Open Access
ARTICLE
Optimizing Decision-Making of A Smart Prosumer Microgrid Using Simulation
1 College of Engineering and Technology, American University of the Middle East, Egaila 54200, Kuwait
2 Operations and IT, ICFAI Business School, Hyderabad, 501203, India
* Corresponding Author: Oussama Accouche. Email:
(This article belongs to the Special Issue: Energy Efficiency and Energy Consumption of Sensor Network for Applications, Including the Various Methods of Energy Storage)
Computers, Materials & Continua 2023, 76(1), 151-173. https://doi.org/10.32604/cmc.2023.038648
Received 22 December 2022; Accepted 12 April 2023; Issue published 08 June 2023
Abstract
Distributed renewable energy sources offer significant alternatives for Qatar and the Arab Gulf region’s future fuel supply and demand. Microgrids are essential for providing dependable power in difficult-to-reach areas while incorporating significant amounts of renewable energy sources. In energy-efficient data centers, distributed generation can be used to meet the facility’s overall power needs. This study primarily focuses on the best energy management practices for a smart microgrid in Qatar while taking demand-side load management into account. This article looked into a university microgrid in Qatar that primarily aimed to get all of its energy from the grid. While diesel generators are categorized as a dispatchable distributed generation with energy storage added to handle solar radiation from the sun and high grid power operating costs in the suggested scenario, wind turbines and solar Photovoltaic (PV) are classified as non-dispatchable distributed generators. The resulting linear math issues are assessed and displayed in MATLAB optimization software using a mixed-integer linear programming (MILP) strategy. According to the simulation results, the suggested energy management strategy reduced the university microgrid’s grid power costs by 38.8%, making it an affordable solution which is somehow greater than the prior case scenario’s 23% savings. The installed solar system capacity’s effects on the economy, society, and finances were also assessed, and it became clear that the best option for the smart microgrid was determined that would be 325 kW of solar PV, 25 kW of wind turbine, and 600 kW of diesel generators, respectively. Given the current situation, university administrators are urged to participate in distributed generators and adopt cutting-edge designs for energy storage technologies due to the significant environmental and financial benefits.Keywords
Energy systems have been experiencing many challenges including complex network overloading, greenhouse gas emissions, insignificant scheduling of energy generation, and rapidly increasing electricity generation costs. The traditional power system is unable to deal with these types of issues and challenges efficiently, but smart microgrid systems with a variety of distributed generation (DGs) combined with modern distribution systems and storage technologies can deal with these issues by implementing demand-response solutions [1]. The smart grid solutions can be assimilated with other renewable energy resources to improve the efficiency and performance of the microgrid.
Smart microgrids have efficient and inexpensive renewable energy solutions due to their economic, environmental, and technical benefits such as energy security, electricity bill savings, emission reduction, and resiliency of a microgrid [2]. Smart microgrids are small-scale, distributed, self-contained energy systems with multiple renewable generation sources and distributed loads, and it operates independently for energy security, electricity bill savings, emission reduction, and resiliency of a microgrid [3]. These emerging microgrid systems improve the reliability, resilience, sustainability, and security of the overall electric power network. Smart Microgrids are one of the promising approaches to providing a wide range of services including power quality, reliability, and cost reduction in future smart electricity networks [4,5].
A microgrid system is a promising solution to overcome these energy challenges. Due to this, Qatar’s energy sector [6] depends exclusively on fossil fuels for electricity generation. A small portion of the total energy generated is used for desalination, with public outages in the summer to cope with the increased demand for power and water generation. Fossil fuels supply over 95% of energy consumption, mainly crude oil and natural gas [7]. Qatar does not have any coal or hydrocarbon sources for traditional power generation. The country has a small refinery capacity which covers only 20% of its domestic consumption. About 34 percent of worldwide carbon emissions come from coal, oil, and natural gas. The usage of smart grids allows us to cope with challenges like global climate change, energy distribution control and efficiency optimization [8]. Due to the variable nature of loads, Qatar universities are among the large-load consumption electricity which comes in the class of mixed loads consumers. These kinds of buildings have the option of prosuming surplus electricity from the grid owing to the accessibility of site power generation [9]. The main motivation of this study is to assure active participation in microgrid operations and to help with an optimal dispatch to achieve demand at the least possible cost, energy management systems are utilized alongside traditional resources [10]. The study focused on the strengthening of energy management systems for an associated battery storage facility and onsite DGs in a smart microgrid. The proposed Energy Management System (EMS) approach may safely and efficiently manage the bidirectional energy flow among the utility network and the microgrid to schedule the battery charging cycles for the energy storage system (ESS) in a way that would reduce energy costs. For a thorough study, the actual academic load at this university was considered. The suggested university microgrid presently includes wind energy, a standby diesel generator as an outer source, and a state grid network connected with a regional electricity market. The Qatar Electricity and Water Company (QEWC) is responsible for the electricity supply, so grid electricity is supplied from their power system. In this study, the eco-friendly environment and economic effects of PV integrated with energy storage were discussed along with the generation of energy using various renewable energy sources. However, this study also focuses on the benefits of integrating photovoltaic (PV) systems with energy storage. By combining PV with energy storage, it is possible to increase the overall efficiency and reliability of renewable energy systems. Additionally, the study might explore the generation of energy using various renewable energy sources, such as solar, wind, or hydropower, to provide a comprehensive understanding of renewable energy systems and their potential benefits. The ultimate goal of the study might be to provide recommendations for promoting the adoption of renewable energy technologies and to help mitigate the effects of climate change.
