Open Access
ARTICLE
Effective Denoising Architecture for Handling Multiple Noises
The Graduate School of Business IT of Kookmin University, Seoul, 02707, Korea
* Corresponding Author: Namgyu Kim. Email:
Computer Systems Science and Engineering 2023, 44(3), 2667-2682. https://doi.org/10.32604/csse.2023.029732
Received 10 March 2022; Accepted 18 April 2022; Issue published 01 August 2022
Abstract
Object detection, one of the core research topics in computer vision, is extensively used in various industrial activities. Although there have been many studies of daytime images where objects can be easily detected, there is relatively little research on nighttime images. In the case of nighttime, various types of noises, such as darkness, haze, and light blur, deteriorate image quality. Thus, an appropriate process for removing noise must precede to improve object detection performance. Although there are many studies on removing individual noise, only a few studies handle multiple noises simultaneously. In this paper, we propose a convolutional denoising autoencoder (CDAE)-based architecture trained on various types of noises. We also present various composing modules for each noise to improve object detection performance for night images. Using the exclusively dark (ExDark) Image dataset, experimental results show that the Sequential filtering architecture showed superior mean average precision(mAP) compared to other architectures.Keywords
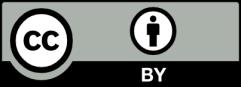