Open Access
ARTICLE
Computational Verification of Low-Frequency Broadband Noise from Wind Turbine Blades Using Semi-Empirical Methods
Department of Mechanical Engineering, School of Technology, GITAM (Deemed to be University), Hyderabad, 502319, India
* Corresponding Author: Vasishta Bhargava Nukala. Email:
Sound & Vibration 2024, 58, 133-150. https://doi.org/10.32604/sv.2024.047762
Received 16 November 2023; Accepted 24 January 2024; Issue published 19 March 2024
Abstract
A significant aerodynamic noise from wind turbines arises when the rotating blades interact with turbulent flows. Though the trailing edge of the blade is an important source of noise at high frequencies, the present work deals with the influence of turbulence distortion on leading edge noise from wind turbine blades which becomes significant in low-frequency regions. Four quasi-empirical methods are studied to verify the accuracy of turbulent inflow noise predicted at low frequencies for a 2 MW horizontal axis wind turbine. Results have shown that all methods exhibited a downward linear trend in noise spectra for a given mean wind speed except at very low frequencies. With an increase in turbulence intensity from 6% to 14%, the sound power was found to increase almost linearly, and the standard error for sound power was reduced for all methods studied. The computed results were validated and agreed well with experiment noise data from Siemens SWT-2.3MW 93 wind turbine.Keywords
Nomenclature
U | Mean or free stream wind speed |
I | Turbulence intensity |
σu | Standard deviation for longitudinal wind speed component |
k, kx, ky, ke | Wave number, Turbulence wavenumber in chord and blade span directions, Turbulent wavenumber of eddies |
Re | Reynolds number |
M | Mach number |
c | Chord length of aerofoil |
L | Span length of aerofoil |
Ω, ω | Rotational speed in RPM, Circular frequency |
R | Blade radius |
re | Effective (projected) distance from source to observer |
Lw | Sound power |
SPL | Sound pressure level |
SPL1/3 | 1/3rd octave sound pressure |
DL, DH | Low & high-frequency directivity functions |
f | 1/3rd octave band frequency |
l | Turbulence length scale |
ρ | Air density |
u | Local flow velocity over the blade span |
S | Acoustic transfer function |
β | Prandtl-Glauert compressibility factor |
ϴ | Directivity angle between the observer and trailing edge of the source along span |
Ф | Directivity angle between the observer and trailing edge of source along chord |
Mc | Convective Mach number |
p, pref | rms acoustic pressure, reference acoustic pressure |
TI | Turbulence Intensity |
H | Height above ground |
B | Number of blades |
φz φww | Longitudinal turbulence spectrum, Turbulence velocity spectrum |
dB | Decibel |
K1 | Frequency dependent scaling function |
w, wr | rms and Reference turbulence intensity |
μPa | Micro Pascal |
Renewable energy is one of the fastest growing energy sources and accounts for more than 10% of global power generation in the world today to tackle power deficits and climate changes across the world Doolan [1]. Among all renewable energy technologies, wind power plants are one of the most important and efficient methods to harness wind energy. As more wind farms are installed across the world, acoustic emissions from wind turbines are seen as a constraint by many establishments. Pedersen et al. [2] conducted a comparative dose-response study on the effects of wind turbine noise from a group of wind farms in the Netherlands and Sweden. They found that most of the noise from wind turbines was found to be visually annoying and caused sleep disturbances compared to other community noise sources from aircraft, railways, road traffic, and shipyards industries [3]. Several researchers, including Moreau et al. [4], Moriarty et al. [5], Grosveld [6], Oerlemans [7], Brooks et al. [8], Lowson [9,10], Amiet [11], Hubbard et al. [12], Kim et al. [13], and van den Berg [14], have conducted studies on flow-induced noise from airfoils used in various applications such as compressors, gas turbines, and helicopters. They used numerical models to predict aerodynamically generated noise, and their findings were reliable. Numerous acoustic experiment techniques and analytical methods have been developed by Kerscher et al. [15], Butt et al. [16], Kümmritz et al. [17], and Licitra et al. [18] to predict the trailing edge noise radiation for different aerodynamic flow conditions. To a large extent, the acoustic properties of airfoil self-noise and turbulent inflow noise sources were based on the boundary layer turbulence parameters, turbulence intensity, and integral length scales. Noise radiated can be exhibited in the form of a dipole or as a quad pole at different frequencies and dependent on mean flow velocity as well as fluctuating velocity. Also, the noise that arises from rotating blades of wind turbine generators is often perceived by inhabitants near wind farms either as “swish” or “thump” type characteristics and undergoes amplitude modulation that occurs at blade passing frequency. The amount of sound produced by a wind turbine is closely linked to the average wind speed measured at the hub height of the turbine, according to studies conducted by Makarewicz et al. [19] and Bowdler [20]. One of the key sources of broadband noise is the inflow turbulent noise that is not yet fully comprehended, as it relies on rapidly changing turbulent velocity spectra. At low frequencies, this type of noise source can also exhibit a periodic or random impulsive type characteristic in the near field. The acoustic waves produced at low frequencies do not get attenuated and tend to undergo amplitude modulation in a far field which can cause serious annoyance patterns among inhabitants living near wind farms. The extent of perceived annoyance level is high when overall noise contains a significant amount of low-frequency content and increases more rapidly than high-frequency noise. Though turbulent inflow noise has been studied extensively using numerical and experimental methods, recently Faria et al. [21] have investigated this noise source by applying rapid distortion theory (RDT) on a flat plate and NACA 0008 airfoil. The rapid distortion theory is also essentially a semi-empirical approach to characterize the turbulence velocity field accurately and predict the inflow turbulence noise well. Faria et al. [21] applied the assumptions for the RDT criterion and verified for different chord lengths, thickness to chord ratio, and turbulent intensity levels which varied up to the range 0.15 m, 8%, and 4%, respectively. For wind turbines, the interaction of turbulence with the leading edge of the blade is a significant source of noise which depends not only on turbulence intensity but also on the length scale of incoming eddies. The size of eddies and turbulence intensity levels play a key role in noise generation at low frequencies. The objective of this paper is primarily dependent on the methods study which investigates the accuracy of modified rapid distortion theory (RDT) to predict turbulence inflow noise when compared to those methods used by Moriarty et al. [5] in the low-frequency part of noise spectra. To the author’s knowledge, this work has not been done in the past and therefore it aids in understanding the qualitative accuracy of semi-empirical method predictions based on the turbulence properties of the velocity energy spectrum. A sensitivity analysis has been performed by varying turbulence intensity and mean wind speed parameters to understand its influence on the aerodynamic noise generated from the leading edge of wind turbine blades. In Section 2, four turbulence inflow noise methods are described based on mean flow velocity, turbulence intensity, length scale, and directivity parameters. In Section 3, a description of the computational setup is presented and consists of a solver to compute the local velocity field on wind turbine blades that is coupled to a noise solver which uses acoustic equations to evaluate the noise data. Section 4 discusses about validation of results obtained for turbulent inflow noise predicted from studied quasi-empirical methods implemented on a 2 MW horizontal axis wind turbine with a blade length of 38 m. Further, the effects of turbulence intensity and mean flow velocity on the turbulence inflow noise are analyzed to provide additional insight into prediction errors in noise level from different methods studied. Finally, in Section 5, conclusions are drawn based on deviations observed in computed turbulent inflow noise for each of the methods.