In the literature, several studies have been undertaken to evaluate the optimal energy systems at various locations for generating energy using various techno-economic approaches and methods. Only a couple of the studies that looked at calculating the Levelized Cost of Electricity (LCOE) for the demand side management are mentioned below.
Xu et al. [11] proposed an integrated module with the Demand Side Management (DSM) which aims to consider the non-changeable loads for the prosumer microgrid. In this proposed module, the microgrid consists of DSM that calculates the operational cost of both the controllable loads and the un-controllable loads. A modified Genetic Algorithm (GA) is developed which optimizes the proposed module. Results show that integration of the micro-market with demand-side management minimizes the electricity cost for the university microgrid. While Nigam [12] examined a different smart microgrid and compared it to the suggested microgrid solution with the existing university smart grid to find the most suitable and efficient microgrid solution. This study focuses on the implementation of a smart microgrid at Illinois University. This paper uses a mixed-integer linear program (MILP) approach that operates the smart microgrid according to the economical, reliable, and sustainable environment. Results show that methodologies implemented in the Illinois university microgrid were to maintain reliability, achieve economic benefit, and fulfil the power supply and demand-side requirements. Likewise, they can import energy from the utility grid if DGs and energy storage systems might be unable to encounter the load conditions [13]. Integrating these microgrids into power networks, operational energy costs are reduced, with an emphasis on the advantages of the distribution system.
However, another author presented a solar PV-based prosumer microgrid solution in [14] to replace hydrocarbon-based electricity generation with a PV-based generation at Purdue University, Indiana. It consists of a solar PV grid with 3-lead-acid batteries. The main objective is to build and install a solar PV microgrid that aims to protect the environment from sulfur, Nitrous oxide (NO), and carbon released from hydrocarbon electricity generation. With the installation of solar microgrids, toxic Greenhouse gas (GHG) emissions are reduced, energy consumption costs are also reduced, power quality is increased, and voltage regulation is improved as well. While Ahsan el at. [15] modelled a Battery Storage System dynamic approach to calculate the operating costs of a smart microgrid and to provide the economic dispatch power/unit energy of the system. This proposed model also examines the voltage dependency of the batteries on the state of charge (SOC) and currents. Using this, it carried out the efficiency conversion analysis on SOC and power output. Results conclude that using this modelled approach rather than LCOE, lowered the microgrid operational cost up to 12% which is the optimal solution.
A solar-diesel hybrid university prosumer microgrid is modelled by Uchechukwu et al. in [16] for the Nnamdi Azikiwe University, Nigeria. The NASA sun irradiance data was anticipated for the entire year by the postgraduate building’s sub-grid. It estimated the load demand for the university building in HOMER software. The installed capacity for the post-graduate building was 84.15 kW. Simulation results delivered the component that was installed for the microgrid. The sub-grid consisted of a Solar PV system (950 pieces in kW/total 300 W), Lithium-Ion Batteries (60 pieces in kWh), a diesel generator (100 kW size), and a converter (145 kW size), etc. Results show that the net present value (NPV) and LCOE were calculated at 1,738,994$ and 0.264$. It somehow lacks in calculating the financial analysis of the system to further optimize the system.
On the other hand, another author presented a hybrid system in [17] with an optimal solution for the smart microgrid of Aligarh University, India. The given microgrid system comprised of the solar PV system, BESS system, and diesel generators (DGs) can serve as a backup to support the microgrid load. The study utilized the Homer Pro software which is used as a microgrid optimization tool. Results analyzed that the system’s investment cost with PV and BESS is 18.2 million USD, or 64.2% higher than that of the existing system (11.18 million USD). Therefore, the cost of investment for a PV system and Grid system is ₹494.92 INR in millions, which is 57.2% cheaper as compared to the cost of the existing system, which is an optimal option.
However, Simmhan et al. [18] demonstrated an architecture of data integration, a machine-learning demand response (DR) based forecasting model and the calculation of load demand by real-time complex event processing (CEP) technique. This paper studied the use of algorithms in power system protection and coordination. The authors used the data integration method, which integrates traditional and new methods of energy management to determine the optimal load scheduling for a system with limited resources. It is situated in an electrical department, at the University of Southern California (USC). This data integration portal will tell the consumers about the temperature, and power consumption in the building USC. Results will display on the portal as well as display the power consumption, load demand response, and power flow in the university building. However, Table 1, analyzed the LCOE that was calculated by considering multiple microgrid configurations at different locations. Another effective method is discussed in [19] where the author proposed a method to effectively design a power system for the University of Beirut (AUB) campus which was affected by a power system outage and dependence on distributed generators. This approach was able to manage the power flow during active and passive hours of the day. Results show that it reduces operation cost for the distributed generation (DG) by 53.7% (for 1st Year) to 3.4% (after 10 years) in operation mode. This proposed system reduces the cost of electricity (COE) for the whole system by 0.137$/kWh-to-0.088$/kWh (1st Year) and 0.144$/kWh-to-0.1$/kWh (for the 10th year) with a net positive cash flow at 6th year. Many of the literature studies discussed above were focused on demand-side management and energy management of smart microgrids and implementing an optimal solution to improve their power system by adopting an effective solution for their microgrid systems. Several studies focused exclusively upon the economic feasibility of PV combined with the ESS for smart microgrids, while others calculated the cost reductions with the inclusion of their PV integration and an optimally scheduled ESS.
