Open Access
ARTICLE
Pythagorean Neutrosophic Planar Graphs with an Application in Decision-Making
1 Department of Mathematics, Sacred Heart College (Autonomous), Tirupattur, 635601, Tamilnadu, India
2 Department of Mathematics, St. Joseph’s College of Engineering, OMR, Chennai, 600119, Tamilnadu, India
3 Department of Mathematics, Faculty of Science and Arts, King Khalid University, Muhayl Assir, Saudi Arabia
4 Department of Mathematics and Computer, Faculty of Science, Ibb University, Ibb, Yemen
* Corresponding Author: P. Chellamani. Email:
Computers, Materials & Continua 2023, 75(3), 4935-4953. https://doi.org/10.32604/cmc.2023.036321
Received 26 September 2022; Accepted 08 February 2023; Issue published 29 April 2023
Abstract
Graph theory has a significant impact and is crucial in the structure of many real-life situations. To simulate uncertainty and ambiguity, many extensions of graph theoretical notions were created. Planar graphs play a vital role in modelling which has the property of non-crossing edges. Although crossing edges benefit, they have some drawbacks, which paved the way for the introduction of planar graphs. The overall purpose of the study is to contribute to the conceptual development of the Pythagorean Neutrosophic graph. The basic methodology of our research is the incorporation of the analogous concepts of planar graphs in the Pythagorean Neutrosophic graphs. The significant finding of our research is the introduction of Pythagorean Neutrosophic Planar graphs, a conceptual blending of Pythagorean Neutrosophic and Planar graphs. The idea of Pythagorean Neutrosophic multigraphs and dual graphs are also introduced to deal with the ambiguous situations. This paper investigates the Pythagorean Neutrosophic planar values, which form the edges of the Pythagorean neutrosophic graphs. The concept of Pythagorean Neutrosophic dual graphs, isomorphism, co-weak and weak isomorphism have also been explored for Pythagorean Neutrosophic planar graphs. A decision-making algorithm was proposed with a numerical illustration by using the Pythagorean Neutrosophic fuzzy graph.Keywords
Graphs are illustrative representations that express the relation between objects and their data. When the relationships are ambiguous, a graph can be implemented as a fuzzy graph model, which has the same structure as a crisp graph but works with ambiguous data. The fuzzy set theory for dealing with incomplete and vague information originated from the work of Zadeh [1]. Following the fuzzy sets, Intuitionistic sets [2], in which the elements possess membership
Kaufmann [5], based on fuzzy relation [6], developed the idea of Fuzzy Graphs (FGs). Later, Rosenfeld [7] defined the basic properties of fuzzy relations, which are generalized with a fuzzy set as a base set, and fuzzy analogs of graphic theoretical concepts like bridges and trees were established with their properties. Bhattacharya [8] introduced the notions of eccentricity, and center explored how a fuzzy group can be associated with a fuzzy graph. Fundamental operations on FGs and their properties were discussed by Mordeson et al. in [9].
Shannon et al. introduced intuitionistic fuzzy relations in [10], and intuitionistic fuzzy graphs (IFG) with their properties were investigated in [11]. The operations of IFGs were described by Parvathi et al. in [12]. In [13,14], Akram et al. established ideas such as strong IFGs and IF hypergraphs. Pythagorean Fuzzy Graphs (PFGs) and their applications were explored by Naz et al. in [15], and the energy of a Pythagorean Fuzzy Graph (PFG) was studied by Akram et al. in [16], followed by the assertion of some PFG operations by Akram et al. in [17]. Akram et al. [18] proposed certain graphs using the base Pythagorean and the abstraction of the fuzzy dual graph with the investigation of its properties in [19].