2.1 Moriarty and Migliore Inflow Method
In this method, the turbulent inflow noise is modeled using the empirical relations developed by Lowson [10], and Amiet [11] for airfoils and wind turbine blades. Lowson [10] used experiment airfoil measurements intended to predict the surface pressure fluctuations at the trailing edge of the airfoil and its influence on the leading edge velocity perturbations. From a given acoustic source, the inflow noise predicted by this method depends strongly on boundary layer turbulence intensity, length scale parameters, and scales with sixth power of local Mach number. The expression for 1/3rd octave sound pressure at an observer position can be evaluated using Eq. (1) which includes high-frequency term, low-frequency correction factor, and acoustic transfer function which takes into account the compressibility effects in turbulent flows. The high-frequency term is aimed to predict the inflow noise and contains the von Karman turbulent energy spectra for the velocity field given by Eq. (2).
where M–local Mach number, DL is the low-frequency directivity function, f–1/3rd octave band frequency, Hz, LFC is the low-frequency correction factor term given by Eq. (3), l is the turbulence length scale, L–span segment length, I–Turbulence intensity in %, c–chord length in m, re is the effective distance between the non-stationary source and (microphone) receiver, U–mean wind speed along the rotor axis, u–local flow velocity over the blade airfoil span, S is the acoustic transfer function given by Eq. (4) expressed in terms of β and K. β is Prandtl-Glauert compressibility factor given by Eq. (5) and K–wave number given by Eq. (6). Further, experiment validation studies conducted by Oerlemans [7], Buck et al. [22] on inflow noise from wind turbines revealed that noise prediction can also be done by using simpler Kolmogorov spectra method for turbulence. However, the use of such method is not accurate to predict low-frequency noise at specific frequency regimes and also difficult to validate the predicted results because of the inherent limitations associated in measurement of turbulence energy dissipation that occur at different length scales common for wind turbines.
The term DL, deals with the directional nature of acoustic waves radiated from a source at low frequencies in the prediction of turbulent inflow noise given by Eq. (7). This function is dependent on the two angles, Ф, θ, which can capture moving source position relative to a fixed coordinate system. The position of the noise source is determined using the correction factors in a shifted coordinate system and based on the observer’s position relative to the trailing edge of the airfoil [23]. Also, the trailing edge noise method formulated by Moriarty et al. [5], and Brooks et al. [8] considers both the low and high-frequency directivity functions to predict noise from an acoustic source. Furthermore, trailing edge noise from wind turbine blades shows a cardioid pattern which can be described by high-frequency directivity function as given by Eq. (8) and scales as p2 α M5 where p is the rms pressure. However, for turbulent inflow noise, the acoustic waves are generated near the leading edge of the blade that becomes more significant at low frequencies which are often exhibited as a dipole pattern perpendicular to the chord line and scales as p2 α M6. Thus, to an extent this dipole pattern varies with mean velocity in a flow field. At high Mach numbers, the pressure waves in the boundary layer convert from the surfaces and produce acoustic waves. The amplitude of acoustic pressure waves can rapidly vary in near fields which can be better analyzed by the high-frequency directivity function given by Eq. (8).
where the terms θ and Ф are the directivity angles between the observer and trailing edge of the source in the span wise and chord wise directions. The term
Hubbard et al. [12] derived an empirical relation for turbulent inflow noise based on the Amiet’s experiment measurements of surface pressures on thin airfoils and flat plates in a wind tunnel. This method can predict acoustic fields for a broad range of frequencies by considering turbulence properties that are approximated based on homogeneous isotropic von-Karman turbulence spectra and also by correlating the surface boundary layer velocity measurements with the acoustic field. The turbulent flow properties were characterized based on the rms value of velocity fluctuations, turbulence wave number of energy-containing turbulent eddies, integral length scale, and turbulence intensity. The overall expression for terms in inflow noise prediction is similar to that of the method described in Section 2.1 except for the empirical constant term 58.4 is replaced with a value of 78.4 given in Eq. (2) to arrive at the Eq. (9).
