Open Access
ARTICLE
Data Augmentation Technology Driven By Image Style Transfer in Self-Driving Car Based on End-to-End Learning
1 School of Mechanical Engineering, Guizhou University, Guiyang, 550025, China.
2 Faculty of electronic and information engineering, Xi’an Jiaotong University, Xi’an, 710049, China.
* Corresponding Author: Jin Zhao. Email: .
Computer Modeling in Engineering & Sciences 2020, 122(2), 593-617. https://doi.org/10.32604/cmes.2020.08641
Received 18 September 2019; Accepted 09 December 2019; Issue published 09 February 2020
Abstract
With the advent of deep learning, self-driving schemes based on deep learning are becoming more and more popular. Robust perception-action models should learn from data with different scenarios and real behaviors, while current end-to-end model learning is generally limited to training of massive data, innovation of deep network architecture, and learning in-situ model in a simulation environment. Therefore, we introduce a new image style transfer method into data augmentation, and improve the diversity of limited data by changing the texture, contrast ratio and color of the image, and then it is extended to the scenarios that the model has been unobserved before. Inspired by rapid style transfer and artistic style neural algorithms, we propose an arbitrary style generation network architecture, including style transfer network, style learning network, style loss network and multivariate Gaussian distribution function. The style embedding vector is randomly sampled from the multivariate Gaussian distribution and linearly interpolated with the embedded vector predicted by the input image on the style learning network, which provides a set of normalization constants for the style transfer network, and finally realizes the diversity of the image style. In order to verify the effectiveness of the method, image classification and simulation experiments were performed separately. Finally, we built a small-sized smart car experiment platform, and apply the data augmentation technology based on image style transfer drive to the experiment of automatic driving for the first time. The experimental results show that: (1) The proposed scheme can improve the prediction accuracy of the end-to-end model and reduce the model’s error accumulation; (2) the method based on image style transfer provides a new scheme for data augmentation technology, and also provides a solution for the high cost that many deep models rely heavily on a large number of label data.Keywords
Cite This Article
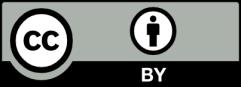