Open Access
ARTICLE
ProNet Adaptive Retinal Vessel Segmentation Algorithm Based on Improved UperNet Network
1 CW Chu College, Jiangsu Normal University, Xuzhou, 221000, China
2 Biomedical Engineering College, Southern Medical University, Guangzhou, 510080, China
* Corresponding Author: Sijia Zhu. Email:
(This article belongs to the Special Issue: Recent Advances in Ophthalmic Diseases Diagnosis using AI)
Computers, Materials & Continua 2024, 78(1), 283-302. https://doi.org/10.32604/cmc.2023.045506
Received 29 August 2023; Accepted 13 November 2023; Issue published 30 January 2024
Abstract
This paper proposes a new network structure, namely the ProNet network. Retinal medical image segmentation can help clinical diagnosis of related eye diseases and is essential for subsequent rational treatment. The baseline model of the ProNet network is UperNet (Unified perceptual parsing Network), and the backbone network is ConvNext (Convolutional Network). A network structure based on depth-separable convolution and 1 × 1 convolution is used, which has good performance and robustness. We further optimise ProNet mainly in two aspects. One is data enhancement using increased noise and slight angle rotation, which can significantly increase the diversity of data and help the model better learn the patterns and features of the data and improve the model's performance. Meanwhile, it can effectively expand the training data set, reduce the influence of noise and abnormal data in the data set on the model, and improve the accuracy and reliability of the model. Another is the loss function aspect, and we finally use the focal loss function. The focal loss function is well suited for complex tasks such as object detection. The function will penalise the loss carried by samples that the model misclassifies, thus enabling better training of the model to avoid these errors while solving the category imbalance problem as a way to improve image segmentation density and segmentation accuracy. From the experimental results, the evaluation metrics mIoU (mean Intersection over Union) enhanced by 4.47%, and mDice enhanced by 2.92% compared to the baseline network. Better generalization effects and more accurate image segmentation are achieved.Keywords
Cite This Article
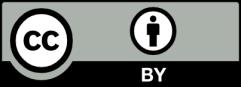