As mentioned earlier, this method also constitutes the low-frequency directivity function and high-frequency corrections for predicting 1/3rd octave sound pressure. The high-frequency correction also involves isotropic von-Karman turbulent velocity spectra to calculate the turbulence velocity fluctuations that act as a source for noise generation. These are coded using empirical constants which overcome the limitations of Amiet’s method related to observer position in the measurement of sound pressure over a frequency range, of 200–2500 Hz in sound spectra. The low-frequency correction involves the spherical directivity and depends on the acoustic transfer function as described in Section 2.1 and is intended to analyze the effect of periodic and random gusts on inflow turbulent noise. To take account of compressibility effects in turbulent flows, it also considers the Prandtl-Glauert compressibility factor expressed in terms of local Mach number, M. As mentioned before DL is the low-frequency directivity function expressed in terms of observer angles in the rotor and azimuthal planes, respectively. The symbols used in Eq. (9) assume the same meaning as given in Eqs. (1) and (2).
In this method, the turbulent inflow noise mechanism from horizontal axis wind turbine (HAWT) blades is predicted based on the theoretical work on fluctuating lift generating force on airfoils and helicopter rotors. The lifting force on HAWT blades is similar to that experienced on helicopter rotor. In the former case, turbulent eddies approach rotor disk at any given mean wind speed, while in latter, they are compressed and elongated alternately at the blade passing frequency in forward flight [6].
As discussed in Section 2.2 the turbulence flow field conditions in this method are also assumed to be homogenous isotropic. From a wind turbine perspective, the longitudinal velocity component is considered significant for estimating turbulence intensity since it captures the turbulent velocity fluctuations and sinusoidal wind gusts appreciably well. For neutral atmospheric stability conditions, the longitudinal turbulence spectrum can be expressed in terms of reference turbulence intensity as given by Eq. (10).
The reference and root mean square turbulence intensity are denoted using wr and
To predict the root mean square sound pressure in the far field from the wind turbine blade, this method uses the expression for acoustic pressure formulated by Curle [24] and given by Eq. (13).
where K1 (f) is the frequency-dependent scaling factor to model the dipole point source located at the hub height of the wind turbine rotor. B is the number of blades, R is the rotor blade radius, U is the free stream velocity, m/s, c is the chord length, r0, c0 are the effective distance between the source (blade segment) and receiver (microphone) and speed of sound, ρ is the air density in kg/m3, φ is the directional projection of azimuth reference angle between source and receiver in rotor plane, p2 is the root mean square pressure, h is the height above ground, Vw is the relative wind velocity on blade, η and η0 are the reduced and reference to reduced order frequency considered at given height above ground. The logarithmic sound pressure is then evaluated using rms sound pressure given by Eq. (14).
where pref is the reference sound pressure for air, ~ 20 μPa.
2.4 Modified Rapid Distortion Theory (RDT)
To understand sudden changes to turbulence characteristics in a flow field, the rapid distortion theory (RDT) method developed by Hunt [25] provides insight into turbulence velocity field and pressure fluctuations in homogeneous isotropic incompressible flows. It essentially uses a wavenumber analysis to calculate the surface pressure fluctuation under homogeneous isotropic turbulence conditions. These perturbations can change rapidly over definite time scales relative to the mean velocity field and can influence the size of eddy formation in a flow field. These eddies contain turbulent kinetic energy that can extract energy from the mean flow field in a cascaded manner which varies with time and eventually gets dissipated. According to Goldstein et al. [26], the rapid distortion theory method takes into account of following assumptions:
• The nominal turbulence intensity in the atmosphere must be weak relative to normalized local turbulence values in a flow field.
• Reynolds number for the impinging turbulent flow and the length scales in turbulent flows must be higher compared to mean flow velocity.
• The time scales for flow distortion to occur must be significantly lower compared to the time scales of local turbulence within the boundary layer and also depend on the characteristic dimensions of the source.
In incompressible flows, the pressure fluctuations in the local flow field characterize the extent of turbulence which significantly affects leading edge noise and varies according to chord Reynolds number. Earlier studies by Moreau et al. [4], dos Santos et al. [27,28] indicated that the flow angle of attack is a critical parameter that affects the boundary layer properties. They conducted experiments and numerical simulations to study the properties of turbulence impingement and its interaction near the leading edge of the airfoil which is responsible for noise production. Further, these studies were conducted for different thin and thick airfoil geometry configurations which include thickness to chord ratio, and camber to predict the far field noise radiation and were based on Amiets method [4,27–29]. As regards the Amiet method, it was inaccurate in predicting the leading edge noise at high frequencies when validated with experiment data because of the small thickness assumption inherent in the method since it disregards the velocity fluctuations for specific wave numbers in turbulence energy spectra. An improved method was developed for the turbulent velocity spectrum which takes account of velocity perturbations at the leading edge of the boundary layer on the airfoils, even at higher wave numbers to predict turbulence interaction noise in a better manner. Consequently, Faria et al. [21] extended this approach and experimented with a flat plate, thin airfoils from the NACA series with sharp trailing edges. The length scale proposed by Hunt [25], and Christophe [30] was adopted to examine its influence on local turbulence properties which led to an improved rapidly distorted turbulent energy spectra, φww for velocity field as given by Eq. (15).
The above Eq. (15) for turbulent velocity energy spectra was substituted in the modified turbulent inflow noise method initially proposed by standard Lowson method for sound pressure given by Eq. (9). The equation for sound pressure derived by Faria et al. [21] produced a change in empirical constant from 78.4 to 89.95 and given by Eq. (16).
kx and ky are the turbulence wave numbers of velocity fluctuations along blade chord and span directions. ke is the turbulence wave number corresponding to energy containing turbulent eddies in velocity spectra.
