Open Access
EDITORIAL
Introduction to the Special Issue on Advances on Modeling and State Estimation for Industrial Processes
1 Key Laboratory of Advanced Process Control for Light Industry (Ministry of Education), Jiangnan University, Wuxi, 214122, China
2 University of Alberta, Edmonton, T6G 2R3, Canada
3 ABB Corporate Research Germany, Ladenburg, 68526, Germany
* Corresponding Author: Shunyi Zhao. Email:
(This article belongs to the Special Issue: Advances on Modeling and State Estimation for Industrial Processes)
Computer Modeling in Engineering & Sciences 2023, 135(1), 1-3. https://doi.org/10.32604/cmes.2022.024993
Received 16 June 2022; Accepted 21 June 2022; Issue published 29 September 2022
Abstract
This article has no abstract.In the past few years, significant progress has been made in modeling and state estimation for industrial processes to improve control performance, reliable monitoring, quick and accurate fault detection, diagnosis, high product quality, fule and resource consumption, etc. However, with the fast development of information technology, numerous essential issues are faced in modeling and state estimation, which generates the new need for novel modeling and or state estimation methodologies and in-depth studies of them. Therefore, this special issue is dedicated to innovative modeling and state estimation from applicability, computational efficiency, and effectiveness.
To disseminate the recent advances in modeling and state estimation in time, the four guest editors conceived the idea of organizing this Special Issue for our readers with interests. This Special Issue is now concluded, and we are glad to announce and share the publication of this issue with our colleagues. It contains ten peer-reviewed papers, which are authored by researchers from research institutes and universities active in the topic of modeling and state estimation worldwide. The Special Issue is dedicated to innovative theory, model, technique, and algorithm, as well as modeling and state estimation applications. Specifically, topics across Variational Bayesian Modeling Methods for Industrial Process, Transfer Modeling for Industrial Process, Unsupervised Modeling for Industrial Process, First Principle Modeling for Industrial Process, Non-parametric Bayesian Modeling for Industrial Process, Distributed Multi-Agent Modeling Algorithms and Its Industrial Applications, Robust Modeling Methods for Industrial Process, Supervised Modeling and Its Industrial Applications, Filter-Aided Methods for Industrial Processes, Nonlinear Modeling Methods, and Its Industrial Applications are included.
Specifically, “Enhancing the Effectiveness of Trimethylchlorosilane Purification Process Monitoring with Variational Autoencoder” by Wang et al. [1], addresses a critically important question regarding the process monitoring of Trimethylchlorosilane Purification Process and proposes a new algorithm based on Variational AutoEncoders (VAE) for the high-dimensional industrial database; “Improved High Order Model-Free Adaptive Iterative Learning Control with Disturbance Compensation and Enhanced Convergence” by Wang et al. [2], proposes to employ the compact format dynamic linearization method to improve high-order model-free adaptive iterative control strategy; “State Estimation Moving Window Gradient Iterative Algorithm for Bilinear Systems Using the Continuous Mixed p-norm Technique” by Liu et al. [3], develops a novel gradient iterative algorithm by means of the continuous mixed p-norm cost function with the purpose of estimating the parameters of the bilinear systems; “Skew t Distribution-Based Nonlinear Filter with Asymmetric Measurement Noise Using Variational Bayesian Inference” by Xu et al. [4], addresses the state estimation problems for nonlinear systems with unknown statistics of measurement noise by means of the cubature Kalman filter and the skew t distribution; “A Novel Bidirectional Interaction Model and Electric Energy Measuring Scheme of EVs for V2G with Distorted Power Loads” by Cui et al. [5], proposes a novel bidirectional interaction model based on modulation theory with nonlinear loads and a novel electric energy measuring scheme of EVs for V2G; “Pattern-Moving-Based Parameter Identification of Output Error Models with Multi-Threshold Quantized Observations” by Li et al. [6], addresses a modified auxiliary model stochastic gradient recursive parameter identification algorithm (M-AM-SGRPIA) for a class of single input single output (SISO) linear output error models with multi-threshold quantized observations; “Range-Only UWB SLAM for Indoor Robot Localization Employing Multi-Interval EFIR Rauch-Tung-Striebel Smoother” by Gao et al. [7], proposes a multi-interval extended finite impulse response (EFIR)-based Rauch-Tung-Striebel (R-T-S) smoother for the range-only ultra-wideband (UWB) simultaneous localization and mapping (SLAM) for robot localization; “Improved adaptive iterated extended Kalman filter for GNSS/INS/UWB-integrated fixed-point positioning” by Wu et al. [8], proposes an adaptive iterated extended Kalman filter for fixed point positioning, which integrates global navigation satellite system, inertial navigation system, ultra wide band; “Change Point Detection for Process Data Analytics Applied to a Multiphase Flow Facility” by Gedda et al. [9], utilizes regularisation-based cost functions to handle ill-posed problems of noisy data with aim of improving the performance of process data analytics; “Self-triggered Consensus Filtering over Asynchronous Communication Sensor Networks” by Xue et al. [10], develops a self-triggered consensus filtering by designing the self-triggered policy and asynchronous distributed filter simultaneously.
