Open Access
EDITORIAL
Deep Learning for COVID-19 Diagnosis via Chest Images
1 School of Computing and Mathematical Sciences, University of Leicester, Leicester, LE1 7RH, UK
2 Department of Information Systems, King Abdulaziz University, Jeddah, 21589, Saudi Arabia
* Corresponding Author: Yudong Zhang. Email:
Computers, Materials & Continua 2023, 76(1), 129-132. https://doi.org/10.32604/cmc.2023.040560
Received 12 March 2023; Accepted 12 April 2023; Issue published 08 June 2023
Abstract
This article has no abstract.COVID-19 is a vastly infectious disease caused by the new coronavirus, officially recognized as severe acute respiratory syndrome coronavirus 2 [1]. This virus has multiplied fast worldwide, causing a global pandemic [2]. It has caused 6.87 million death tolls until 20/March/2023.
The easiest way to stop COVID-19 is to diagnose and quarantine infected patients as rapidly as possible [3]. Both reverse transcription polymerase chain reaction (RT-PCR) [4] and real-time RT-PCR [5] are commonly used to detect the virus nucleic acid from nasopharyngeal swabs. However, they suffer from obvious shortcomings, such as environmental contamination [6], slow reporting (it costs from hours to weeks to receive the outcomes), and high false-negative results [7].
Chest imaging [8] is an effective method for rapid analysis of COVID-19. It is one type of medical imaging technique to examine the body’s chest or thorax area, including the heart, lungs, and other structures. Four common chest imaging scan modalities are available, chest X-ray radiograph (CXR) [9], chest computed tomography (CCT) [10], chest magnetic resonance imaging [11], and chest ultrasound [12]. In clinical practice, CXR and CCT are particularly suitable for diagnosing COVID-19.
Nevertheless, manual diagnosis of COVID-19 from CXR or CCT is a tedious task that requires significant time and effort. The lesions of COVID-19 are displayed with prominent signs of ground-glass opacities (GGOs) [13] in CCT. Moreover, it is challenging for junior radiologists with limited experience to detect GGO regions [14] from chest images. Besides, the manual diagnosis is possibly affected by loads of aspects (sentiment, tiredness, exhaustion, etc.).
However, machine learning (ML) algorithms [15] constantly and precisely follow the pre-designed instructions more speedily and consistently than radiologists do. Additionally, the lesions of early-phase COVID-19 lungs are minor and marginal, like the adjacent healthy tissues. Those small lesions are effortlessly perceived by ML methods [16] but possibly neglected by human experts.
Deep learning (DL) [17] is nowadays the most successful type of ML algorithm. Compared to standard ML algorithms, DL has the following five advantages: (i) DL algorithms normally have higher accuracies than traditional ML algorithms. (ii) DL algorithms have better feature extraction abilities, eliminating the need for manual feature engineering. (iii) DL models can be easily scaled to large-size datasets [18], which is particularly suitable for big data applications. (iv) DL algorithms are more flexible than traditional ML algorithms [19]. (v) DL algorithms do not need as much human help as traditional ML algorithms, reducing human intervention [20].
Many successful DL algorithms have been proposed in previous years. One successful algorithm is the deep rank-based average pooling network (DRAPNet) [21], published in this journal. This paper was indexed as ESI highly cited paper recently. In the paper, the authors first introduce enhanced multiple-way data augmentation [22]. Second, the authors present the n-conv rank-based average pooling module [21]. Third, the authors propose their DRAPNet based on NRAPM. Finally, heatmaps are produced based on Grad-CAM for explainable analysis. The authors tested their DRAPNet algorithm on a four-class dataset. DRAPNet has attained a 95.49% micro-averaged F1 score [21].
There are many other successful DL-based COVID-19 diagnosis algorithms, such as AVNC [23], deep stacked sparse autoencoder analytical (DSSAE) [24], IFFA-DTLMS [25], deep stacked ensemble learning model [26], ANC [27], etc.
Because of the limited sizes of the open-access datasets with ground truth labels, weakly supervised learning (WSL) [28], trained by weak labels or partial annotations, is particularly suitable for constructing efficient COVID-19 models [29]. WSL can attain excellent results for limited-labeled datasets, where acquiring accurately labeled samples are expensive or challenging [30].
COVID-19 has been widespread worldwide for three years and five months. We believe ML and DL can continue to help diagnose COVID-19. Firstly, those algorithms can be used to develop more accurate and reliable algorithms for automated diagnosis of COVID-19, which can save radiologists time and effort. Secondly, ML and DL algorithms can predict COVID-19 disease progression and prognosis [31], helping identify high-risk patients who may require more intensive treatment. Thirdly, ML and DL can identify patterns and correlations between imaging findings and clinical outcomes, leading to the discovery of new biomarkers [32] and more effective treatments.
Overall, ML and DL have the potential to significantly improve the accuracy and efficiency of COVID-19 diagnosis and treatment planning based on chest images.