In the present study, the simulation setup for predicting sound pressure from wind turbine blades consists of two solvers. One flow solver uses the blade element momentum (BEM) method to compute the local relative velocity field along the blade span. The important outputs of the BEM solver include flow angle of attack, induction factors, and local flow velocity as well as aerodynamic loads on individual blade sections. These are coupled to an acoustic solver which uses the acoustic equations of four different turbulent inflow noise methods described in Section 2 as well as from Brooks et al. [8] method for trailing edge noise prediction to compute, sound pressure level at a given wind speed. Fig. 1a demonstrates the flow chart for the simulation setup adopted for predicting aerodynamic noise from wind turbine blades. It must be noted that secondary outputs from the BEM solver can be used to evaluate structural loads on turbine components. Fig. 1b illustrates the input process variables used in semi-empirical methods for predicting the aerodynamic noise. The flow chart also illustrates the dependence of parameters involved in methods for analysing the trailing edge and leading edge noise. The BEM approach considers the entire blade span into a series of aerofoils preferably at least 20 segments and modelled as a distributed point source in the noise simulation. Flow over aerofoils is assumed as 2D incompressible and quasi uniform along the blade span length. The limits for boundary layer parameters viz. boundary layer thickness, displacement thickness was obtained from XFOIL program which includes a wide range of values for flow angle of attack and Reynolds number [31]. They are approximated using interpolation functions on the pressure and suction sides of selected aerofoils, viz. NACA 0012, NACA 6320, and NACA 63215, respectively. The interpolation and amplitude functions are given by using the Eqs. (35) to (50) given in Brooks et al. [8]. Further, to approximate the data computed between each segment along the whole blade span, a linear interpolation technique is used and given from Eqs. (6.25) to (6.27) given in Hansen [32]. This boundary layer data of aerofoils serves as input for the noise solver to evaluate the acoustic equations in each case. In the present simulation work for a 2 MW wind turbine, the flow Reynolds number varies up to 4 million.
Figure 1: (a) Illustration of computational setup implemented for noise prediction (b) Flow chart for input process variables used in semi-empirical methods for predicting the leading edge and trailing edge noise
Fig. 2a shows the illustration of the aerodynamic self-noise mechanism from wind turbine blades and turbulent inflow noise perceived by an observer in a far field. The sound pressure level is thus calculated by logarithmic addition of individual sources iteratively relative to the observer position. The receiver height was assumed to be fixed at ~1.5 m and the source height at 80 m above ground. The distance of the receiver location was set at 120 m, which is approximately the total turbine height (HH + D/2). The noise calculation assumes a tower diameter of 4 m and blade span length of ~38 m with a maximum chord length and blade twist of ~3.2 m and 12°. According to IEC 61400-11 regulations for measurements of acoustic emissions from wind turbines, this criterion is important for consistent measurement of sound pressure level from a given source at any given hub height (HH) of turbine and rotor diameter, D. Acoustic pressure waves that radiate from a source are likely to propagate in farther distances and subjected to attenuation. However, for assessing noise in the near field, the geometric divergence is more important and has been considered in the analysis. The simulations for turbulent inflow and trailing edge noise were implemented in MATLAB R2022b software.
Figure 2: (a) Sketch of airfoil self-noise and turbulent inflow mechanisms from a wind turbine rotor (b) Illustration of microphone (downwind) position surrounding the source located in the center as well as the microphone measurement distances and position according to IEC 61400-11 standards concerning the source
According to Moriarty et al. [5], Oerlemans [7], Amiet [11], van den Berg [14], peak noise levels are recorded when the microphone receiver is in downwind of the rotor. For the downwind of the rotor plane, the receiver location appears as shown in Fig. 2b where maximum noise radiation occurs during the downward direction of blade movement. Hence this condition is taken into account in the present study. The dynamic parameters for the turbine such as the rotor rotations per minute (RPM) are set at 17 RPM. To compute the standard error for sound power predicted from methods, noise data is generated using 5 iterative simulations each at different turbulence intensities keeping other factors constant. Further, in validation of the simulated results, the experiment noise data for SWT-2.3MW 93 m turbine has been considered. Even though A-weighting and equivalent scales are used for measuring outdoor noise levels from wind turbines, however, for leading edge noise direct noise measurements without a weighting filter can be considered. In the present study, measured data in un-weighted form was taken to validate the computed noise data.
For wind turbines, low-frequency broadband noise is an important source that occurs from the trailing edge of the blade surface and is often perceived as a “swish” phenomenon. One of the reasons for this phenomenon is attributed to the edge scattering of pressure waves and subsequent amplitude modulation at blade passing frequencies in the far field. The interaction of turbulent flows over the trailing edge of the blade surface can generate noise very well which becomes dominant in high frequencies. On the other hand, turbulent inflow noise results when pressure waves within the boundary layer undergo scattering in response to the turbulent inflows that move past the leading edge of rotating blades. For downwind turbines, the turbulent inflows can also produce an impulse type of noise which is often heard as a loud “thump” in the far field and caused due to transient aerodynamic blade loading in the rotor plane. The impulsive sound is cyclical and can be attributed to blade, and tower-wake interactions as well as inflow velocity gradients in the rotor plane. However, in this section, we discuss the computational results obtained from the quasi-empirical methods developed for analyzing turbulent inflow noise mechanisms as described in Section 2.
