Open Access
ARTICLE
Dense Spatial-Temporal Graph Convolutional Network Based on Lightweight OpenPose for Detecting Falls
1 School of Computer Science, Nanjing University of Information Science & Technology, Nanjing, 210044, China
2 Wuxi Research Institute, Nanjing University of Information Science & Technology, Wuxi, 214100, China
3 Jiangsu Collaborative Innovation Center of Atmospheric Environment and Equipment Technology (CICAEET), Nanjing University of Information Science & Technology, Nanjing, 210044, China
4 School of Automation, Nanjing University of Information Science & Technology, Nanjing, 210044, China
5 School of Artificial Intelligence, Nanjing University of Information Science & Technology, Nanjing, 210044, China
* Corresponding Author: Xiaorui Zhang. Email:
Computers, Materials & Continua 2023, 77(1), 47-61. https://doi.org/10.32604/cmc.2023.042561
Received 04 June 2023; Accepted 04 September 2023; Issue published 31 October 2023
Abstract
Fall behavior is closely related to high mortality in the elderly, so fall detection becomes an important and urgent research area. However, the existing fall detection methods are difficult to be applied in daily life due to a large amount of calculation and poor detection accuracy. To solve the above problems, this paper proposes a dense spatial-temporal graph convolutional network based on lightweight OpenPose. Lightweight OpenPose uses MobileNet as a feature extraction network, and the prediction layer uses bottleneck-asymmetric structure, thus reducing the amount of the network. The bottleneck-asymmetrical structure compresses the number of input channels of feature maps by 1 × 1 convolution and replaces the 7 × 7 convolution structure with the asymmetric structure of 1 × 7 convolution, 7 × 1 convolution, and 7 × 7 convolution in parallel. The spatial-temporal graph convolutional network divides the multi-layer convolution into dense blocks, and the convolutional layers in each dense block are connected, thus improving the feature transitivity, enhancing the network’s ability to extract features, thus improving the detection accuracy. Two representative datasets, Multiple Cameras Fall dataset (MCF), and Nanyang Technological University Red Green Blue + Depth Action Recognition dataset (NTU RGB + D), are selected for our experiments, among which NTU RGB + D has two evaluation benchmarks. The results show that the proposed model is superior to the current fall detection models. The accuracy of this network on the MCF dataset is 96.3%, and the accuracies on the two evaluation benchmarks of the NTU RGB + D dataset are 85.6% and 93.5%, respectively.Keywords
Cite This Article
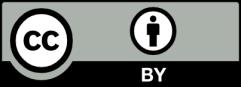