Open Access
ARTICLE
A Deep Reinforcement Learning-Based Technique for Optimal Power Allocation in Multiple Access Communications
1 Department of Industrial Engineering, College of Engineering, University of Houston, Houston, TX, 77204, USA
2 Department of Computer Science, Iowa State University, Ames, Iowa, USA
3 ETSI de Telecomunicación, Universidad Politécnica de Madrid, Madrid, Spain
4 Faculty of School of Plant and Environmental Sciences, Virginia Polytechnic Institute and State University, Blacksburg, Virginia, 24060, USA
* Corresponding Author: Diego Martín. Email:
Intelligent Automation & Soft Computing 2024, 39(1), 93-108. https://doi.org/10.32604/iasc.2024.042693
Received 08 June 2023; Accepted 24 January 2024; Issue published 29 March 2024
Abstract
For many years, researchers have explored power allocation (PA) algorithms driven by models in wireless networks where multiple-user communications with interference are present. Nowadays, data-driven machine learning methods have become quite popular in analyzing wireless communication systems, which among them deep reinforcement learning (DRL) has a significant role in solving optimization issues under certain constraints. To this purpose, in this paper, we investigate the PA problem in a -user multiple access channels (MAC), where transmitters (e.g., mobile users) aim to send an independent message to a common receiver (e.g., base station) through wireless channels. To this end, we first train the deep Q network (DQN) with a deep Q learning (DQL) algorithm over the simulation environment, utilizing offline learning. Then, the DQN will be used with the real data in the online training method for the PA issue by maximizing the sumrate subjected to the source power. Finally, the simulation results indicate that our proposed DQN method provides better performance in terms of the sumrate compared with the available DQL training approaches such as fractional programming (FP) and weighted minimum mean squared error (WMMSE). Additionally, by considering different user densities, we show that our proposed DQN outperforms benchmark algorithms, thereby, a good generalization ability is verified over wireless multi-user communication systems.Keywords
Cite This Article
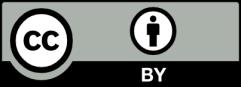