To assess sound power produced by the candidate turbine used in the study all the inflow noise methods were simulated at a wind speed of 12 m/s and turbulence intensity of 10%. These values for turbulence intensity and wind speed lie within a moderate range which is typically experienced for common wind turbine applications. It can be observed from Fig. 3 that the sound power, Lw as predicted using Grosveld method was found to reach a peak value of 112 dB at low frequencies, f below 100 Hz and continued to decrease with increasing frequencies. The Lw values agreed closely with Moriarty et al. [5], standard Lowson and modified rapid distortion theory (RDT) methods that vary within 4 dB. The slope of the line for sound power predicted by Grosveld method also showed a linear downward trend at all frequencies in the spectra. It must be noted that for frequencies, f above 100 Hz the acoustic power predicted from the Grosveld method showed a constant difference of 4–5 dB relative to Moriatty & Migliore, Lowson methods and significant difference up to 20 dB concerning modified rapid distortion theory. Also, the sound power predicted by the modified rapid distortion theory (RDT) method agreed much better with experiment data of turbine, i.e., Siemens SWT-2.3MW 93 in the low-frequency region. This shows that the modified RDT method does not overestimate the inflow noise, unlike the remaining methods which tend to predict the sound power values higher by ~15 to 20 dB in the mid-band to high frequency region. To some extent, this disagreement is caused because the turbulent velocity spectra for Moriarty & Migliore and modified RDT methods were based on the standard Lowson method that was not implemented in the Grosveld method. The experiment noise data shows a jump near f~1 kHz which is caused due to the trailing edge noise from the blade. This can be explained well in Fig. 4 which demonstrates the combined turbulent inflow noise and trailing edge noise computed for the 2 MW turbine model in the present study. The trailing edge noise was computed using the Brooks et al. [8] method which was originally developed for predicting the self-noise from airfoils. For the 2 MW wind turbine model, the trailing edge noise was computed by using the interpolation functions explained by Eqs. (35) to (50) given in Brooks et al. [8] as mentioned in Section 3.
Figure 3: Comparison of the turbulent inflow noise predicted from the Grosveld, Moriarty, Lowson, and modified rapid distortion theory (RDT) methods at a mean wind speed of 12 m/s at turbulence intensity of 10% and validated with experiment data of Siemens SWT-2.3MW 93 model operating at 14.5 RPM and available from [33]
Figure 4: Combined sound power level from turbulent inflow and trailing edge noise (Brooks et al. [8]) computed for turbulence intensities of 6%, 8 %, 10%, and 12% at a mean wind speed of 10 m/s
It is apparent from Fig. 4 that turbulent inflow noise shows dominance in the low-frequency region of the spectra, 20 Hz < f < 250 Hz for all values of turbulence intensities. However, with an increase in frequency, the sound power continued to increase and showed two broadband humps initially near f~800 Hz and later at f~1 kHz. As mentioned earlier, turbulence intensity has a significant influence on leading edge noise production from wind turbine blades. Hence, this effect was studied by varying the turbulent intensity for a range of 6% to 14%. As wind turbine blades are composed of airfoils with varying chord lengths and twist angles, the noise radiated from the suction and pressure sides of airfoils vary according to flow conditions. The acoustic equations in Brooks et al. [8] method for predicting sound pressure are modeled using spectral shape functions for pressure and suction sides of airfoils. To a large extent, the properties of these shape functions for a given 1/3rd octave frequency are dependent on the flow angle of attack, local flow velocity, and boundary layer properties, e.g., boundary layer displacement thickness and flow Reynolds number. For a given flow angle of attack, the boundary layer thickness continuously grows in thickness along the chord line, and for high or stall angles of attack, the boundary layer separates along its chord line. The boundary layer flow separation can occur from both the suction and pressure sides of the trailing edge and depend strongly on the flow angle of attack. Complete boundary layer flow separation usually occurs from the suction side for large positive angles of attack but for negative angles of attack, the flow can also separate from the pressure side of the airfoil and under these conditions the noise radiation is depicted as closely spaced humps near mid band to high frequencies in noise spectra. Further from Fig. 4 at turbulence intensity of 6%, the noise level varied from 100 dB at f~20 Hz and reached 93 dB for f~200 Hz, however for turbulence intensity values greater than 10% it was found to vary up to 106, and 97 dB, respectively. This shows a change of ~9 to 10 dB that can be observed in the low-frequency region while in the mid band to high frequencies the turbulent boundary layer trailing edge noise (TBL-TEN) dominates over turbulent inflow noise. Further, the trailing edge noise source shows the same pattern in sound spectra with insignificant changes in sound power values for high frequencies, i.e., frequencies between f > 1 kHz and f < 4 kHz, regardless of change in turbulent intensity levels. However, for f > 4 kHz the trailing edge noise still shows considerable change in its amplitude with an increase in turbulence intensity. Hence, for subsonic turbulent flows, low-frequency broadband noise is an important phenomenon from wind turbine blades that are affected by boundary layer turbulence properties and turbulence velocity spectra characteristics [34,35].
Fig. 5 demonstrates the change in sound pressure with measured turbulence intensity. The experiment data points for sound pressure at different turbulent intensity values viz. between 10% and 25% have been obtained from Buck et al. [36] and show an exponentially increasing trend with a change of 10 dB. The turbulence intensity values measured in their experiment campaign were obtained at a wind farm location to check the influence of turbulence intensity on the measured sound pressure and to validate the experiment noise data from wind turbines that do not exceed the local emissions limits. It must be noted that the turbulence intensity values measured during day or night time, will also influence the measured sound pressure. According to Pedersen et al. [2], Amiet et al. [11], van den Berg [14], Makarewicz et al. [19], Bowdler [20] measured apparent sound pressure level is strongly influenced by local turbulence intensity and the noise exposure perceived by inhabitants near a wind farm are highly subjective. During day time, the perceived noise limits ranged between 35–40 dB and background noises often masked the source noise radiation from wind farms, however, during evening and night times, the apparent sound pressure levels were found higher by 5–10 dB compared to day time limits. To a large extent, this increase can be attributed to temperature gradients in the atmosphere which affect the velocity profiles in the boundary layer and subsequently influence the turbulence intensity during day, evening, and night times. However, uncertainties in sound pressure can also be caused by the wind flow-induced noise measured by microphones which can increase the amplitude of low-frequency noise. Furthermore, in the present study, a logarithmic distribution for data was used for fitting the measured turbulence intensity data points given by Eq. (17) to estimate sound pressure level and involves two coefficients A and B.