The important objectives and novelties of this research work are as follows:
a) An intelligent and efficient energy management system proposed to enhance the schedule of the onsite solar PV, diesel generators, energy storage systems, and grid energy using MILP and time of use (TOU) based real-time responses to increasing renewable energy resources (RER) consumption while reducing operational power costs and system peak-hours as well as load consumption reductions.
b) With stochastic solar PV generation, which was used in a smart prosumer microgrid, degradation battery cost is also considered.
c) An economical study is also carried out here to examine the techno-economic impact on multiple attached RER resources and to find an optimal solution for the Qatar university smart microgrid, which is also centred on the net-metering TOU-based environments.
The remainder of this article is arranged as follows. Section 2 describes the model of the proposed system and solution approach. The entire problem formulation is presented in Section 3. Next,Section 4 includes a series of case studies with simulation solutions. Lastly, Section 5 offers the author’s final remarks and some future research proposals.
3.1 Suggested Conceptual Framework
The prosumer microgrid is made up of several kinds of energy storage facilities, loads, and some generation supplies (Diesel generator and PV). An energy supplier from a grid has a facility known as a net-metering facility for the smart prosumer, who may use it to exchange any excess energy with the grid. Fig. 1 depicts the suggested model’s conceptual model, which includes the utility grid, energy management structure, and a prosumer microgrid. The proposed EMS is focused and deployed at the prosumer end that receives load demand and pricing data, weather forecasts, the baseline condition of the energy storage, and its relevant input parameters and finds the best way to satisfy the demand of the required load using existing resources while staying within operational and design restrictions.
Figure 1: Conceptual model of the proposed smart prosumer microgrid (SPM)
The controlling scheduler uses this optimum solution to allocate existing resources. The suggested EMS also has the capability of storing several essential parameters, which might be used for a variety of purposes in the future. Energy exchange data, pricing data, and smart prosumers load data are stored in market databases (DB), Real-time DB, and smart prosumer DBs. The given model is presented in the following subsections.
The proposed system’s architectural model of the University of Qatar is constructed as a mixed-integer linear optimal control problem to minimize the prosumer microgrid operational expenses while taking into consideration the lifespan of the energy storage system. The proposed model has many additional components with certain limitations and restrictions, which are mentioned in detail in the following sections.
The proposed model tries to reduce the operational costs (J) of the given prosumer microgrid, which comprises an interchange of energy costs ‘E’, the wind turbine WT’ costs, ‘DG’ generating units cost, and the energy storage deterioration (2)–(5). The total expenses for calculating the energy resources are given by Eq. (1). As shown in Eqs. (4)–(6), several factors, such as the no. of cycle used, assets investments, and entire system capacity, affects the battery life, whilst charging/discharging efficiency and power are expressed as
Here, some of the factors are costs expressed as
Figure 2: Proposed architectural design of EMS model
The load equality constraints symbolize the demand-side equilibrium. The expression (7) must be met and fulfilled to attain equilibrium. However, the prosumer load profile is denoted as
ESS does not need to be a neglected factor in power management, since it supports to contribute the system’s power in any tragic occasion such as power system failure. Although this ESS is normally hard to charge or discharge quickly, their energy limitation has indeed been incorporated in the limitations (8)–(9). Whereas, up to (12) the battery charging state “BSOCt” relies on the prior state represented as BSOC(t−1), which is combined with an Eq. (13) at any time t. The extreme limitations of the battery charging status are correspondingly denoted by BSOCmin and BSOCmax and in an equation to avoid ESS overcharging and discharging issues (14). A battery state of charge (BSOCt) at t interval (day’s end) is equal with the primary state of the battery (BSOCo) that occurs at the beginning of a day is shown in Eq. (15).
To efficiently plan the energy contribution in an energy management system, the output power of the storage system
The energy storage’s output gradient is as follows:
3.6 Diesel Generator with Grid Limitations
As utilities rely on their components of load demand, they must negotiate peak load contracts through consumers regularly. Some demand that goes beyond the scope of such a contract will consequence in penalties or termination of the electricity connection. Diesel generators, likewise, cannot manage loads more than their design capacity. For the generating units and the grid-connected PV, phrases are used to address the availability of power restrictions, as shown in the Eqs. (17)–(18).
3.7 Grid and Smart Prosumer Energy Exchange
The exchanged net energy with a utility grid in a day is expressed as
3.8 Probabilistic Solar PV Modelling
Solar production patterns are quite unbalanced and not regular which is dependent on an environment having high irradiance output [34]. The entire year’s data will be examined under random settings. This article makes use of an earlier constructed solar irradiance simulation [35–37]. Additionally, the curve parameters of the probability distribution function are computed. The Latin Hypercube (LHS) global sampling method may generate 365 scenarios in 24 h [38–40]. The fast-forwarding approach is normally used to reduce approximately 40 randomized random generated scenarios [41,42] for the goal of reducing the calculation or computational burden, as indicated in [42].
