Open Access
ARTICLE
Fault Detection and Identification Using Deep Learning Algorithms in Induction Motors
1
Department of Electronic Engineering, Quaid-e-Awam University of Engineering Science and Technology, Nawabshah, 67480,
Pakistan
2
NCRA Condition Monitoring Systems Lab, Mehran University of Engineering and Technology, Jamshoro, 76062, Pakistan
3
Department of Electronic Engineering, Mehran University of Engineering and Technology, Jamshoro, 76062, Pakistan
4
Design and Creative Technology, Torrens University Australia, Melbourne, Australia
5
Department of Electronics and Power Engineering, Pakistan Navy Engineering College NUST, Karachi, 75350, Pakistan
* Corresponding Author: Majid Hussain. Email:
(This article belongs to the Special Issue: Failure Detection Algorithms, Methods and Models for Industrial Environments)
Computer Modeling in Engineering & Sciences 2022, 133(2), 435-470. https://doi.org/10.32604/cmes.2022.020583
Received 01 December 2021; Accepted 24 February 2022; Issue published 21 July 2022
Abstract
Owing to the 4.0 industrial revolution condition monitoring maintenance is widely accepted as a useful approach to avoiding plant disturbances and shutdown. Recently, Motor Current Signature Analysis (MCSA) is widely reported as a condition monitoring technique in the detection and identification of individual and multiple Induction Motor (IM) faults. However, checking the fault detection and classification with deep learning models and its comparison among themselves or conventional approaches is rarely reported in the literature. Therefore, in this work, we present the detection and identification of induction motor faults with MCSA and three Deep Learning (DL) models namely MLP, LSTM, and 1D-CNN. Initially, we have developed the model of Squirrel Cage induction motor in MATLAB and simulated it for single phasing and stator winding faults (SWF) using Fast Fourier Transform (FFT), Short Time Fourier Transform (STFT), and Continuous Wavelet Transform (CWT) to detect and identify the healthy and unhealthy conditions with phase to ground, single phasing and in multiple fault conditions using Motor Current Signature Analysis. The faults impact on stator current is presented in the time and frequency domain (i.e., power spectrum). The simulation results show that the scalogram has shown good results in time-frequency analysis for fault and showing its impact on the energy of current during individual fault and multiple fault conditions. This is further investigated with three deep learning models (i.e., MLP, LSTM, and 1D-CNN) for checking the fault detection and identification (i.e., classification) improvement in a three-phase induction motor. By simulating the three-phase induction motor in various healthy and unhealthy conditions in MATLAB, we have collected current signature data in the time domain, labeled them accordingly and created the 50 thousand samples dataset for DL models. All the DL models are trained and validated with a suitable number of architecture layers. By simulation, the multiclass confusion matrix, precision, recall, and F1-score are obtained in several conditions. The result shows that the stator current signature of the motor can be used to detect individual and multiple faults. Moreover, deep learning models can efficiently classify the induction motor faults based on time-domain data of the stator current signature. In deep learning (DL) models, the LSTM has shown better accuracy among all other three models. These results show that employing deep learning in fault detection and identification of induction motors can be very useful in predictive maintenance to avoid shutdown and production cycle stoppage in the industry.Keywords
Cite This Article
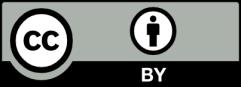