Funding Statement: This paper is partially supported by 12 UK grants: Sino-UK Education Fund (OP202006); MRC (MC_PC_17171); Royal Society (RP202G0230); BHF (AA/18/3/34220); Hope Foundation for Cancer Research (RM60G0680); GCRF (P202PF11); BBSRC (RM32G0178B8); Sino-UK Industrial Fund (RP202G0289); LIAS (P202ED10 & P202RE969); Data Science Enhancement Fund (P202RE237); Fight for Sight (24NN201).
Conflicts of Interest: The authors declare that they have no conflicts of interest to report regarding the present study.
References
1. X. W. Gu, S. W. Chen, H. S. Zhu and M. Brown, “COVID-19 imaging detection in the context of artificial intelligence and the internet of things,” CMES-Computer Modeling in Engineering & Sciences, vol. 132, pp. 507–530, 2022. [Google Scholar]
2. K. T. Rune and J. J. Keech, “Is it time to stock up? Understanding panic buying during the COVID-19 pandemic,” Australian Journal of Psychology, vol. 75, Article ID: 2180299, 2023. [Google Scholar]
3. J. Liu, J. Qi, W. Chen, Y. Wu and Y. J. Nian, “Deep learning for detecting COVID-19 using medical images,” Bioengineering-Basel, vol. 10, Article ID: 19, 2023. [Google Scholar]
4. R. Ak, E. Yilmaz, A. U. Seyhan and F. Doganay, “Recurrence of COVID-19 documented with rt-pcr,” JCPSP-Journal of the College of Physicians and Surgeons Pakistan, vol. 31, pp. S26–S28, 2021. [Google Scholar]
5. P. M. Etaware, “Virtual system for early detection of COVID-19 infection “etaware-cdt-2020 prototype design” (corroborated by rrt-pcr data),” Biomedical Signal Processing and Control, vol. 73, Article ID: 103337, 2022. [Google Scholar]
6. M. Sawano, K. Takeshita, H. Ohno and H. Oka, “A short perspective on a COVID-19 clinical study: ‘Diagnosis of COVID-19 by rt-pcr using exhale breath condensate samples’,” Journal of Breath Research, vol. 14, Article ID: 042003, 2020. [Google Scholar]
7. V. Pecoraro, A. Negro, T. Pirotti and T. Trenti, “Estimate false-negative rt-pcr rates for sars-cov-2. A systematic review and meta-analysis,” European Journal of Clinical Investigation, vol. 52, Article ID: e13706, 2022. [Google Scholar]
8. T. T. Ho, W. J. Kim, C. H. Lee, G. Y. Jin, K. J. Chae et al., “An unsupervised image registration method employing chest computed tomography images and deep neural networks,” Computers in Biology and Medicine, vol. 154, Article ID: 106612, 2023. [Google Scholar]
9. N. Gaggion, L. Mansilla, C. Mosquera, D. H. Milone and E. Ferrante, “Improving anatomical plausibility in medical image segmentation via hybrid graph neural networks: Applications to chest X-ray analysis,” IEEE Transactions on Medical Imaging, vol. 42, pp. 546–556, 2023. [Google Scholar] [PubMed]
10. S. Bazdar, A. Kwee, L. Houweling, Y. D. de Wit-van Wijck, F. Hoesein et al., “A systematic review of chest imaging findings in long covid patients,” Journal of Personalized Medicine, vol. 13, Article ID: 282, 2023. [Google Scholar]
11. L. Wucherpfennig, S. M. F. Triphan, S. Wege, H. U. Kauczor, C. P. Heussel et al., “Magnetic resonance imaging detects improvements of pulmonary and paranasal sinus abnormalities in response to elexacaf tor/tezacaf tor/ivacaf tor therapy in adults with cystic fibrosis,” Journal of Cystic Fibrosis, vol. 21, pp. 1053–1060, 2022. [Google Scholar] [PubMed]
12. S. Montgomery, F. R. Li, C. Funk, E. Peethumangsin, M. Morris et al., “Detection of pneumothorax on ultrasound using artificial intelligence,” Journal of Trauma and Acute Care Surgery, vol. 94, pp. 379–384, 2023. [Google Scholar] [PubMed]
13. A. Ali, A. Adebayo and J. Ali, “Ground glass opacities in a patient receiving treatment with all-trans retinoic acid and arsenic trioxide,” Chest, vol. 162, pp. 1299A–1300A, 2022. [Google Scholar]
14. K. B. Lewandowska, A. Lewandowska, I. Baranska, M. Klatt, E. Augstynowicz-Kopec et al., “Not always covid-pulmonary bcgosis as an unexpected cause of severe respiratory failure and massive ground glass opacities in the lungs,” European Respiratory Journal, vol. 60, pp. 3176, 2022. [Google Scholar]
15. K. H. Abegaz and I. Etikan, “Boosting the performance of artificial intelligence-driven models in predicting COVID-19 mortality in Ethiopia,” Diagnostics, vol. 13, Article ID: 658, 2023. [Google Scholar]