Yager proposed the concept of fuzzy multiset in [20], and fuzzy planar graphs were developed along with their properties in [21] and [22]. Alshehri et al. [23] established some exciting proofs of Intuitionistic fuzzy planar graphs, and the concept of bipolar fuzzy planar graphs was described in [24]. Strong neutrosophic graphs were introduced in [25], and single-valued neutrosophic graphs were instituted by Broumi et al. [26]. Akram et al. introduced neutrosophic graphs and neutrosophic soft graphs along with their applications [27]. Single-valued neutrosophic hypergraphs and intuitionistic neutrosophic soft graphs were studied in [28,29]. The recent development of planar graphs in this area can be seen in [30–34]. The concept of the Pythagorean Neutrosophic graphs [35] was developed using the Pythagorean Neutrosophic set [36] and further into other graph-theoretical concepts in [37–40].
In this study, the graph-theoretical results are applied in the Pythagorean Neutrosophic fuzzy environment. The concept of planar graphs is more captivating because of their complexity. In designing circuits, circuits or lines are arranged so they do not intersect to avoid circuit problems, and planar graphs can be used to tackle this problem. Though all developments in fuzzy graph theory have advantages, the Pythagorean Neutrosophic graphs have their advantage with more fuzzified inputs. This research article elaborates on the abstraction of Pythagorean Neutrosophic Multi Graphs (PNMGs), Pythagorean Neutrosophic Planar Graphs (PNPGs), and Pythagorean Neutrosophic Dual Graphs (PNDGs). The potential applications of these graphs can be used to assess and design a variety of real-world challenges. Planarity is a crucial feature that is investigated in this work. A significant addition to the literature is the development of the Pythagorean neutrosophic planar and multigraphs with their characterizations.
The following is the order in which this article is organized: Section 2 deals with introducing the Pythagorean Neutrosophic Multi Graphs (PNMGs) and investigating their properties. Section 3 proposes the concept of the Pythagorean Neutrosophic Planar Graphs (PNPGs) along with the results and the investigation of its characteristics. An algorithm for decision-making using Pythagorean Neutrosophic fuzzy graphs has been proposed with the examination of a practical numerical illustration in Section 4 and the work is concluded in Section 5.
2 Pythagorean Neutrosophic Multigraphs
Definition 2.1. A Pythagorean Neutrosophic Multi Set
Definition 2.2.
1.
2.
3.
4.
5.
Definition 2.3. Let
Example 2.1. Let the multigraph be
Let
Definition 2.4. Let
Example 2.2. The vertices
Definition 2.5.
Definition 2.6.
1. G has order,
2. G has size,
3. The total degree of
Definition 2.7. Let
Example 2.3. Consider
Figure 1: Pythagorean neutrosophic multigraph
Definition 2.8. If each node of G has the same degree of
Definition 2.9. Let G be a
1. The edge
2. The edge
where
Definition 2.10. If the degree of
Example 2.4. Degree of the edges in
Theorem 2.1. Let
Proof. Consider,
Thus, for the
Thus, the
Theorem 2.2. Let
Proof. Consider
For every edge,
Thus, G is regular and edge regular
Definition 2.11. The strength of
An edge
Definition 2.12. Let
The planarity decreases when the number of points of intersection in
3 Pythagorean Neutrosophic Planar Graphs
The concept of the Pythagorean Neutrosophic planar graphs has been discussed.
Definition 3.1. Let G be a
Example 3.1. Take a multigraph
The
Figure 2: Pythagorean neutrosophic planar graph
The strength for the edges
Therefore
Theorem 3.1. Let G be a complete
Definition 3.2. A
Theorem 3.2. Let G be a
Proof. Let G be a
Thus,
(i.e.,)
This becomes a contradiction to the fact
Definition 3.3. Let G be a
Definition 3.4. A
Example 3.2. The
Figure 3: Faces in pythagorean neutrosophic planar graph
Clearly, the
Definition 3.5. Let G be a
Two common faces
Theorem 3.3. Let G be a
Theorem 3.4. Let
Definition 3.6. Let
The
Between
Example 3.3. Take a
Figure 4: Pythagorean neutrosophic dual graph
We symbolize the vertices of
The vertex set
There is one common edge
Definition 3.7. An isomorphism of two
1.
2.
Example 3.4. Consider two
Figure 5: Pythagorean neutrosophic planar graph
The
Thus, the
Definition 3.8. A weak isomorphism of two
1.
2.