In Eq. (20), the normally distributed function or a Gaussian function [43] is utilized to develop an uncertain model for solar irradiation. While
Figure 3: Mean value of solar irradiance and standard deviation patterns
3.9 Grid and WT Energy Exchange
Eq. (22) expresses the wind energy generation
The minimal cut-in velocity needed for the wind turbine to create power is stated as
The Levelized cost of energy is calculated in many aspects to undertake an efficient and equitable economic study of the systems. It is determined by dividing the entire system installation cost in dollars by the amount of energy produced (kWh). The LCOE of a specific energy storage system is mainly defined as dollars per kWh of electricity. It considers all related expenses, like installation, operating, or maintenance costs, as well as capital expenditures. It may also be thought of as the lowest price on which electrical energy generation must be sold to keep the budget in range to improve net profit throughout the lifespan of the electricity generating with an energy storage component [39]. The LCOE calculation may be written as follows:
The general solution methodology of the MILP approach is presented as follows (see Fig. 4): Because the proposed system objective function and the system restrictions happen essentially with a linear model by a large number of additional integers variable, the MILP approach is used to handle problems like linear optimization [40]. This MILP methodology is generally used as a global optimization method for solving a variety of optimization issues in branding, planning, and optimum scheduling.
Figure 4: Proposed system flowchart
Furthermore, it is evaluated with several metaheuristic approaches that provide inferior results, while MILP produces the best ideal outcomes. Consequently, the MILP approach is widely employed in EMS optimizations [41]. The following is the MILP’s general structure in Eqs. (24) and (25):
This proposed concept is being utilized for the smart prosumer microgrid in the Qatar region as described in Section 3. There are a couple of dormitories, ten college departments, and many faculties in the university. Currently, the university relies on a 2 MW grid connection to meet its needs. Based on a quick review of the available space on the university rooftop, the capacity of the PV system is estimated at 4 MW.
Due to regulatory restrictions and financial limits, we are only able to build 4 MW PV due to the Qatar Electricity and Water Company (QEWC) of Qatar allowing a limited amount of energy trade among utility networks. In our scenario, as contrasted to the [42], a local 325 kW PV System is examined for a full economical and technical study. Other implications concentrated on the utilization of the accessible portable generator as a standby in an event of an electrical grid failure or breakdown.
Moreover, the power grid is intended to have an excellent net-metering system which permits energy export to regulate equal to 1 MW in compensating for the microgrid energy usage costs. Due to an overall hostel load, academic block load, administrative offices load, and a load of housing colonies on campus, the university load varies constantly.
As it has 320 bright days per year and 9–12 h of sunshine each day, solar PV is a practical and workable method to alleviate energy difficulties in Qatar. Qatar produces 5.1 MWh/m2 of solar power each day from a 1 MW PV power plant, as per the [43] research. Thus, in this research, an optimal solution is created by developing an efficient system for the prosumer microgrid. A BESS network has been examined for our method, and a lithium-ion battery offers a couple of benefits extended lifespan, better operation, high power density, high reliability, and considerable self-discharge [44].
In different case studies, an optimum microgrid planning and approach is shown for Qatar’s hot season as it remains the same all year long. Variations in load conditions are usually normal over the year and for convenience of study, energy patterns are taken to be the same for each season. In Qatar, the highest electricity consumption cycles are Jan and Aug, as they are the system peak periods [45]. Peak load profiles for some months like (Jan and Aug) are used for economic and financial evaluation, with worst-case scenarios considered. The best potential outcome for cost reduction is achieved by selecting the worst-case scenario. To calculate the economic advantage, PV energy can be supplied to the grid to maximize the benefits.
When an institution has a working day, the administrative loads and loads of academic blocks are intended to be higher, whereas the peak energy needs in the dormitories and resident societies are examined until the middle of the night. Table 2 shows several metrics that are related to a system, while Table 3 shows a TOU scheme’s electricity pricing data. Specifically, the daily solar data utilized is taken from [46] and data properties are demonstrated and evaluated with the help of probability distribution functions indicated in the Eq. (19). Our main goal for PDF is normally to generate the daily patterns of solar on regular basis [47,48], whereas the previously present irradiance flow predicts the PV generating output power utilizing Eq. (20).
Many case scenarios are examined here in our study case, in which energy exchange analysis and energy usage according to our load are expressed using price-based metrics. To better know the power requirement for all seasons, many scenarios have been developed. Overall load patterns of load pattern are considered also, which shows the load pattern behaviour of the campus microgrid in such a way, their residential load pattern behavior, their academic load, and general load behavior are illustrated in Figs. 5 and 6.
Figure 5: Overall load pattern behaviour of the microgrid
Figure 6: Case scenario (b): Energy exchange with grid and PV
Case Scenario (a): In our first case scenario, the campus’s electricity needs are met entirely by the grid. The campus does not have solar, wind, ESS, or a diesel generator. The operating cost of electricity is determined to be $1630.8 using the time of use (TOU) rate. In this case scenario, LCOE is computed as 0.113$/kWh. But the findings revealed that in the first case, the day-to-day operating costs of the grid’s energy are exceptionally expensive, and this case scenario is utilized further as a study to evaluate and investigate other case scenarios.
Case Scenario (b): In the 2nd case scenario, solar is associated with an institutional smart microgrid, allowing it to both export surplus power towards the utility grid and supply the demand. Solar PV yields 8584.3 kWh, demonstrating its efficiency. The LCOE is calculated in this case by 0.052$/kWh. The net cost calculated for the electricity/day is lowered by 46.08%, to $748.5 from the base case scenario.