Figure 5: Comparison of log-fitted sound pressure as a function of turbulence intensity computed using Eq. (17) with experiment data from Buck et al. [36]
where A, and B are constants defined as 6 and 8 for the present study and TI is the turbulence intensity. It must be noted that the sound pressure varies with measured turbulence intensity in relation p2 α TI2 which implies a ~6 dB increase in turbulent inflow noise when turbulence intensity is doubled. However, it must be noted that deviations between measured and log-fitted sound pressure levels could be possibly caused due to spatial sampling errors in the measurement of turbulence intensity and influence the sound pressure level. Thus, the results of the present data fit agreed well with experiment noise data conducted as part of survey research studies on perceived noise.
It is well known that to assess the uncertainties of turbulence properties, advanced experimental methods such as hot wire anemometry (HWA), particle image velocimetry (PIV), and laser Doppler velocimetry measurement techniques (LDV) can be used for flow field measurements which can measure velocity perturbations more accurately. However, simulating unsteady turbulent flows at different time scales and acoustic fields using computational fluid dynamics (CFD) based methods such as URANS, DNS or LES is a complex task which requires high computational cost and hence has not been considered in this study [37–40].
For wind turbine noise prediction the effect of flow angle of attack can significantly influence the extent of boundary layer flow separation on blades and consequently the boundary layer properties, e.g., thickness, displacement thickness values. At low to moderate flow angles of attack, the boundary layer flow will remain attached along the chord line of the blade and produce lesser turbulence for which the noise radiation occurs predominantly closer to the trailing edge. However, at very high or stall angles of attack, airfoils experience a dynamic stall phenomenon for which the boundary layer separates completely along the chord line of the blade surface due to adverse pressure gradients within the boundary layer. This phenomenon tends to increase the trailing edge noise up to 15 dB at mid band frequencies. It can be noted that for leading edge noise the incoming turbulence intensity seen by blades is more significant than other parameters of the inflow noise methods described earlier. Also, length scales can give information about the formation and size of incoming eddies in a flow field. These eddies contain varying levels of turbulent kinetic energy which ultimately undergoes viscous dissipation at smaller length scales in flow field. Thus, the impact of turbulence properties is essential and through empirical formulations it can readily be used to estimate the inflow of turbulent noise from wind turbine blades.
Fig. 6a demonstrates the normal turbulence intensity (TI) at different mean wind speeds using IEC 61400-1 design code for three reference turbulence classes, Class A, Class B, and Class C computed using Eq. (11) given in [41]. At a given height above ground, the wind conditions usually include mean flow velocity combined with fluctuating velocity components that is responsible for turbulence characteristics at a site. As mentioned earlier, the representative normal turbulence values for three reference classes at a given site according to the IEC 61400-1 design code are equivalent to 16%, 14%, and 12%, respectively. It can be seen that with an increase in mean wind speeds up to 10 m/s, the turbulence intensity reduces exponentially but they reach steady values at high wind speeds. Similarly, Fig. 6b shows the standard deviation of turbulent velocity obtained for the same three reference turbulence classes using Eq. (11) given in [41]. In contrast to turbulence intensity, the standard deviation of turbulent velocity increased linearly with an increase in mean wind speed for all reference site turbulence classes.
Figure 6: (a) Comparison of IEC turbulence classes for different mean wind speeds at normal turbulence levels (NTM) (b) Representative standard deviation of longitudinal turbulent wind speed component, for three IEC turbulence classes at different mean wind speeds (m/s)
The sound power, Lw for turbulent inflow noise computed by all four methods shows the relative difference in the low-frequency region of spectra and at different turbulent intensities. For this reason, the standard error for sound power, Lw has been evaluated. Table 1 shows that the standard error for Lw in the frequency range, 20 Hz < f < 315 Hz is reduced with increasing values of turbulence intensity at a mean wind speed of 10 m/s. This suggests that all four methods predict similar error trends. Also, for a given turbulence intensity, with an increase in frequency, the standard error for noise decreased by at least 0.5 dB up to f~80 Hz after which the error increased continuously and varied by ~2 dB. The maximum standard error of 3 dB was found at f~315 Hz at a low turbulence intensity of 6%. This can be caused due to uncertainties in model input parameters which have a significant effect on turbulent inflow noise at low frequencies. However, at high frequencies, these model uncertainties diminish and have a negligible effect on the leading edge noise. As mentioned earlier, the sound pressure level is affected by mean wind speed at hub height and turbulence intensity hence a contour plot that depicts the change in sound pressure is also illustrated in Fig. 7. It can be seen from Fig. 7 that with an increase in mean wind speed and turbulence intensity, the sound pressure is found to increase in a nonlinear manner and ranges between 50 to ~70 dB.
Figure 7: Contour plot of total sound pressure level computed for different mean wind speeds and turbulence intensities
Hence, to summarize, the turbulent inflow noise contributes significantly to the aerodynamic noise signature of wind turbines and is affected by mean flow velocity, and turbulence velocity energy spectra as well as the turbulence intensity factors.
The present study investigated the effects of turbulence intensity, and mean wind speed on turbulent inflow noise from wind turbine blades using four different semi-empirical methods. With increasing values of turbulence intensity and mean wind speed, the aerodynamic noise was found to increase in the low-frequency part of noise spectra and the predicted values from each method exhibited good agreement with experiment data. According to various methods, it has been observed that leading edge noise decreases linearly with different slopes for increasing frequencies. The noise remains dominant in the low-frequency region of the noise spectra, regardless of any changes in mean wind speed and turbulence intensity. The comparison for turbulent inflow noise data computed using each method also shows that the maximum standard error for sound power is ~3 dB found at 315 Hz in sound spectra for a turbulence intensity of 6%. The modified rapid distortion turbulence method predicted the sound power more accurately in low-frequency regions where the leading edge noise is dominant but showed a disagreement of ~20 dB compared to the remaining methods for mid-band to high frequencies. Thus, the prediction of the leading edge noise from wind turbine blades is highly dependent on turbulence intensity, and turbulent velocity energy spectra characteristics considered for each method.
