Open Access
ARTICLE
Facial Expression Recognition Based on Multi-Channel Attention Residual Network
1 School of Information Engineering, Anhui University of Chinese Medicine, Hefei, China
2 Graduate School, Angeles University Foundation, Angeles City, Philippines
* Corresponding Author: Tongping Shen. Email:
(This article belongs to the Special Issue: Models of Computation: Specification, Implementation and Challenges)
Computer Modeling in Engineering & Sciences 2023, 135(1), 539-560. https://doi.org/10.32604/cmes.2022.022312
Received 04 March 2022; Accepted 13 May 2022; Issue published 29 September 2022
Abstract
For the problems of complex model structure and too many training parameters in facial expression recognition algorithms, we proposed a residual network structure with a multi-headed channel attention (MCA) module. The migration learning algorithm is used to pre-train the convolutional layer parameters and mitigate the overfitting caused by the insufficient number of training samples. The designed MCA module is integrated into the ResNet18 backbone network. The attention mechanism highlights important information and suppresses irrelevant information by assigning different coefficients or weights, and the multi-head structure focuses more on the local features of the pictures, which improves the efficiency of facial expression recognition. Experimental results demonstrate that the model proposed in this paper achieves excellent recognition results in Fer2013, CK+ and Jaffe datasets, with accuracy rates of 72.7%, 98.8% and 93.33%, respectively.Keywords
Cite This Article
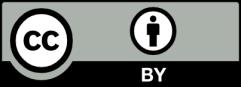