Case Scenario (c): The ESS is combined with the Solar and grid connection in this assumed scenario. The suggested technique is employed to estimate the net electricity cost, which is $712.9, and to scheme the charging time patterns efficiently while considering all related expenses. With the use of TOU-based tariffs and the BESS optimum scheduling process, the LCOE was computed as $0.050/kWh, also depicted in Table 3. However, the modest rise in LCOE calculation is due to BESS cost, which remains here. When compared to baseline scenario 1(a), the overall cost of power is reduced by 43.89%, which is displayed in Fig. 7.
Figure 7: Case scenario (c): Scheduled energy trade with grid, PV, and storage power
Case Scenario (d): The smart prosumer microgrid in the fourth scenario combines a backup generator with the BESS network and Solar PV in reducing the grid peak power demand during peak hours (6:30 PM to 12:00 PM). The grid can only import power equal to 50 kW, which has limitations for the connected grid, while the power output of this diesel generator is controlled to 600 kW in the peak hours, which is displayed in the Fig. 8.
Figure 8: Case scenario (d): Scheduled energy trade with PV, grid, storage power, and diesel generator
An overall electricity cost is calculated to be $590.5 each day using BESS optimum scheduling. When it is contrasted with base scenario (a), the LCOE determined in this example is 0.046$/kWh, which is 41.02% better than the previous case scenario and operates with the 8.119829 s computational time.
Case Scenario (e): Scenario 1, (e): In this case scenario, a wind turbine is connected with energy storage, rooftop solar, a diesel engine, and the utility grid. In February and March, wind speed and direction are (3–5) times greater than in the other months. The smart prosumer microgrid chose a WT with a power rating of 25 kW after examining many variables, including hub height of 36.6 m, rated wind conditions of 14 m/s, wind cut-in and cut-out velocities are 3.5 m/s and 25 m/s, respectively as 25 m/s is an optimal option. The results are presented in Fig. 9.
Figure 9: Proposed case scenario (e): Energy trade with PV, utility grid, storage power, diesel generator, and wind turbine system
The LCOE for this scenario was determined to be 0.044$/kWh, which is 38.99%. The prosumer net cost electricity in this case yields 8743.5 kWh, demonstrating the best optimally scheduled efficiency. The LCOE calculated intended for rooftop solar remains 0.044$/kWh. The incorporation of the WT network with the energy system, rooftop solar, diesel engine, and grid-connected resulted in a 568.8$/day reduction in the electricity cost-benefit for the Qatar smart prosumer microgrid.
The results of existing approaches to the proposed model are summarized in Table 4.
In Table 5, * this does not include the expenses of ESS, Solar PV, wind system, or diesel generator, which are additional system costs. To compute this cost, the LCOE for each scenario is employed. The LCOE of photovoltaics is predicted to be 0.048 dollars per kWh [42]. The installation costs of diesel generators and energy storage are partially offset by contributing of 0.155$/kWh and 0.065$/kWh, respectively, to the given model, which also included operating and repair costs of certain energy storage systems and diesel generators in various cases. If the prosumer is mentioned inside the carbon development framework, then the prosumer will receive a receivable carbon credit (CC) [42].
4.3 Carbon Emission Effects on the Energy Costs
The impacts of different solar PV sizes of a smart microgrid to buy electricity from the utility grid and emissions of carbon dioxide for a day are investigated. When rooftop solar is incorporated doubled, the GHG emission is also reduced by 2 times, in addition to cost savings. The graph in Fig. 10 has some colourful bars that demonstrate the multiple kinds of PV incorporation according to the proposed case scenarios and their impacts on electricity cost obtained from the grid. In all of the above cases, these calculated values can analyze the differences between their operating energy costs.
Figure 10: Entire electricity net cost/day with an analysis of different case scenarios
4.4 Economic Effects on the Given Parameters
The research also shows the power ratings of system components, as well as their capital costs, service & maintenance expenses, replacement costs, return on investment, increased efficiency, and lifespan.
Rooftop Solar, BESS, converters, wind energy systems, DGs, utility grid, and other additional devices throughout the distributed generation is one of the target aspects. The impacts of various parts of the system which are presently in use are investigated. With various components joined in the system, its NPV cost is calculated. If the system included components rated at 1000 kW rooftop Solar, 550 kW diesel generator, 3500 kW capacity of the energy system, and 1400 kW converters, the net current cost was estimated to be around 12.8 million dollars as illustrated in Fig. 11.
Figure 11: NPV calculation in different case scenarios
Therefore, an ideal smart and effective solution for our microgrid has been determined as an ideal solution for the Qatar Microgrid. As, it is determined that an optimal solution for our system would be 325 kW of solar PV, 25 kW of the wind turbine, and 600 kW of diesel generators, respectively. By estimating a daily power cost of 568.8$/kWh as an ideal solution for the smart microgrid, an optimum system is implemented.
However, when compared to the other case scenarios, the savings evaluated at 38.99%, which is somehow greater than the prior case scenario’s 23% savings. It is determined that the optimum smart system with the addition of a WT, PV for the system results in larger savings, making it the best viable option for the smart prosumer microgrid.
