Open Access
ARTICLE
Target Detection Algorithm in Foggy Scenes Based on Dual Subnets
1 School of Computer Science, Jiangsu University of Science and Technology, Zhenjiang, 212100, China
2 Information Department, China Merchants Heavy Industry, Nantong, 226116, China
* Corresponding Author: Yuecheng Yu. Email:
(This article belongs to the Special Issue: Deep Learning based Object Detection and Tracking in Videos)
Computers, Materials & Continua 2024, 78(2), 1915-1931. https://doi.org/10.32604/cmc.2024.046125
Received 19 September 2023; Accepted 11 December 2023; Issue published 27 February 2024
Abstract
Under the influence of air humidity, dust, aerosols, etc., in real scenes, haze presents an uneven state. In this way, the image quality and contrast will decrease. In this case, It is difficult to detect the target in the image by the universal detection network. Thus, a dual subnet based on multi-task collaborative training (DSMCT) is proposed in this paper. Firstly, in the training phase, the Gated Context Aggregation Network (GCANet) is used as the supervisory network of YOLOX to promote the extraction of clean information in foggy scenes. In the test phase, only the YOLOX branch needs to be activated to ensure the detection speed of the model. Secondly, the deformable convolution module is used to improve GCANet to enhance the model’s ability to capture details of non-homogeneous fog. Finally, the Coordinate Attention mechanism is introduced into the Vision Transformer and the backbone network of YOLOX is redesigned. In this way, the feature extraction ability of the network for deep-level information can be enhanced. The experimental results on artificial fog data set FOG_VOC and real fog data set RTTS show that the map value of DSMCT reached 86.56% and 62.39%, respectively, which was 2.27% and 4.41% higher than the current most advanced detection model. The DSMCT network has high practicality and effectiveness for target detection in real foggy scenes.Keywords
Cite This Article
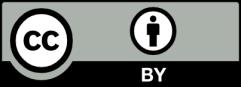
This work is licensed under a Creative Commons Attribution 4.0 International License , which permits unrestricted use, distribution, and reproduction in any medium, provided the original work is properly cited.