Open Access
ARTICLE
A Novel Interacting Multiple-Model Method and Its Application to Moisture Content Prediction of ASP Flooding
Automation School, Beijing University of Posts and Telecommunications, Beijing, China.
College of Information and Control Engineering, China University of Petroleum (East China), Qingdao, China.
*Corresponding author: Shurong Li. Email:
Computer Modeling in Engineering & Sciences 2018, 114(1), 95-116. https://doi.org/10.3970/cmes.2018.114.095
Abstract
In this paper, an interacting multiple-model (IMM) method based on data-driven identification model is proposed for the prediction of nonlinear dynamic systems. Firstly, two basic models are selected as combination components due to their proved effectiveness. One is Gaussian process (GP) model, which can provide the predictive variance of the predicted output and only has several optimizing parameters. The other is regularized extreme learning machine (RELM) model, which can improve the over-fitting problem resulted by empirical risk minimization principle and enhances the overall generalization performance. Then both of the models are updated continually using meaningful new data selected by data selection methods. Furthermore, recursive methods are employed in the two models to reduce the computational burden caused by continuous renewal. Finally, the two models are combined in IMM algorithm to realize the hybrid prediction, which can avoid the error accumulation in the single-model prediction. In order to verify the performance, the proposed method is applied to the prediction of moisture content of alkali-surfactant-polymer (ASP) flooding. The simulation results show that the proposed model can match the process very well. And IMM algorithm can outperform its components and provide a nice improvement in accuracy and robustness.Keywords
Cite This Article
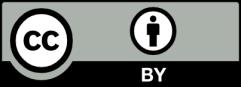