As a final remark, it is hoped that the presented topics will give this special issue a much more lasting value and make it appealing to a broad audience of researchers, practitioners, and students interested in modeling and state estimation.
Acknowledgement: We would like to thank the authors for their contributions to this Special Issue. We also thank the journal of CMES for the supports for publications of this Special Issue.
Funding Statement: This work is supported by the 111 Project (B12018).
Conflicts of Interest: The authors declare that they have no conflicts of interest to report regarding the present study.
References
1. Wang, J., Zhao, S., Liu, F., Ma, Z. (2022). Enhancing the effectiveness of trimethylchlorosilane purification process monitoring with variational autoencoder. Computer Modeling in Engineering & Sciences, 132(2), 531–552. DOI 10.32604/cmes.2022.019521. [Google Scholar] [CrossRef]
2. Wang, Z., Gao, F., Liu, F. (2022). Enhancing the effectiveness of trimethylchlorosilane purification process monitoring with variational autoencoder. Computer Modeling in Engineering & Sciences, 134(1), 343–355. DOI 10.32604/cmes.2022.020569. [Google Scholar] [CrossRef]
3. Liu, W., Ma, J., Xiong, W. (2022). State estimation moving window gradient iterative algorithm for bilinear systems using the continuous mixed p-norm technique. Computer Modeling in Engineering & Sciences, 134(2), 873–892. DOI 10.32604/cmes.2022.020565. [Google Scholar] [CrossRef]
4. Xu, C., Mao, Y., Chen, H., Tao, H., Liu, F. (2022). Skew t distribution-based nonlinear filter with asymmetric measurement noise using variational Bayesian inference. Computer Modeling in Engineering & Sciences, 131(1), 349–364. DOI 10.32604/cmes.2021.019027. [Google Scholar] [CrossRef]
5. Cui, J., Li, Q., Cao, B., Li, X., Yan, Q. (2022). A novel bidirectional interaction model and electric energy measuring scheme of EVs for V2G with distorted power loads. Computer Modeling in Engineering & Sciences, 130(3), 1789–1806. DOI 10.32604/cmes.2022.017958. [Google Scholar] [CrossRef]
6. Li, X., Xu, Z., Han, C., Li, N. (2022). Pattern-moving-based parameter identification of output error models with multi-threshold quantized observations. Computer Modeling in Engineering & Sciences, 130(3), 1807–1825. DOI 10.32604/cmes.2022.017799. [Google Scholar] [CrossRef]
7. Gao, Y., Ma, W., Cao, J., Qu, J., Xu, Y. (2022). Range-only UWB SLAM for indoor robot localization employing multi-interval EFIR rauch-tung-striebel smoother. Computer Modeling in Engineering & Sciences, 130(2), 1221–1237. DOI 10.32604/cmes.2022.017533. [Google Scholar] [CrossRef]
8. Wu, Q., Li, C., Shen, T., Xu, Y. (2023). Improved adaptive iterated extended kalman filter for GNSS/INS/UWB-integrated fixed-point positioning. Computer Modeling in Engineering & Sciences, 134(3), 1761–1772. DOI 10.32604/cmes.2022.020545. [Google Scholar] [CrossRef]
9. Gedda, R., Beilina, L., Tan, R. (2023). Change point detection for process data analytics applied to a multiphase flow facility. Computer Modeling in Engineering & Sciences, 134(3), 1737–1759. DOI 10.32604/cmes.2022.019764. [Google Scholar] [CrossRef]
10. Xue, H., Wen, J., Swain, A., Luan, X. (2022). Self-triggered consensus filtering over asynchronous communication sensor networks. Computer Modeling in Engineering & Sciences, 134(2), 857–871. DOI 10.32604/cmes.2022.020127. [Google Scholar] [CrossRef]
Cite This Article
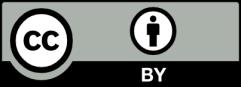