Acknowledgement: Authors would like to thank GITAM (Deemed to be University) for providing the technical facilities and support for this work.
Funding Statement: The authors received no specific funding for this study.
Author Contributions: The authors confirm contribution to the paper as follows: study conception and design: Vasishta Bhargava Nukala, Chinmaya Prasad Padhy; data collection: Vasishta Bhargava Nukala; analysis and interpretation of results: Vasishta Bhargava Nukala, Chinmaya Prasad Padhy; draft manuscript preparation: Vasishta Bhargava Nukalam, Chinmaya Prasad Padhy. All authors reviewed the results and approved the final version of the manuscript.
Availability of Data and Materials: Available upon request.
Conflicts of Interest: The authors declare that they have no conflicts of interest to report regarding the present study.
References
1. Doolan, C. (2011). Wind turbine noise mechanisms and some concepts for its control. Proceedings of Acoustics, Gold Coast, Australia. [Google Scholar]
2. Pedersen, E., van den Berg, F., Bakker, R., Bouma, J. (2009). Response to noise from modern wind farms in the Netherlands. Journal of Acoustical Society of America, 126(2), 634–643. https://doi.org/10.1121/1.3160293 [Google Scholar] [PubMed] [CrossRef]
3. Fredianelli, L., Stefano, C., Gaetano, L. (2019). A procedure for deriving wind turbine noise limits by taking into account annoyance. Science of the Total Environment, 648, 728–736. https://doi.org/10.1016/j.scitotenv.2018.08.107 [Google Scholar] [PubMed] [CrossRef]
4. Moreau, S., Roger, M. (2005). Effect of angle of attack and airfoil shape on turbulence-interaction noise. 11th AIAA/CEAS Aeroacoustics Conference, Monterey, California, USA. https://doi.org/10.1121/10.0013703 [Google Scholar] [PubMed] [CrossRef]
5. Moriarty, P., Migliore, P. (2003). Semi–empirical noise prediction code for wind turbines. NREL Report, TP-500-34478. [Google Scholar]
6. Grosveld, W. F. (1984). Prediction of broadband noise from horizontal axis wind turbines. AIAA/NASA 9th Aeroacoustics Conference, vol. 1, no. 4, pp. 292–299. Williamsberg, USA. https://doi.org/10.2514/3.22796 [Google Scholar] [CrossRef]
7. Oerlemans, S. (2009). Detection of aero-acoustic sound sources on aircrafts and wind turbines (Ph.D. Thesis). University of Twente, Enschede, Netherlands. [Google Scholar]
8. Brooks, T. F., Pope, D. S., Marcolini, M. A. (1989). Airfoil self noise and prediction. https://ntrs.nasa.gov/archive/nasa/casi.ntrs.nasa.gov/19890016302.pdf (accessed on 10/08/2016). [Google Scholar]
9. Lowson, M. V. (1970). Theoretical analysis of compressor noise. Journal of Acoustical Society of America, 47(1), 371–385. https://doi.org/10.1121/1.1911508 [Google Scholar] [CrossRef]
10. Lowson, M. V. (1992). Assessment and prediction of wind turbine noise. Flow Solutions Report 92/19. STSU W/13/00284/REP. Bristol, England. [Google Scholar]
11. Amiet, R. K. (1976). Noise due to turbulent flow past a trailing edge. Journal of Sound and Vibration, 47(3), 387–393. https://doi.org/10.1016/0022-460X(76)90948-2 [Google Scholar] [CrossRef]
12. Hubbard, H. H., Shephard, K. P. (1991). Aeroacoustics of large wind turbines. Journal of Acoustical Society of America, 89(6), 2495–2508. https://doi.org/10.1121/1.401021 [Google Scholar] [CrossRef]
13. Kim, H., Lee, S. (2012). Aerodynamic noise analysis of large horizontal axis wind turbines considering fluid structure interaction. Renewable Energy, 42, 46–53. https://doi.org/10.1016/j.renene.2011.09.019 [Google Scholar] [CrossRef]
14. van den Berg (2006). The sound of high winds: The effect of atmospheric stability on wind turbine sound and microphone noise (Doctoral Thesis). University of Groningen, Netherlands. [Google Scholar]
15. Kerscher, M., Vonrhein, B., Ueberle, F., Rokita, D. (2016). How acoustic camera measurements can help to increase the acceptance of wind turbines. Wind Europe Summit, Hamburg, Germany. [Google Scholar]
16. Butt, A. H., Akbar, B., Aslam, J., Akram, N., Soudagar, M. E. M. et al. (2020). Development of a linear acoustic array for aero-acoustic quantification of camber-bladed vertical axis wind turbine. Sensors, 20(20), 5954. [Google Scholar] [PubMed]
17. Kümmritz, S., Ali, M., Johannes, P. (2020). Development of a standard approach for wind turbine measurements with an acoustic camera for optimization purposes. Forum Acusticum. https://doi.org/10.48465/fa.2020.0524 [Google Scholar] [CrossRef]
18. Licitra, G., Francesco, A., Bernardini, M., Antonio, M., Francesco, F. et al. (2023). Acoustic beamforming algorithms and their applications in environmental noise. Current Pollution Reports, 9, 486–509. [Google Scholar]
19. Makarewicz, R., Golebiewiski, R. (2018). Amplitude modulation of wind turbine noise. SJ Journal of Aviation and Aeronautical Science, 1, 1–4. https://scienceforecastoa.com/Articles/SJASS-V1-E1-1008.pdf (accessed on 02/12/2020). [Google Scholar]
