Open Access
ABSTRACT
Machine Learning Prediction of Creep Rupture Time for Steels
Research and Services Division of Materials Data and Integrated System, National Institute for Materials Science, Sengen, Tsukuba, Japan.
Research Center for Advanced Science and Technology, University of Tokyo, Tokyo, Japan.
*Corresponding Author: Masahiko Demura. Email: .
The International Conference on Computational & Experimental Engineering and Sciences 2019, 22(2), 123-123. https://doi.org/10.32604/icces.2019.05303
Abstract
Creep is a complicated and time-dependent phenomenon, which is affected by the initial state and the degradation of microstructures. It is thus considered that the information about the microstructure is essential to predict the creep rupture time. On the other hand, there is a strong, practical need for the prediction without the investigation of microstructures nor the disclosure of the detailed process that should control the initial microstructures. In this study, we examined how modern machine learning technique can help to predict the creep rupture time in heat-resistant ferrite-type steels without the direct information about the microstructures and the process by using the high-quality experimental data generated in NIMS. The descriptors were here chemical composition expressed by each mass percent of 20 elements including Fe and test conditions such as creep stress and temperature. We used XGBoost for the machine learning model, one of the cutting-edge ensemble tree regression models, together with Tree Parzen estimator algorithm for tuning hyperparameters.The result demonstrated that the learned model provided a reasonable prediction. The root mean square error was 0.485 in natural logarithm of creep rupture time between the predicted and the experimental values for the test data that were not used for the training. This means that the creep rupture time can be predicted with an error within 1.7 (e^0.485) times. Note that we used only the chemical composition and the test conditions to build the model. It indicates that the information about the microstructures could be indirectly included in those descriptors. In the presentation, we will discuss on this point.
This work was supported by Council for Science, Technology and Innovation (CSTI), Cross-ministerial Strategic Innovation Promotion Program (SIP), “Structural Materials for Innovation” and “Materials Integration for Revolutionary Design System of Structural Materials” (Funding agency: JST).
Cite This Article
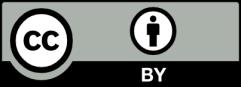