In this study, a smart prosumer microgrid’s management and scheduling of energy storage systems as well as the effects of rooftop solar are examined to lower the microgrid’s operational costs for electricity by using predictive load data. It is suggested that the proposed smart system consider PV, BESS, and generator as DGs which focused on a variety of situations, analyzing numerous effects in each. In MATLAB, the optimum scheduling issue was evaluated and treated as a MILP problem with battery lifetime considerations. The time-of-use tariff pricing was investigated, and ESS was used with a demand response system that can be recharged smartly at efficient times aiming in achieving a cost-cutting goal without compromising the system’s longevity. In the absence of an ESS or diesel generator, the utility provider provides all the microgrid necessary energy, resulting in higher operational expenses. When Solar, WT, energy storage, and generators are combined with the prosumer microgrid, the average saving calculated is 38.99%. The influence of various sizes of Photovoltaic systems on the ecosystem investigated and determined which resulted as an ideal solution for a smart microgrid would be a 325 kW of solar PV, 25 kW of the wind turbine, and 600 kW of diesel generators, respectively. In addition to microgrids, there are several other important solutions that can be incorporated further to ensure energy security for smart prosumer microgrids, such as reducing dependence on fossil fuels, making the microgrid less dependent on the traditional grid, upgrading to more efficient smart grid appliances, optimizing heating and cooling systems can reduce energy demand, using demand response programs incentivize consumers to reduce their energy use during periods of high demand, using advanced control systems to operate more efficiently. By incorporating these solutions, smart prosumer microgrids can help ensure energy security, reduce dependence on fossil fuels, and promote sustainability.
Funding Statement: The authors received no specific funding for this study.
Author Contributions: Conceptualization, O.A.; methodology, O.A. and R.G.; software, R.G.; data curation, O.A.; writing—original draft preparation, O.A.; writing—review and editing, O.A.; supervision, R.G.
Conflicts of Interest: The authors declare that they have no conflicts of interest to report regarding the present study.
References
1. H. S. Shreenidhi and N. S. Ramaiah, “A two-stage deep convolutional model for demand response energy management system in IoT-enabled smart grid,” Sustainable Energy, Grids and Networks, vol. 30, pp. 100630, 2022. [Google Scholar]
2. E. Tebekaemi and D. Wijesekera, “Secure overlay communication and control model for decentralized autonomous control of smart micro-grids,” Sustainable Energy, Grids and Networks, vol. 18, pp. 100222, 2019. [Google Scholar]
3. L. Jacob Varghese, K. Dhayalini, S. Sira Jacob, I. Ali, A. Abdelmaboud et al., “Optimal load forecasting model for peer-to-peer energy trading in smart grids,” Computers, Materials & Continua, vol. 70, no. 1, pp. 1053–1067, 2022. [Google Scholar]
4. N. A. Bourahlaa, M. Benghanem and H. Bouzeboudja, “Conception and analysis of a photovoltaic microgrid in the USTO campus,” in Int. Conf. on Electrical Sciences and Technologies in Maghreb, Algiers, Algeria, pp. 1–6, 2019. https://doi.org/10.1109/CISTEM.2018.8613489 [Google Scholar] [CrossRef]
5. M. H. Alsharif, K. Raju, A. Jahid, M. A. Albreem, P. Uthansakulvet et al., “Optimal cost-aware paradigm for off-grid green cellular networks in Oman,” Computers, Materials & Continua, vol. 68, no. 2, pp. 2665–2680, 2021. [Google Scholar]
6. A. Al Wahedi and Y. Bicer, “A case study in Qatar for optimal energy management of an autonomous electric vehicle fast charging station with multiple renewable energy and storage systems,” Energies, vol. 13, no. 19, pp. 5095, 2020. [Google Scholar]
7. Y. Pezhmani, M. Z. Oskouei, N. Rezaei and H. Mehrjerdi, “A centralized stochastic optimal dispatching strategy of networked multi-carrier microgrids considering transactive energy and integrated demand response: Application to water–energy nexus,” Sustainable Energy, Grids and Networks, vol. 31, pp. 100751, 2022. [Google Scholar]
8. H. Javed, M. Irfan, M. Shehzad, H. Abdul Muqeet, J. Akhter et al., “Recent trends, challenges, and future aspects of P2P energy trading platforms in electrical-based networks considering blockchain technology: A roadmap toward environmental sustainability,” Frontiers in Energy Research, vol. 10, no. March, pp. 1–20, 2022. [Google Scholar]
9. E. C. Okonkwo, I. Wole-Osho, O. Bamisile, M. Abid and T. Al-Ansari, “Grid integration of renewable energy in Qatar: Potentials and limitations,” Energy, vol. 235, pp. 121310, 2021. [Google Scholar]
