security experts, this method uses the byte sequences of the original network packets directly, which can extract potential features of the network packets in greater depth. It regards the request packet and response packet in a session as a Request-Response Pair (RRP). The feature of RRP is extracted using a one-dimensional convolutional neural network, and then the RRP is judged to be normal or abnormal based on the extracted feature. Experimental results demonstrate that this model is better than several other machine learning and neural network models, with F1, accuracy, precision, and recall above 99%.Keywords
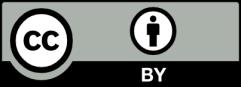