Example 3.5. Consider two
Figure 6: Pythagorean neutrosophic planar graphs
A mapping y:
But
Thus
Definition 3.9. A co-weak isomorphism of two
1.
2.
Example 3.6. Take
Figure 7: Pythagorean neutrosophic planar graphs
A mapping
forall
4 Application in Decision Making Problem
The following algorithm is our proposed technique for multi-criteria decision making.
Step 1: Input the alternatives
Step 2: Aggregate all
Step 3: Calculate the aggregated value of each criteria
Step 4: Use the score function,
calculate the score matrix for the problem.
Step 5: Calculate the choice matrix by the function
Step 6: Now arrange the alternatives in an order and choose the maximum as the optimal decision.
In this competitive world, time is the most precious asset for everyone. In the given 24 h a day, saving time and using it for multiple duties, and chores is an important quality. Even though time management is in our hands, travelling from one point destination for the people who doesn’t drive is hard in recent times, cabs and travelling applications is one of the trending and useful facility in our cities. By the existing trends and techniques, the fastest and money-saving possibility is vital in day-to-day life. Consider the following scenario: a person wants to travel from a point to his destination and is given a set of mobile booking applications to choose a ride. Let there be these 5 cabs booking applications namely
Step 1: The alternatives are
Figure 8: Pythagorean neutrosophic fuzzy directed graph for M(k) (k = 1, 2, 3, 4, 5)
Step 2 and 3: Using PNFA operator, the aggregated values are calculated as follows:
Step 4: By using the score function, the score matrix is calculated in Table 6.
Step 5: Deriving the choice values of alternatives using the function, we get
Step 6: The order of ranking obtained for the problem is
Thus, the alternate with maximum value
The proposed model is compared with the decision-making method in [39] and is verified that the same ranking is obtained. Table 7 provides a comparison of both algorithms, showing the optimal alternative and results. Both algorithms provide the same optimum decision, as can be seen in the comparison table.
Graph theory is known for its vast applications in various fields most vitally in designing networking problems. In particular, numerous graph theoretical concepts have been introduced to model vagueness in networking problems. PN graphs, an extension of FGs, and a fusion of Pythagorean and Neutrosophic graphs have better flexibility to be applied in real-world problems. The article has initiated the concept of PN Multi Graph and PN Planar Graph employing the concept of PNGs. The concept of PN Dual Graph, isomorphism, weak and co-weak isomorphism has been explored for PN Planar Graphs and their results have been examined. An algorithm has been proposed using Pythagorean Neutrosophic fuzzy graphs with a numerical example for a real-life problem. The limitation of the set and study is that it is limited when it is compared with the newly proposed sets, but the advancements pave for the new concept of planar graphs in Pythagorean neutrosophic environment. The advantage of this proposed study is this set is more fuzzifying than the previous studies because of the set and their properties. This research can be extended further to investigate Interval- valued PNGs, bipolar PNGs, and their implementation in real-life situations.
Acknowledgement: The authors extend their appreciation to the Deanship of Scientific Research at King Khalid University for funding this work through the Large Group Research Project under grant number (R.G.P.2/181/44).
Funding Statement: This research was supported by Deanship of Scientific Research at King Khalid University.
Conflicts of Interest: The authors declare that they have no conflicts of interest to report regarding the present study.
