Open Access
ARTICLE
Deep-Learning-Based Production Decline Curve Analysis in the Gas Reservoir through Sequence Learning Models
1 State Key Laboratory of Shale Oil and Gas Enrichment Mechanisms and Effective Development, Beijing, 100083, China
2 Sinopec Petroleum Exploration and Production Research Institute, Beijing, 100083, China
3 Department of Oil-Gas Field Development Engineering, College of Petroleum Engineering, China University of Petroleum, Beijing,
102249, China
* Corresponding Author: Liang Xue. Email:
(This article belongs to the Special Issue: Modeling of Fluids Flow in Unconventional Reservoirs)
Computer Modeling in Engineering & Sciences 2022, 131(3), 1579-1599. https://doi.org/10.32604/cmes.2022.019435
Received 24 September 2021; Accepted 24 November 2021; Issue published 19 April 2022
Abstract
Production performance prediction of tight gas reservoirs is crucial to the estimation of ultimate recovery, which has an important impact on gas field development planning and economic evaluation. Owing to the model’s simplicity, the decline curve analysis method has been widely used to predict production performance. The advancement of deep-learning methods provides an intelligent way of analyzing production performance in tight gas reservoirs. In this paper, a sequence learning method to improve the accuracy and efficiency of tight gas production forecasting is proposed. The sequence learning methods used in production performance analysis herein include the recurrent neural network (RNN), long short-term memory (LSTM) neural network, and gated recurrent unit (GRU) neural network, and their performance in the tight gas reservoir production prediction is investigated and compared. To further improve the performance of the sequence learning method, the hyperparameters in the sequence learning methods are optimized through a particle swarm optimization algorithm, which can greatly simplify the optimization process of the neural network model in an automated manner. Results show that the optimized GRU and RNN models have more compact neural network structures than the LSTM model and that the GRU is more efficiently trained. The predictive performance of LSTM and GRU is similar, and both are better than the RNN and the decline curve analysis model and thus can be used to predict tight gas production.Keywords
Cite This Article
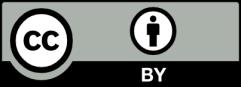