Open Access
ARTICLE
Text-to-Sketch Synthesis via Adversarial Network
1 Department of Information Science and Engineering, NMAM Institute of Technology (Nitte Deemed to be University), Nitte, India
2 Department of Computer Science and Engineering, NMAM Institute of Technology (Nitte Deemed to be University), Nitte, India
3 School of Computing, Skyline University College, University City, Sharjah, UAE
4 Department of Electronics and Communication Engineering, SR University, Warangal, Telangana, India
5 Department of Computer Engineering–AI & BDA, Marwadi University, Rajkot, Gujarat, India
* Corresponding Authors: Sannidhan Manjaya Shetty. Email: ; Biswaranjan Acharya. Email:
(This article belongs to the Special Issue: Susceptibility to Adversarial Attacks and Defense in Deep Learning Systems)
Computers, Materials & Continua 2023, 76(1), 915-938. https://doi.org/10.32604/cmc.2023.038847
Received 31 December 2022; Accepted 18 April 2023; Issue published 08 June 2023
Abstract
In the past, sketches were a standard technique used for recognizing offenders and have remained a valuable tool for law enforcement and social security purposes. However, relying on eyewitness observations can lead to discrepancies in the depictions of the sketch, depending on the experience and skills of the sketch artist. With the emergence of modern technologies such as Generative Adversarial Networks (GANs), generating images using verbal and textual cues is now possible, resulting in more accurate sketch depictions. In this study, we propose an adversarial network that generates human facial sketches using such cues provided by an observer. Additionally, we have introduced an Inverse Gamma Correction Technique to improve the training and enhance the quality of the generated sketches. To evaluate the effectiveness of our proposed method, we conducted experiments and analyzed the results using the inception score and Frechet Inception Distance metrics. Our proposed method achieved an overall inception score of 1.438 ± 0.049 and a Frechet Inception Distance of 65.29, outperforming other state-of-the-art techniques.Keywords
Cite This Article
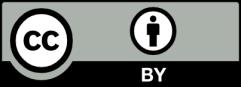