References
1. L. A. Zadeh, “Fuzzy sets,” Information and Control, vol. 8, pp. 338–353, 1965. [Google Scholar]
2. K. T. Atanassov, “Intuitionistic fuzzy sets,” Fuzzy Sets and Systems, vol. 20, pp. 87–96, 1986. [Google Scholar]
3. F. Smarandache, “A unifying field in logics,” in Neutrosophy: Neutrosophic Probability, Set and Logic, Rehoboth: American Research Press, 1999. [Google Scholar]
4. R. R. Yager, “Pythagorean fuzzy subsets,” in Proc. of the Joint IFSA World Congress and NAFIPS Annual Meeting, Edmonton, AB, Canada, pp. 57–61, 2013. [Google Scholar]
5. A. Kaufmann, “Introduction a la Theorie des Sour-Ensembles Flous; Masson etCie 1,” Compléments et Nouvelles Applications, Masson, Paris, vol. 4, 1977. [Google Scholar]
6. L. A. Zadeh, “Similarity relations and fuzzy orderings,” Information Sciences, vol. 3, pp. 177–200, 2020. [Google Scholar]
7. A. Rosenfeld, “Fuzzy graphs,” In: L. A. Zadeh, K. S. Fu and M. Shimura (Eds.Fuzzy Sets and Their Applications to Cognitive and Decision Processes, pp. 77–95, Cambridge, MA, USA: Academic Press, 1975. [Google Scholar]
8. P. Bhattacharya, “Some remarks on fuzzy graphs,” Pattern Recognition Letters, vol. 5, pp. 297–302, 1987. [Google Scholar]
9. J. N. Mordeson and C. S. Peng, “Operations on fuzzy graphs,” Information Sciences, vol. 79, pp. 159–170, 1994. [Google Scholar]
10. A. Shannon and K. T. Atanassov, “A first step to a theory of intuitionistic fuzzy graphs,” In: D.Lakov, (Ed.Proc. of the Fuzzy Based Expert Systems, Sofia, Bulgaria, pp. 59–61, 1994. [Google Scholar]
11. A. Shannon and K. T. Atanassov, “Intuitionistic fuzzy graphs from α−,β−,(α,β)-levels,” Notes Intuitionistic Fuzzy Sets, vol. 1, pp. 32–35, 1995. [Google Scholar]
12. R. Parvathi, M. G. Karunambigai and K. T. Atanassov, “Operations on intuitionistic fuzzy graphs,” in Proc. of the IEEE Int. Conf. on Fuzzy Systems, Jeju Island, Korea, pp. 1396–1401, 2009. [Google Scholar]
13. M. Akram and W. A. Dudek, “Intuitionistic fuzzy hypergraphs with applications,” Information Sciences, vol. 218, pp. 182–193, 2013. [Google Scholar]
14. M. Akram and B. Davvaz, “Strong intuitionistic fuzzy graphs,” Filomat, vol. 26, pp. 177–196, 2012. [Google Scholar]
15. S. Naz, S. Ashraf and M. Akram, “A novel approach to decision-making with pythagorean fuzzy information,” Mathematics, vol. 6, pp. 1–28, 2018. [Google Scholar]
16. M. Akram and S. Naz, “Energy of pythagorean fuzzy graphs with applications,” Mathematics, vol. 6, pp. 136, 2018. [Google Scholar]
17. M. Akram, A. Habib, F. Ilyas and J. M. Dar, “Specific types of pythagorean fuzzy graphs and application to decision-making,” Mathematical and Computational Applications, vol. 23, pp. 42, 2018. [Google Scholar]
18. M. Akram, J. M. Dar and S. Naz, “Certain graphs under pythagorean fuzzy environment,” Complex & Intelligent Systems, vol. 5, pp. 127–144, 2019. [Google Scholar]
19. N. Abdul-Jabbar, J. H. Naoom and E. H. Ouda, “Fuzzy dual graphs,” Al-Nahrain Journal of Science, vol. 12, pp. 168–171, 2009. [Google Scholar]
20. R. R. Yager, “On the theory of bags,” International Journal of General Systems, vol. 13, pp. 23–37, 1986. [Google Scholar]
21. A. Pal, S. Samanta and M. Pal, “Concept of fuzzy planar graphs,” in Proc. of the Science and Information Conf., London, UK, vol. 14, pp. 557–563, 2013. [Google Scholar]
22. A. Pal, S. Samanta and M. Pal, “New concept of fuzzy planar graphs,” International Journal of Advanced Research in Artificial Intelligence, vol. 3, pp. 52–59, 2014. [Google Scholar]