10. M. S. Shahzad, H. Javed, H. A. Muqeet, H. A. Khan, N. Ahmed et al., “An optimal scheduling and planning of campus microgrid based on demand response and battery lifetime,” Pakistan Journal of Engineering & Technology, vol. 4, no. 3, pp. 8–17, 2021. Available at: https://hpej.net/journals/pakjet/article/view/1188 [Google Scholar]
11. F. Xu, W. Wu, F. Zhao, Y. Zhou, Y. Wang et al., “A micro-market module design for university demand-side management using self-crossover genetic algorithms,” Applied Energy, vol. 252, no. May, pp. 113456, 2019. https://doi.org/10.1016/j.apenergy.2019.113456 [Google Scholar] [CrossRef]
12. S. Nigam, “Quantification of benefits of the campus grid,” Ph.D. Dissertation, University of Illinios,United States, 2017. [Google Scholar]
13. E. Hossain, I. Khan, F. Un-Noor, S. S. Sikander and M. S. H. Sunny, “Application of big data and machine learning in smart grid, and associated security concerns: A review,” IEEE Access, vol. 7, no. c, pp. 13960–13988, 2019. https://doi.org/10.1109/ACCESS.2019.2894819 [Google Scholar] [CrossRef]
14. M. S. Wilfing, “Integration of solar microgrids,” Ph.D. Dissertation, Purdue University, United States, 2019. [Google Scholar]
15. A. Ahsan, Q. Zhao, A. M. Khambadkone and M. H. Chia, “Dynamic battery operational cost modeling for energy dispatch,” in ECCE 2016-IEEE Energy Conversion Congress and Exposition (ECCE), Milwaukee, USA, pp. 1–5, 2016. https://doi.org/10.1109/ECCE.2016.7855055 [Google Scholar] [CrossRef]
16. A. Uchechukwu, A. C. O. Azubogu and H. C. Inyiama, “Modeling and simulation of campus solar-diesel hybrid microgrid using HOMER grid (SPGS Nnamdi Azikiwe university awka campus as a case study),” The International Journal of Engineering and Science, vol. 8, pp. 97–108, 2019. https://doi.org/10.9790/1813-08120297108 [Google Scholar] [CrossRef]
17. F. Ahmad and M. S. Alam, “Optimal sizing and analysis of solar PV, wind, and energy storage hybrid system for campus microgrid,” Smart Science, vol. 6, no. 2, pp. 150–157, 2018. https://doi.org/10.1080/23080477.2017.1417005 [Google Scholar] [CrossRef]
18. Y. Simmhan, V. Prasanna, S. Aman, S. Natarajan, W. Yin et al., “Demo abstract: Toward data-driven demand-response optmisation in a campus microgrid,” in Proc. of the Third ACM Workshop on Embedded Sensing Systems for Energy-Efficiency in Buildings, Washington, USA, pp. 41–42, 2011. [Google Scholar]
19. E. Muh and F. Tabet, “Comparative analysis of hybrid renewable energy systems for off-grid applications in Southern Cameroons,” Renewable Energy, vol. 135, pp. 41–54, 2019. [Google Scholar]
20. E. M. Nfah and J. M. Ngundam, “Feasibility of pico-hydro and photovoltaic hybrid power systems for remote villages in Cameroon,” Renewable Energy, vol. 34, no. 6, pp. 1445–1450, 2009. [Google Scholar]
21. J. S. Ramos and H. M. Ramos, “Sustainable application of renewable sources in water pumping systems: Optmised energy system configuration,” Energy Policy, vol. 37, no. 2, pp. 633–643, 2009. [Google Scholar]
22. J. Kenfack, F. P. Neirac, T. T. Tatietse, D. Mayer, M. Fogue et al., “Microhydro-PV-hybrid system: Sizing a small hydro-PV-hybrid system for rural electrification in developing countries,” Renewable Energy, vol. 34, no. 10, pp. 2259–2263, 2009. [Google Scholar]
23. A. Asrari, A. Ghasemi and M. H. Javidi, “Economic evaluation of hybrid renewable energy systems for rural electrification in Iran—A case study,” Renewable and Sustainable Energy Reviews, vol. 16, no. 5, pp. 3123–3130, 2012. [Google Scholar]
24. Y. E. A. Eldahab, A. Zekry and N. H. Saad, “Enhancing the optmisation of hybrid renewable energy systems by using statistical calculations and data mining analysis,” International Journal of Renewable Energy Research, vol. 9, no. 2, pp. 868–886, 2019. [Google Scholar]
25. S. K. Nandi and H. R. Ghosh, “Prospect of wind–PV-battery hybrid power system as an alternative to grid extension in Bangladesh,” Energy, vol. 35, no. 7, pp. 3040–3047, 2010. [Google Scholar]
26. G. Rohani and M. Nour, “Techno-economical analysis of stand-alone hybrid renewable power system for Ras Musherib in United Arab Emirates,” Energy, vol. 64, pp. 828–841, 2014. [Google Scholar]
27. K. Kusakana and H. J. Vermaak, “Cost and performance evaluation of hydrokinetic-diesel hybrid systems,” Energy Procedia, vol. 61, pp. 2439–2442, 2014. [Google Scholar]
28. K. Kusakana, “Techno-economic analysis of off-grid hydrokinetic-based hybrid energy systems for onshore/remote area in South Africa,” Energy, vol. 68, pp. 947–957, 2014. [Google Scholar]
29. H. A. Muqeet, I. A. Sajjad, A. Ahmad, M. M. Iqbal, S. Ali et al., “Optimal operation of energy storage system for a prosumer microgrid considering economical and environmental effects,” in RAEE 2019–2019 Int. Symp. on Recent Advances in Electrical Engineering, Slamabad, Pakistan, vol. 4, pp. 1–6, 2019. https://doi.org/10.1109/RAEE.2019.8887002 [Google Scholar] [CrossRef]
