Open Access
ARTICLE
An Efficient Sleep Spindle Detection Algorithm Based on MP and LSBoost
1 School of Software, South China Normal University, Foshan, 528225, China
2 Pazhou Lab, Guangzhou, 510330, China
3 School of Biomedical Sciences and Engineering, South China University of Technology, Guangzhou, 510650, China
4 Department of Psychiatry, New York University School of Medicine, New York, 10016, USA
* Corresponding Author: Haiyun Huang. Email:
Computers, Materials & Continua 2023, 76(2), 2301-2316. https://doi.org/10.32604/cmc.2023.037727
Received 15 November 2022; Accepted 11 April 2023; Issue published 30 August 2023
Abstract
Sleep spindles are an electroencephalogram (EEG) biomarker of non-rapid eye movement (NREM) sleep and have important implications for clinical diagnosis and prognosis. However, it is challenging to accurately detect sleep spindles due to the complexity of the human brain and the uncertainty of neural mechanisms. To improve the reliability and objectivity of sleep spindle detection and to compensate for the limitations of manual annotation, this study proposes a new automatic detection algorithm based on Matching Pursuit (MP) and Least Squares Boosting (LSBoost), where the automatic sleep spindle detection algorithm can help reduce the visual annotation workload of sleep clinicians. Specifically, MP is a time-frequency analysis method suitable for extracting spindle wave characteristics, which can accurately locate spindle waves on a time-frequency plane. LSBoost is an ensemble learning classification method to deal with unbalanced data. Initially, the MP method is used to search for EEG segments that are possible spindle waves from the filtered raw EEG data. Then, the designed feature segments are thrown into the LSBoost classifier to further identify the real spindles from all candidates and output the final results. The proposed method is verified on the common public dataset DREAMS. The experiment results show that the sensitivity and F1-scores based on the sample-based assessments achieve 68.2% and 55.4%, respectively. Furthermore, the Recall and F1-score based on the event assessments are 83.8% and 70.8%, respectively. These results show that the proposed algorithm is robust to the subject changes in the DREAMS dataset. In addition, it improves the quality of sleep spindle detection, which is expected to assist the manual marking of experts.Keywords
Cite This Article
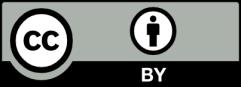