20. Bowdler, D. (2008). Amplitude modulation of wind turbine noise: A review of evidence. Acoustics Bulletin, 33(4). https://docs.wind-watch.org/bowdler–amofwindturbines.pdf (accessed on 10/04/2018). [Google Scholar]
21. Faria, M. A., Saab, J. Y., Rodriguez, S., Pimenta, M. M. (2020). A rapid distortion theory based airfoil turbulent inflow noise prediction method. Journal of Brazilian Society of Mechanical Sciences and Engineering, 42, 397. https://doi.org/10.1007/s40430-020-02468-2 [Google Scholar] [CrossRef]
22. Buck, S., Oerlemans, S., Palo, S. (2018). Experiment validation of a wind turbine turbulent inflow noise prediction code. 22nd AIAA/CEAS Aeroacoustics Conference, Lyon, France. https://doi.org/10.2514/1.J056134 [Google Scholar] [CrossRef]
23. Friman, M. (2011). Directivity of sound from wind turbines, A study of horizontal radiation pattern from wind turbine (Master Thesis). Department of Aeronautical & Vehicle Engineering, KTH, Stockholm, Sweden. [Google Scholar]
24. Curle, N. (1955). The influence of solid boundaries upon aerodynamic sound. Proceedings of the Royal Society of London, 231, 505–514. https://doi.org/10.1098/rspa.1955.0191 [Google Scholar] [CrossRef]
25. Hunt, J. C. (1973). A theory of turbulent flow round two-dimensional bluff bodies. Journal of Fluid Mechanics, 61(4), 625–706. https://doi.org/10.1017/S0022112073000893 [Google Scholar] [CrossRef]
26. Goldstein, M. E., Afsar, M. Z., Leib, S. J. (2013). Nonhomogeneous rapid distortion theory on transversely sheared mean flows. Journal of Fluid Mechanics, 736, 532–569. https://doi.org/10.1017/jfm.2013.518 [Google Scholar] [CrossRef]
27. dos Santos, F. L., Botero-Bolivar, L., Venner, C., de Santana, L. D. (2022). Modelling the turbulence spectrum dissipation range for leading edge noise prediction. AIAAJ, 60(6). https://doi.org/10.2514/1.J061106 [Google Scholar] [CrossRef]
28. dos Santos, F. L., Botero-Bolivar, L., Venner, C., de Santana, L. D. (2022). Inflow turbulence distortion for aerofoil leading edge noise prediction for large turbulence length scales for zero-mean loading. The Journal of the Acoustical Society of America, 153(3), 1811–1822. https://doi.org/10.1121/10.0017458 [Google Scholar] [PubMed] [CrossRef]
29. Amiet, R. (1975). Acoustic radiation from an airfoil in turbulent stream. Journal of Sound and Vibration, 41(4), 407–420. https://doi.org/10.1016/S0022-460X(75)80105-2 [Google Scholar] [CrossRef]
30. Christophe, J. (2011). Application of hybrid methods to high frequency aero-acoustics (Ph.D. Thesis). The von Karman Institute for Fluid Dynamics. [Google Scholar]
31. XFOIL. MIT Press. https://web.mit.edu/drela/Public/web/xfoil/ (accessed on 23/04/2023). [Google Scholar]
32. Hansen, M. O. L. (2010). Aerodynamics of wind turbines, 2nd edition. London, UK: Earthscan publishers. [Google Scholar]
33. Siemens gamesa renewable energy. https://www.siemensgamesa.com (accessed on 23/04/2023). [Google Scholar]
34. Bhargava, V., Samala, R. (2019). Acoustic emissions from wind turbine blades. Journal of Aerospace Technology and Management, 11. https://doi.org/10.5028/jatm.v11.1071 [Google Scholar] [CrossRef]
35. Bhargava, V., Samala, R., Anumula, C. (2019). Prediction of broadband noise from symmetric and cambered airfoils. INCAS Bulletin, 11(1). https://doi.org/10.13111/2066-8201.2019.11.1.3 [Google Scholar] [CrossRef]
36. Buck, S., Oerlemans, S. (2016). Experiment characterization of turbulent inflow noise on a full scale wind turbine. Journal of Sound and Vibration, 385(4), 219–238. https://doi.org/10.1016/j.jsv.2016.09.010 [Google Scholar] [CrossRef]
37. Ferziger, J. H., Peric, M. (2004). Computational methods for fluid dynamics, 3rd edition. Nurenberg, Germany: Springer Publishers. [Google Scholar]
38. Worsnop, R., Bryan, G. H., Lundquist, J. K., Zhang, J. A. (2017). Using large eddy simulations to define spectral and coherence characteristics of the hurricane boundary layer for wind energy applications. Journal of Boundary Layer Meteorology, 165, 55–86. https://doi.org/10.1007/s10546-017-0266-x [Google Scholar] [CrossRef]
39. Han, X. X., Liu, D. Y., Xu, C., Shen, W. Z., Li, L. M. et al. (2019). Monin-Obukhov similarity theory for modeling of wind turbine wakes under atmospheric stable conditions: Breakdown and modifications. Journal of Applied Sciences, 9(20), 4256. https://doi.org/10.3390/app9204256 [Google Scholar] [CrossRef]
40. Lungu, D., Pieter, V. G. (2014). Characteristics of wind turbulence with applications to wind codes (Master Thesis). Technical University Bucharest, Romania, Delft University of Technology, Netherlands. [Google Scholar]
41. IEC 61400-1, Wind Turbines, Part–1 Design requirements, 3rd edition. International Electro-Technical Publications. https://webstore.iec.ch/ (accessed on 01/05/2023). [Google Scholar]
Cite This Article
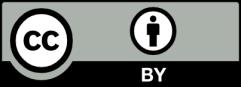