23. N. Alshehri and M. Akram, “Intuitionistic fuzzy planar graphs,” Discrete Dynamics in Nature and Society, vol. 2014, pp. 1–9, 2014. [Google Scholar]
24. M. Akram, S. Samanta and M. Pal, “Application of bipolar fuzzy sets in planar graphs,” International Journal of Applied and Computational Mathematics, vol. 3, pp. 773–785, 2017. [Google Scholar]
25. R. Dhavaseelan, R. Vikramaprasad and V. Krishnaraj, “Certain types of neutrosophic graphs,” International Journal of Mathematical Sciences and Applications, vol. 5, pp. 333–339, 2015. [Google Scholar]
26. S. Broumi, M. Talea, A. Bakali and F. Smarandache, “Single-valued neutrosophic graphs,” Journal of New Theory, vol. 10, pp. 86–101, 2016. [Google Scholar]
27. M. Akram and S. Shahzadi, “Neutrosophic soft graphs with application,” Journal of Intelligent & Fuzzy Systems, vol. 32, pp. 841–858, 2017. [Google Scholar]
28. M. Akram and S. Shahzadi, “Representation of graphs using intuitionistic neutrosophic soft sets,” Journal of Mathematical Analysis, vol. 7, pp. 1–23, 2016. [Google Scholar]
29. M. Akram, S. Shahzadi and A. B. Saeid, “Single-valued neutrosophic hypergraphs,” TWMS Journal of Applied and Engineering Mathematics, vol. 8, pp. 122–135, 2018. [Google Scholar]
30. G. Muhiuddin, S. Hameed, A. Rasheed and U. Ahmad, “Cubic planar graph and its application to road network,” Mathematical Problems in Engineering, vol. 2022, pp. 1–12, 2022. [Google Scholar]
31. T. Mahapatra, S. Sahoo, G. Ghorai and M. Pal, “Interval valued m-polar fuzzy planar graph and its application,” Artificial Intelligence Review, vol. 54, no. 3, pp. 1649–1675, 2021. [Google Scholar]
32. R. Mahapatra, S. Samanta and M. Pal, “Generalized neutrosophic planar graphs and its application,” Journal of Applied Mathematics and Computing, vol. 65, no. 1, pp. 693–712, 2021. [Google Scholar]
33. G. Sur and Ö. Erkan, “Surface quality optimization of CFRP plates drilled with standard and step drill bits using TAGUCHI, TOPSIS and AHP method,” Engineering Computations, vol. 38, no. 5, pp. 2163–2187, 2021. [Google Scholar]
34. Ö. Erkan, D. Mustafa, I. Birahan and N. T. Ibrahim, “Selection of optimal machining conditions for the composite materials by using taguchi and GONNs,” Measurement, vol. 48, pp. 306–313, 2014. [Google Scholar]
35. D. Ajay and P. Chellamani, “Pythagorean neutrosophic fuzzy graphs,” International Journal of Neutrosophic Science, vol. 11, pp. 108–114, 2020. [Google Scholar]
36. R. Jansi, K. Mohana and F. Smarandache, “Correlation measure for pythagorean neutrosophic fuzzy sets with T and F as dependent neutrosophic components,” Neutrosophic Sets and Systems, vol. 30, pp. 1–16, 2019. [Google Scholar]
37. D. Ajay, P. Chellamani, G. Rajchakit, N. Boonsatit and P. Hammachukiattikul, “Regularity of pythagorean neutrosophic graphs with an illustration in MCDM,” AIMS Mathematics, vol. 7, no. 5, pp. 9424–9442, 2022. [Google Scholar]
38. D. Ajay, S. John Borg and P. Chellamani, “Domination in pythagorean neutrosophic graphs with an application in fuzzy intelligent decision making,” in Int. Conf. on Intelligent and Fuzzy Systems, Cham,Turkey, Springer, pp. 667–675, 2022. [Google Scholar]
39. P. Chellamani and D. Ajay, “Pythagorean neutrosophic dombi fuzzy graphs with an application to MCDM,” Neutrosophic Sets and Systems, vol. 47, pp. 411–431, 2021. [Google Scholar]
40. D. Ajay and P. Chellamani, “Operations on pythagorean neutrosophic graphs,” AIP Conference Proceedings, vol. 2516, no. 1, pp. 200028, 2022. [Google Scholar]
Cite This Article
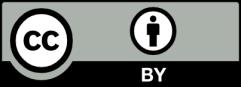