16. M. A. Marzaleh, M. Peyravi, S. Mousavi, F. Sarpourian, M. Seyedi et al., “Artificial intelligence functionalities during the COVID-19 pandemic,” Disaster Medicine and Public Health Preparedness, vol. 17, pp. e336, Article ID: e336, 2023. [Google Scholar]
17. D. A. Boiko, A. S. Kashin, V. R. Sorokin, Y. Agaev, R. G. Zaytsev et al., “Analyzing ionic liquid systems using real-time electron microscopy and a computational framework combining deep learning and classic computer vision techniques,” Journal of Molecular Liquids, vol. 376, Article ID: 121407, 2023. [Google Scholar]
18. D. Blondeau-Patissier, T. Schroeder, G. Suresh, Z. B. Li, F. I. Diakogiannis et al., “Detection of marine oil-like features in sentinel-1 sar images by supplementary use of deep learning and empirical methods: Performance assessment for the great barrier reef marine park,” Marine Pollution Bulletin, vol. 188, Article ID: 114598, 2023. [Google Scholar]
19. R. Arablouei, L. Wang, L. Currie, J. Yates, F. A. P. Alvarenga et al., “Animal behavior classification via deep learning on embedded systems,” Computers and Electronics in Agriculture, vol. 207, Article ID: 107707, 2023. [Google Scholar]
20. F. Ramezani, S. Parvez, J. P. Fix, A. Battaglin, S. Whyte et al., “Automatic detection of multilayer hexagonal boron nitride in optical images using deep learning-based computer vision,” Scientific Reports, vol. 13, 2023. [Google Scholar]
21. S. -H. Wang, K. M. Attique and G. Vishnuvarthanan, “Deep rank-based average pooling network for COVID-19 recognition,” Computers, Materials, & Continua, pp. 2797–2813, 2022. [Google Scholar]
22. Y. Shehu and R. Harper, “Enhancements to language modeling techniques for adaptable log message classification,” IEEE Transactions on Network and Service Management, vol. 19, pp. 4662–4675, 2022. [Google Scholar]
23. S. -H. Wang and S. Fernandes, “Avnc: Attention-based vgg-style network for COVID-19 diagnosis by cbam,” IEEE Sensors Journal, vol. 22, pp. 17431–17438, 2022. [Google Scholar] [PubMed]
24. S. -H. Wang, “Dssae: Deep stacked sparse autoencoder analytical model for COVID-19 diagnosis by fractional Fourier entropy,” ACM Transactions on Management Information Systems, vol. 13, Article ID: 2, 2021. [Google Scholar]
25. M. Ragab, M. W. Al-Rabia, S. S. Binyamin and A. A. Aldarmahi, “Intelligent firefly algorithm deep transfer learning based COVID-19 monitoring system,” CMC-Computers Materials & Continua, vol. 74, pp. 2889–2903, 2023. [Google Scholar]
26. G. Madhu, B. L. Bharadwaj, R. Boddeda, S. Vardhan, K. S. Kautish et al., “Deep stacked ensemble learning model for COVID-19 classification,” CMC-Computers Materials & Continua, vol. 70, pp. 5467–5486, 2022. [Google Scholar]
27. Y. -D. Zhang, “Anc: Attention network for COVID-19 explainable diagnosis based on convolutional block attention module,” Computer Modeling in Engineering & Sciences, vol. 127, pp. 1037–1058, 2021. [Google Scholar]
28. A. Mohammed, C. C. Wang, M. Zhao, M. Ullah, R. Naseem et al., “Weakly-supervised network for detection of COVID-19 in chest ct scans,” IEEE Access, vol. 8, pp. 155987–156000, 2020. [Google Scholar] [PubMed]
29. R. Karthik, R. Menaka, M. Hariharan and D. Won, “Ct-based severity assessment for COVID-19 using weakly supervised non-local CNN,” Applied Soft Computing, vol. 121, Article ID: 108765, 2022. [Google Scholar]
30. M. S. Kim, B. Y. Lim, H. S. Shin and H. Y. Kwon, “Historical credibility for movie reviews and its application to weakly supervised classification,” Information Sciences, vol. 630, pp. 325–340, 2023. [Google Scholar]
31. N. R. Sivakumar, “Ensemble deep learning for iot based COVID 19 health care pollution monitor,” Intelligent Automation and Soft Computing, vol. 35, 2023. [Google Scholar]
32. T. Nguyen, H. H. Pham, K. H. Le, A. T. Nguyen, T. Thanh et al., “Detecting COVID-19 from digitized ECG printouts using 1d convolutional neural networks,” PLoS One, vol. 17, Article ID: e0277081, 2022. [Google Scholar]
Cite This Article
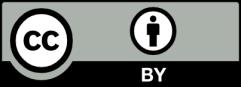