Open Access
ARTICLE
Deep Learning-Based Digital Image Forgery Detection Using Transfer Learning
1 Center of Excellence in Cybercrimes and Digital Forensics (CoECDF), Naif Arab University for Security Sciences (NAUSS), Riyadh, 14812, Saudi Arabia
2 School of Engineering, Information Technology and Physical Sciences, Federation University, Brisbane,QLD 4000, Australia
* Corresponding Author: Emad Ul Haq Qazi. Email:
Intelligent Automation & Soft Computing 2023, 38(3), 225-240. https://doi.org/10.32604/iasc.2023.041181
Received 13 April 2023; Accepted 26 June 2023; Issue published 27 February 2024
Abstract
Deep learning is considered one of the most efficient and reliable methods through which the legitimacy of a digital image can be verified. In the current cyber world where deepfakes have shaken the global community, confirming the legitimacy of a digital image is of great importance. With the advancements made in deep learning techniques, now we can efficiently train and develop state-of-the-art digital image forensic models. The most traditional and widely used method by researchers is convolution neural networks (CNN) for verification of image authenticity but it consumes a considerable number of resources and requires a large dataset for training. Therefore, in this study, a transfer learning based deep learning technique for image forgery detection is proposed. The proposed methodology consists of three modules namely; preprocessing module, convolutional module, and the classification module. By using our proposed technique, the training time is drastically reduced by utilizing the pre-trained weights. The performance of the proposed technique is evaluated by using benchmark datasets, i.e., BOW and BOSSBase that detect five forensic types which include JPEG compression, contrast enhancement (CE), median filtering (MF), additive Gaussian noise, and resampling. We evaluated the performance of our proposed technique by conducting various experiments and case scenarios and achieved an accuracy of 99.92%. The results show the superiority of the proposed system.Keywords
Cite This Article
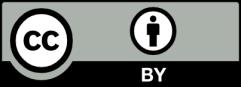