Open Access
ARTICLE
Novel Time Series Bagging Based Hybrid Models for Predicting Historical Water Levels in the Mekong Delta Region, Vietnam
1
Institute of Geography, Vietnam Academy of Science and Technology, Hanoi, 10000, Viet Nam
2
Department of Civil, Environmental and Natural Resources Engineering, Lulea University of Technology, Lulea, 971 87, Sweden
3
Institute of Geological Sciences, Vietnam Academy of Science and Technology (VAST), Dong Da, 10000, Hanoi, Viet Nam
4
University of Transport Technology, Thanh Xuan, Ha Noi, 10000, Viet Nam
5
Department of Watershed & Arid Zone Management, Gorgan University of Agricultural Sciences & Natural Resources, Gorgan,
4918943464, Iran
6
DDG (R) Geological Survey of India, Gandhinagar, 382010, India
* Corresponding Authors: Nadhir Al-Ansari. Email: ; Binh Thai Pham. Email:
(This article belongs to the Special Issue: Soft Computing Techniques in Materials Science and Engineering)
Computer Modeling in Engineering & Sciences 2022, 131(3), 1431-1449. https://doi.org/10.32604/cmes.2022.018699
Received 11 August 2021; Accepted 27 September 2021; Issue published 19 April 2022
Abstract
Water level predictions in the river, lake and delta play an important role in flood management. Every year Mekong River delta of Vietnam is experiencing flood due to heavy monsoon rains and high tides. Land subsidence may also aggravate flooding problems in this area. Therefore, accurate predictions of water levels in this region are very important to forewarn the people and authorities for taking timely adequate remedial measures to prevent losses of life and property. There are so many methods available to predict the water levels based on historical data but nowadays Machine Learning (ML) methods are considered the best tool for accurate prediction. In this study, we have used surface water level data of 18 water level measurement stations of the Mekong River delta from 2000 to 2018 to build novel time-series Bagging based hybrid ML models namely: Bagging (RF), Bagging (SOM) and Bagging (M5P) to predict historical water levels in the study area. Performances of the Bagging-based hybrid models were compared with Reduced Error Pruning Trees (REPT), which is a benchmark ML model. The data of 19 years period was divided into 70:30 ratio for the modeling. The data of the period 1/2000 to 5/2013 (which is about 70% of total data) was used for the training and for the period 5/2013 to 12/2018 (which is about 30% of total data) was used for testing (validating) the models. Performance of the models was evaluated using standard statistical measures: Coefficient of Determination (R2), Root Mean Square Error (RMSE) and Mean Absolute Error (MAE). Results show that the performance of all the developed models is good (R2 > 0.9) for the prediction of water levels in the study area. However, the Bagging-based hybrid models are slightly better than another model such as REPT. Thus, these Bagging-based hybrid time series models can be used for predicting water levels at Mekong data.Keywords
Cite This Article
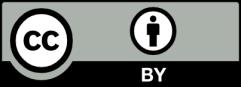