Open Access
ARTICLE
Alcoholism Detection by Wavelet Energy Entropy and Linear Regression Classifier
1 School of Electronic Science and Engineering, Nanjing University, Nanjing, 210093, China
2 Department of Electrical Engineering, College of Engineering, Zhejiang Normal University, Jinhua, 321004, China
3 Educational Information Center, Liming Vocational University, Quanzhou, 362000, China
* Corresponding Author: Yan Yan. Email:
(This article belongs to the Special Issue: Recent Advances on Deep Learning for Medical Signal Analysis (RADLMSA))
Computer Modeling in Engineering & Sciences 2021, 127(1), 325-343. https://doi.org/10.32604/cmes.2021.014489
Received 01 October 2020; Accepted 08 January 2021; Issue published 30 March 2021
Abstract
Alcoholism is an unhealthy lifestyle associated with alcohol dependence. Not only does drinking for a long time leads to poor mental health and loss of self-control, but alcohol seeps into the bloodstream and shortens the lifespan of the body’s internal organs. Alcoholics often think of alcohol as an everyday drink and see it as a way to reduce stress in their lives because they cannot see the damage in their bodies and they believe it does not affect their physical health. As their drinking increases, they become dependent on alcohol and it affects their daily lives. Therefore, it is important to recognize the dangers of alcohol abuse and to stop drinking as soon as possible. To assist physicians in the diagnosis of patients with alcoholism, we provide a novel alcohol detection system by extracting image features of wavelet energy entropy from magnetic resonance imaging (MRI) combined with a linear regression classifier. Compared with the latest method, the 10-fold cross-validation experiment showed excellent results, including sensitivity , specificity , Precision , accuracy , F1 score and MCC .Keywords
Cite This Article
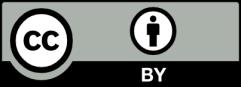