Open Access
ARTICLE
DFE-GCN: Dual Feature Enhanced Graph Convolutional Network for Controversy Detection
1 College of Software, Xinjiang University, Urumqi, 830000, China
2 Key Laboratory of Signal Detection and Processing in Xinjiang Uygur Autonomous Region, Xinjiang University, Urumqi, 830000, China
3 Key Laboratory of Multilingual Information Technology in Xinjiang Uygur Autonomous Region, Xinjiang University, Urumqi, 830000, China
* Corresponding Author: Wenzhong Yang. Email:
Computers, Materials & Continua 2023, 77(1), 893-909. https://doi.org/10.32604/cmc.2023.040862
Received 01 April 2023; Accepted 07 August 2023; Issue published 31 October 2023
Abstract
With the development of social media and the prevalence of mobile devices, an increasing number of people tend to use social media platforms to express their opinions and attitudes, leading to many online controversies. These online controversies can severely threaten social stability, making automatic detection of controversies particularly necessary. Most controversy detection methods currently focus on mining features from text semantics and propagation structures. However, these methods have two drawbacks: 1) limited ability to capture structural features and failure to learn deeper structural features, and 2) neglecting the influence of topic information and ineffective utilization of topic features. In light of these phenomena, this paper proposes a social media controversy detection method called Dual Feature Enhanced Graph Convolutional Network (DFE-GCN). This method explores structural information at different scales from global and local perspectives to capture deeper structural features, enhancing the expressive power of structural features. Furthermore, to strengthen the influence of topic information, this paper utilizes attention mechanisms to enhance topic features after each graph convolutional layer, effectively using topic information. We validated our method on two different public datasets, and the experimental results demonstrate that our method achieves state-of-the-art performance compared to baseline methods. On the Weibo and Reddit datasets, the accuracy is improved by 5.92% and 3.32%, respectively, and the F1 score is improved by 1.99% and 2.17%, demonstrating the positive impact of enhanced structural features and topic features on controversy detection.Keywords
Cite This Article
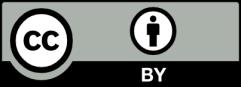