30. S. K. Nandi and H. R. Ghosh, “A wind–PV-battery hybrid power system at Sitakunda in Bangladesh,” Energy Policy, vol. 37, no. 9, pp. 3659–3664, 2009. [Google Scholar]
31. B. Kim, S. Bae and H. Kim, “Optimal energy scheduling and transaction mechanism for multiple microgrids,” Energies, vol. 10, no. 4, pp. 566, 2017. https://doi.org/10.3390/en10040566 [Google Scholar] [CrossRef]
32. R. Chedid, A. Sawwas and D. Fares, “Optimal design of a university campus micro-grid operating under unreliable grid considering PV and battery storage,” Energy, vol. 200, pp. 117510, 2020. https://doi.org/10.1016/j.energy.2020.117510 [Google Scholar] [CrossRef]
33. D. Let, B. I. Tene, A. G. Husu, M. F. Stan, L. M. Stancu et al., “Feasibility of a micro grid scale up at campus level-case study,” in 11th Int. Conf. on Electronics, Computers and Artificial Intelligence (ECAI), May 2018, Bucharest, Romania, pp. 1–6, 2019. https://doi.org/10.1109/ECAI46879.2019.9041969 [Google Scholar] [CrossRef]
34. Q. Liu, K. Michael Kamoto and X. Liu, “Microgrids-as-a-service for rural electrification in sub-saharan Africa,” Computers, Materials & Continua, vol. 63, no. 3, pp. 1249–1261, 2020. [Google Scholar]
35. H. C. Hesse, R. Martins, P. Musilek, M. Naumann, C. N. Truong et al., “Economic optmisation of component sizing for residential battery storage systems,” Energies, vol. 10, no. 7, pp. 835, 2017. https://doi.org/10.3390/en10070835 [Google Scholar] [CrossRef]
36. H. Chenoweth, The Rise of University Microgrids, webpage, pp. 1, 2018. https://info.higheredfacilitiesforum.com/blog/the-rise-of-university-microgrids [Google Scholar]
37. D. Syed, A. Zainab, A. Ghrayeb, S. S. Refaat, H. Abu-Rub et al., “Smart grid big data analytics: Survey of technologies, techniques, and applications,” IEEE Access, vol. 9, pp. 59564–59585, 2021. [Google Scholar]
38. C. S. Lai and M. D. McCulloch, “Levelized cost of electricity for solar photovoltaic and electrical energy storage,” Applied Energy, vol. 190, pp. 191–203, 2017. https://doi.org/10.1016/j.apenergy.2016.12.153 [Google Scholar] [CrossRef]
39. H. Javed, H. A. Muqeet, M. Shehzad, M. Jamil, A. A. Khan et al., “Optimal energy management of a campus microgrid considering financial and economic analysis with demand response strategies,” Energies, vol. 14, no. 24, pp. 8501, 2021. https://doi.org/10.3390/en14248501 [Google Scholar] [CrossRef]
40. J. Gulomov, O. Accouche, R. Aliev, M. Azab and I. Gulomova, “Analyzing the zno and ch3nh3pbi3 as emitter layer for silicon based heterojunction solar cells,” Computers, Materials & Continua, vol. 74, no. 1, pp. 575–590, 2023. [Google Scholar]
41. Q. Energy, Qatar Energy Information, Enerdata, 2021. https://www.enerdata.net/estore/energy-market/qatar/ [Google Scholar]
42. I. S. Bayram and M. Koc, “Demand side management for peak reduction and PV integration in Qatar,” in 2017 IEEE 14th Int. Conf. on Networking, Sensing and Control (ICNSC), Calabria, Italy, pp. 251–256, 2017. [Google Scholar]
43. H. A. Muqeet, H. Javed, M. N. Akhter, M. Shahzad, H. M. Munir et al., “Sustainable solutions for advanced energy management system of campus microgrids: Model opportunities and future challenges,” Sensors, vol. 22, no. 6, pp. 1–26, 2022. [Google Scholar]
44. M. Vahedipour-Dahraie, H. Rashidizadeh-Kermani, A. Anvari-Moghaddam and J. M. Guerrero, “Stochastic frequency-security constrained scheduling of a microgrid considering price-driven demand response,” in SPEEDAM 2018-Int. Symp. on Power Electronics, Electrical Drives, Automation and Motion, Amalfi, Italy, pp. 716–721, 2018. https://doi.org/10.1109/SPEEDAM.2018.8445233 [Google Scholar] [CrossRef]
45. S. Ul, H. Hashmi, A. Ulasyar, H. S. Zad, A. Khattak et al., “Optimal day-ahead scheduling for campus microgrid by using MILP approach,” Pakistan Journal of Engineering and Technology, vol. 4, no. 2, pp. 21–27, 2021. [Google Scholar]
46. Y. Yang, Q. Ye, L. J. Tung, M. Greenleaf and H. Li, “Integrated size and energy management design of battery storage to enhance grid integration of large-scale PV power plants,” IEEE Transactions on Industrial Electronics, vol. 65, no. 1, pp. 394–402, 2018. https://doi.org/10.1109/TIE.2017.2721878 [Google Scholar] [CrossRef]
47. A. Kumari and S. Tanwar, “A secure data analytics scheme for multimedia communication in a decentralized smart grid,” Multimedia Tools and Applications, vol. 81, pp. 1–26, 2021. https://doi.org/10.1007/s11042-021-10512-z [Google Scholar] [CrossRef]
48. A. Kumari and S. Tanwar, “Al-based peak load reduction approach for residential buildings using reinforcement learning,” in 2021 Int. Conf. on Computing, Communication, and Intelligent Systems (ICCCISIEEE, Noida, India, pp. 972–977, 2021. https://doi.org/10.1109/ICCCIS51004.2021.9397241 [Google Scholar] [CrossRef]
Cite This Article
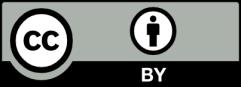