Open Access
ARTICLE
Brain Functional Networks with Dynamic Hypergraph Manifold Regularization for Classification of End-Stage Renal Disease Associated with Mild Cognitive Impairment
1 School of Microelectronics and Control Engineering, Changzhou University, Changzhou, 213164, China
2 School of Computer Science and Artificial Intelligence, Changzhou University, Changzhou, 213164, China
3 Department of Radiology, Changzhou Second People’s Hospital Affiliated to Nanjing Medical University, Changzhou, 213003, China
* Corresponding Author: Zhuqing Jiao. Email:
(This article belongs to the Special Issue: Computer Modeling of Artificial Intelligence and Medical Imaging)
Computer Modeling in Engineering & Sciences 2023, 135(3), 2243-2266. https://doi.org/10.32604/cmes.2023.023544
Received 30 April 2022; Accepted 08 August 2022; Issue published 23 November 2022
Abstract
The structure and function of brain networks have been altered in patients with end-stage renal disease (ESRD). Manifold regularization (MR) only considers the pairing relationship between two brain regions and cannot represent functional interactions or higher-order relationships between multiple brain regions. To solve this issue, we developed a method to construct a dynamic brain functional network (DBFN) based on dynamic hypergraph MR (DHMR) and applied it to the classification of ESRD associated with mild cognitive impairment (ESRDaMCI). The construction of DBFN with Pearson’s correlation (PC) was transformed into an optimization model. Node convolution and hyperedge convolution superposition were adopted to dynamically modify the hypergraph structure, and then got the dynamic hypergraph to form the manifold regular terms of the dynamic hypergraph. The DHMR and L1 norm regularization were introduced into the PC-based optimization model to obtain the final DHMR-based DBFN (DDBFN). Experiment results demonstrated the validity of the DDBFN method by comparing the classification results with several related brain functional network construction methods. Our work not only improves better classification performance but also reveals the discriminative regions of ESRDaMCI, providing a reference for clinical research and auxiliary diagnosis of concomitant cognitive impairments.Graphical Abstract
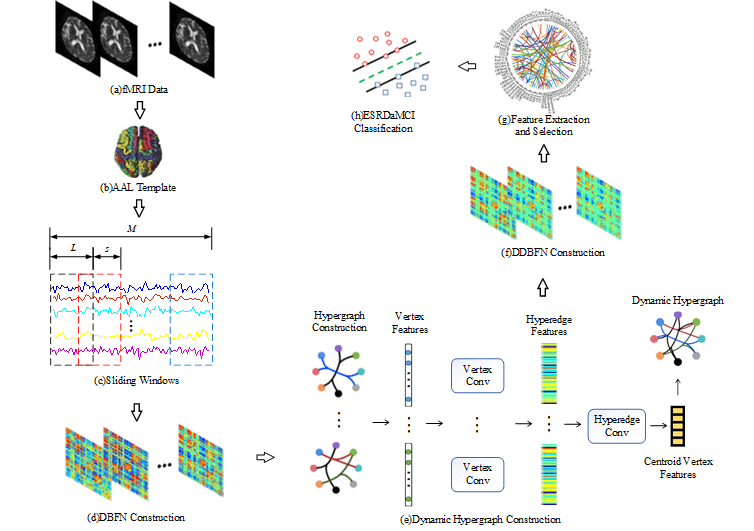
Keywords
Cite This Article
Xi, Z., Song, C., Zheng, J., Shi, H., Jiao, Z. (2023). Brain Functional Networks with Dynamic Hypergraph Manifold Regularization for Classification of End-Stage Renal Disease Associated with Mild Cognitive Impairment. CMES-Computer Modeling in Engineering & Sciences, 135(3), 2243–2266. https://doi.org/10.32604/cmes.2023.023544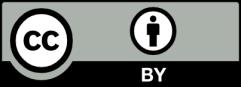