Open Access
ARTICLE
Unmanned Ship Identification Based on Improved YOLOv8s Algorithm
1 Key Laboratory of Modern Power System Simulation and Control & Renewable Energy Technology, School of Electrical Engineering, Northeast Electric Power University, Jilin, 132012, China
2 School of Biomedical Engineering, Taiyuan University of Technology, Taiyuan, 030600, China
* Corresponding Author: Jin Lei. Email:
(This article belongs to the Special Issue: Machine Vision Detection and Intelligent Recognition)
Computers, Materials & Continua 2024, 78(3), 3071-3088. https://doi.org/10.32604/cmc.2023.047062
Received 23 October 2023; Accepted 29 November 2023; Issue published 26 March 2024
Abstract
Aiming at defects such as low contrast in infrared ship images, uneven distribution of ship size, and lack of texture details, which will lead to unmanned ship leakage misdetection and slow detection, this paper proposes an infrared ship detection model based on the improved YOLOv8 algorithm (R_YOLO). The algorithm incorporates the Efficient Multi-Scale Attention mechanism (EMA), the efficient Reparameterized Generalized-feature extraction module (CSPStage), the small target detection header, the Repulsion Loss function, and the context aggregation block (CABlock), which are designed to improve the model’s ability to detect targets at multiple scales and the speed of model inference. The algorithm is validated in detail on two vessel datasets. The comprehensive experimental results demonstrate that, in the infrared dataset, the YOLOv8s algorithm exhibits improvements in various performance metrics. Specifically, compared to the baseline algorithm, there is a 3.1% increase in mean average precision at a threshold of 0.5 (mAP (0.5)), a 5.4% increase in recall rate, and a 2.2% increase in mAP (0.5:0.95). Simultaneously, while less than 5 times parameters, the mAP (0.5) and frames per second (FPS) exhibit an increase of 1.7% and more than 3 times, respectively, compared to the CAA_YOLO algorithm. Finally, the evaluation indexes on the visible light data set have shown an average improvement of 4.5%.Keywords
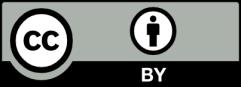